新建
Transformer
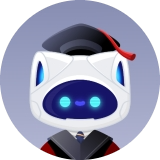
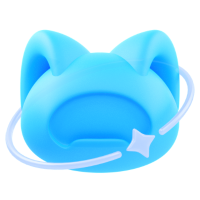
更新于 2024-12-23
推荐镜像 :DeePMD-kit:3.0.0-cuda12.1
推荐机型 :c2_m4_cpu
赞
双击即可修改
代码
文本
[2]
!pip install torch
!pip install pandas
!pip install numpy
!pip install matplotlib
!pip install scikit-learn
!pip install tensorflow
!pip install scikeras
Looking in indexes: https://pypi.tuna.tsinghua.edu.cn/simple Requirement already satisfied: torch in /opt/deepmd-kit-3.0.0/lib/python3.12/site-packages (2.4.1.post302) Requirement already satisfied: filelock in /opt/deepmd-kit-3.0.0/lib/python3.12/site-packages (from torch) (3.16.1) Requirement already satisfied: typing-extensions>=4.8.0 in /opt/deepmd-kit-3.0.0/lib/python3.12/site-packages (from torch) (4.12.2) Requirement already satisfied: sympy in /opt/deepmd-kit-3.0.0/lib/python3.12/site-packages (from torch) (1.13.3) Requirement already satisfied: networkx in /opt/deepmd-kit-3.0.0/lib/python3.12/site-packages (from torch) (3.4.2) Requirement already satisfied: jinja2 in /opt/deepmd-kit-3.0.0/lib/python3.12/site-packages (from torch) (3.1.4) Requirement already satisfied: fsspec in /opt/deepmd-kit-3.0.0/lib/python3.12/site-packages (from torch) (2024.10.0) Requirement already satisfied: setuptools in /opt/deepmd-kit-3.0.0/lib/python3.12/site-packages (from torch) (75.6.0) Requirement already satisfied: MarkupSafe>=2.0 in /opt/deepmd-kit-3.0.0/lib/python3.12/site-packages (from jinja2->torch) (3.0.2) Requirement already satisfied: mpmath<1.4,>=1.1.0 in /opt/deepmd-kit-3.0.0/lib/python3.12/site-packages (from sympy->torch) (1.3.0) WARNING: Running pip as the 'root' user can result in broken permissions and conflicting behaviour with the system package manager, possibly rendering your system unusable.It is recommended to use a virtual environment instead: https://pip.pypa.io/warnings/venv. Use the --root-user-action option if you know what you are doing and want to suppress this warning.
--------------------------------------------------------------------------- KeyboardInterrupt Traceback (most recent call last) File /opt/deepmd-kit-3.0.0/lib/python3.12/site-packages/IPython/utils/_process_posix.py:151, in ProcessHandler.system(self, cmd) 150 else: --> 151 child = pexpect.spawn(self.sh, args=['-c', cmd]) # Vanilla Pexpect 152 flush = sys.stdout.flush File /opt/deepmd-kit-3.0.0/lib/python3.12/site-packages/pexpect/pty_spawn.py:205, in spawn.__init__(self, command, args, timeout, maxread, searchwindowsize, logfile, cwd, env, ignore_sighup, echo, preexec_fn, encoding, codec_errors, dimensions, use_poll) 204 else: --> 205 self._spawn(command, args, preexec_fn, dimensions) 206 self.use_poll = use_poll File /opt/deepmd-kit-3.0.0/lib/python3.12/site-packages/pexpect/pty_spawn.py:303, in spawn._spawn(self, command, args, preexec_fn, dimensions) 300 self.args = [a if isinstance(a, bytes) else a.encode(self.encoding) 301 for a in self.args] --> 303 self.ptyproc = self._spawnpty(self.args, env=self.env, 304 cwd=self.cwd, **kwargs) 306 self.pid = self.ptyproc.pid File /opt/deepmd-kit-3.0.0/lib/python3.12/site-packages/pexpect/pty_spawn.py:315, in spawn._spawnpty(self, args, **kwargs) 314 '''Spawn a pty and return an instance of PtyProcess.''' --> 315 return ptyprocess.PtyProcess.spawn(args, **kwargs) File /opt/deepmd-kit-3.0.0/lib/python3.12/site-packages/ptyprocess/ptyprocess.py:315, in PtyProcess.spawn(cls, argv, cwd, env, echo, preexec_fn, dimensions, pass_fds) 314 os.close(exec_err_pipe_write) --> 315 exec_err_data = os.read(exec_err_pipe_read, 4096) 316 os.close(exec_err_pipe_read) KeyboardInterrupt: During handling of the above exception, another exception occurred: UnboundLocalError Traceback (most recent call last) Cell In[2], line 2 1 get_ipython().system('pip install torch') ----> 2 get_ipython().system('pip install pandas') 3 get_ipython().system('pip install numpy') 4 get_ipython().system('pip install matplotlib') File /opt/deepmd-kit-3.0.0/lib/python3.12/site-packages/ipykernel/zmqshell.py:657, in ZMQInteractiveShell.system_piped(self, cmd) 655 self.user_ns["_exit_code"] = system(cmd) 656 else: --> 657 self.user_ns["_exit_code"] = system(self.var_expand(cmd, depth=1)) File /opt/deepmd-kit-3.0.0/lib/python3.12/site-packages/IPython/utils/_process_posix.py:167, in ProcessHandler.system(self, cmd) 162 out_size = len(child.before) 163 except KeyboardInterrupt: 164 # We need to send ^C to the process. The ascii code for '^C' is 3 165 # (the character is known as ETX for 'End of Text', see 166 # curses.ascii.ETX). --> 167 child.sendline(chr(3)) 168 # Read and print any more output the program might produce on its 169 # way out. 170 try: UnboundLocalError: cannot access local variable 'child' where it is not associated with a value
代码
文本
[2]
import torch
import torch.nn as nn
import torch.optim as optim
from torch.nn.utils.rnn import pad_sequence
import torch.nn.functional as F
import math
import random
import numpy as np
import pandas as pd
import matplotlib.pyplot as plt
from sklearn.preprocessing import MinMaxScaler
代码
文本
[3]
device = 'cuda' if torch.cuda.is_available() else 'cpu' # 如果电脑有 GPU ,则在 GPU 上运算,否则在 CPU 上运算
代码
文本
[4]
#如果是非0元素且不是最后一个元素且与下一元素不同的添加到结果列表 result 中
def split_list_by_value(lst):
result = []
temp = []
for i in range(len(lst)):
if lst[i] != 0:
temp.append(lst[i])
if i == len(lst)-1 or lst[i] != lst[i+1]:
result.append(temp)
temp = []
return result
#将长度为 length 的子列表添加到结果列表 result 中
def split_list_by_lengths(lst, lengths):
result = []
start = 0
for length in lengths:
result.append(lst[start:start+length])
start += length
return result
代码
文本
[5]
total_num = 222#数据总数
train_size = 194
test_size = 28
CC_input = []
cyc_len = []
baty_lst = [2,3,4,6,7,8]
for i in baty_lst:
CC_data = pd.read_csv(f"/personal/Capacity data/Data_Capacity_25C0{i}.csv")#用于导入不同电池批次的数据
cycle_num = CC_data.iloc[:, 1:2]["cycle number"].tolist()# .iloc 是用来基于行和列的位置进行索引选择的方法,导入第二行循环次数 cycle number 的数据,并把 Dataframe 数据转换为列表
vol_all = CC_data.iloc[:, 3:4]['Ewe/V'].tolist()# .iloc 是用来基于行和列的位置进行索引选择的方法,导入第四行电压 Ewe/V 的数据, Dataframe 数据转换为列表
cyc = split_list_by_value(cycle_num)#分割循环次数,每个电池的循环都变成 cycle_num 列表中的一个子列表
if i == 7:
cyc = cyc[:-7]#由于部分EIS的数据点异常,去除电池批号为7的最后7个元素
cyc_len.append(len(cyc))
lengths = [len(sublist) for sublist in cyc]#计算 cyc 中每个子列表的长度
vol_list = split_list_by_lengths(vol_all, lengths)#按照 cyc 中每个子列表的长度去分割电压数据
tensor_vol = [torch.tensor(sublist) for sublist in vol_list]
padded_vol = pad_sequence(tensor_vol, batch_first=True, padding_value=0)#将 tensor_vol 中的张量填充到相同的长度, batch_first=True 表示批次大小为第一个维度, padding_value=0 表示用0填充
#print(padded_vol.shape)
new_vol = [t[:1654].view(1654,1) for t in padded_vol]#将 padded_vol 中的每个张量截取前1654个元素,并调整形状为(1, 1654, 1)
CC_input += new_vol#将 new_vol 列表中的元素添加到 CC_input 列表中
print(len(CC_input), cyc_len)
222 [40, 39, 34, 40, 33, 36]
代码
文本
[6]
indices = list(range(len(CC_input)))
random.shuffle(indices)# shuffle 是 random 模块中的一个函数,用于将传入的 indices 就地打乱顺序
代码
文本
[7]
scaler = MinMaxScaler(feature_range=(0, 1))
# CC_input_scaled = []
# for data in CC_input:
# data = np.array(data).squeeze() # 转换为数组并去除多余的维度
# scaled_data = scaler.fit_transform(data.reshape(-1, 1))# 将数据重新形状为(-1, 1)使其适应 MinMaxScaler 的输入格式
# CC_input_scaled.append(torch.tensor(scaled_data).view(1, -1, 1))# 将标准化后的数据转换为 Tensor 并添加到列表中
代码
文本
[8]
train_indices = indices[:train_size]#打乱训练集索引
test_indices = indices[train_size:]#打乱测试集索引
encoder_input_train=[CC_input[i] for i in train_indices]
encoder_input_test=[CC_input[i] for i in test_indices]
代码
文本
[9]
decoder_input = []
decoder_target = []
cyc_tot = 0
#创建一个三维列表,用于存储电化学阻抗谱( EIS )数据.其 shape 为(222, 240, 2).第一维包含222个元素,代表四个电池的总循环次数,每个元素是一个包含两个子列表(实部和虚部)的列表,每个子列表有240个元素(每个电池每个循环的 EIS 有60个点,每个电池每个循环有四个 state ,一共240个)
EIS_list = [[[0 for i in range(240)] for j in range(2)] for r in range(222)]
lst = [1, 4, 5, 9]
for k in baty_lst:
EIS_tot = []
for i in lst:
EIS = pd.read_csv(f"/personal/EIS data/EIS_state_{i}_25C0{k}.csv")#导入不同批次电池不同电池soc状态的EIS数据
cyc_n = cyc_len[baty_lst.index(k)]
#print(cyc_n)
EIS_tot.append(EIS.iloc[:, 3:7][" Re(Z)/Ohm"].tolist()[0:60 * cyc_n])#选择第四列 Re(Z)/Ohm 实部数据
EIS_tot.append(EIS.iloc[:, 4:7][" -Im(Z)/Ohm"].tolist()[0:60 * cyc_n])#选择第五列 -Im(Z)/Ohm 虚部数据
cyc_tot += cyc_n
EIS_totm = [[e2 for e2 in e1] for e1 in EIS_tot]#扁平化嵌套列表
print(np.array(EIS_totm).shape, cyc_tot)
lengths = [60 for i in range(cyc_n)]
# print(lengths)
for i in range(4):
EIS_R = split_list_by_lengths(EIS_totm[2 * i], lengths)#将 EIS 数据的实部按照每个电池的循环次数分割成多个子列表
EIS_I = split_list_by_lengths(EIS_totm[2 * i + 1], lengths)#将 EIS 数据的虚部按照每个电池的循环次数分割成多个子列表
for j in range(cyc_tot - cyc_n, cyc_tot, 1):#这个循环遍历当前电池的 EIS 数据
EIS_list[j][0][60 * i:60 * (i + 1)] = EIS_R[j - cyc_tot + cyc_n]#将实部数据 EIS_R 中的第 j - cyc_tot + cyc_n 个元素(即当前电池的实部数据)赋值给 EIS_list 的相应位置. 60 * i:60 * (i + 1) 确定了在 EIS_list 中的起始和结束索引
EIS_list[j][1][60 * i:60 * (i + 1)] = EIS_I[j - cyc_tot + cyc_n]
EIS_list = [np.array(t).squeeze().T for t in EIS_list]#每个电池的EIS数据都被整理成一个二维数组,其中行代表不同的 SOC 状态,列代表不同的数据点
print(np.array(EIS_list)[0].shape)
(8, 2400) 40 (8, 2340) 79 (8, 2040) 113 (8, 2400) 153 (8, 1980) 186 (8, 2160) 222 (240, 2)
代码
文本
[10]
EIS_scaled = []
for i in range(len(EIS_list)):
EIS_data = EIS_list[i] # 获取 EIS 数据
# 使用 MinMaxScaler 对每个 EIS 数据进行标准化
scaled_EIS = scaler.fit_transform(EIS_data) # 将数据标准化到[0, 1]
EIS_scaled.append(torch.tensor(scaled_EIS).float()) # 转换为 Tensor 并添加到列表中
print("Standardized EIS data:", np.array(EIS_scaled).shape)
Standardized EIS data: (222, 240, 2)
代码
文本
[11]
decoder_target = [torch.tensor(t).float().view(240,2) for t in EIS_scaled] # 去掉最后一个维度
decoder_input = [torch.ones(240,1) for t in EIS_scaled] # 去掉最后一个维度
decoder_input_train = [decoder_input[i] for i in train_indices]
decoder_target_train = [decoder_target[i] for i in train_indices]
decoder_input_test = [decoder_input[i] for i in test_indices]
decoder_target_test = [decoder_target[i] for i in test_indices]
print(encoder_input_train[0].shape)
print(decoder_input_train[0].shape)
torch.Size([1654, 1]) torch.Size([240, 1]) /tmp/ipykernel_234/2944544641.py:1: UserWarning: To copy construct from a tensor, it is recommended to use sourceTensor.clone().detach() or sourceTensor.clone().detach().requires_grad_(True), rather than torch.tensor(sourceTensor). decoder_target = [torch.tensor(t).float().view(240,2) for t in EIS_scaled] # 去掉最后一个维度
代码
文本
[12]
class TransformerModel(nn.Module):
def __init__(self, feature_dim, hidden_dim, num_heads, num_layers, output_dim):
super(TransformerModel, self).__init__()
# Encoder 和 Decoder 的层
self.encoder = nn.TransformerEncoder(
nn.TransformerEncoderLayer(d_model=hidden_dim, nhead=num_heads),
num_layers=num_layers
)
self.decoder = nn.TransformerDecoder(
nn.TransformerDecoderLayer(d_model=hidden_dim, nhead=num_heads),
num_layers=num_layers
)
# 输出层将隐藏状态映射到目标空间
self.fc_out = nn.Linear(hidden_dim, output_dim)
self.embedding = nn.Linear(feature_dim, hidden_dim) # 线性变换,用于特征嵌入
def forward(self, encoder_input, decoder_input, use_teacher_forcing=True):
"""
encoder_input: [batch_size, seq_len, feature_dim]
decoder_input: [batch_size, seq_len, feature_dim]
"""
batch_size, seq_len, _ = encoder_input.size()
# 将输入通过embedding层映射到更高的维度
encoder_input = self.embedding(encoder_input) # [batch_size, seq_len, hidden_dim]
decoder_input = self.embedding(decoder_input) # [batch_size, seq_len, hidden_dim]
# Encoder
encoder_input = encoder_input.permute(1, 0, 2) # 转换为 [seq_len, batch_size, hidden_dim]
memory = self.encoder(encoder_input) # [seq_len, batch_size, hidden_dim]
# Decoder
decoder_input = decoder_input.permute(1, 0, 2) # 转换为 [seq_len, batch_size, hidden_dim]
output = self.decoder(decoder_input, memory) # [seq_len, batch_size, hidden_dim]
# 输出层
output = output.permute(1, 0, 2) # 转换回 [batch_size, seq_len, hidden_dim]
output = self.fc_out(output) # [batch_size, seq_len, output_dim]
return output
# 设置模型参数
feature_dim = 1 # 输入数据的特征维度,电压数据
hidden_dim = 256 # 隐藏层维度
num_heads = 1 # 多头注意力的头数
num_layers = 4 # Encoder 和 Decoder 的层数
output_dim = 2 # 输出维度(阻抗数据:实部和虚部)
# 实例化模型并转移到设备
model = TransformerModel(feature_dim, hidden_dim, num_heads, num_layers, output_dim).to(device)
/opt/deepmd-kit-3.0.0/lib/python3.12/site-packages/torch/nn/modules/transformer.py:307: UserWarning: enable_nested_tensor is True, but self.use_nested_tensor is False because encoder_layer.self_attn.batch_first was not True(use batch_first for better inference performance) warnings.warn(f"enable_nested_tensor is True, but self.use_nested_tensor is False because {why_not_sparsity_fast_path}")
代码
文本
[15]
criterion = nn.MSELoss().to(device)
optimizer = optim.Adam(model.parameters(), lr=0.001)
# 调整输入维度为 (seq_len, batch_size, feature_dim)
# 假设 encoder_input_train 和 decoder_input_train 是 [batch_size, seq_len, feature_dim] 格式的输入
# 训练过程
num_epochs = 1500
batch_size = 4
train_losses = [] # 记录每个epoch的训练损失
for epoch in range(num_epochs):
epoch_loss = 0.0
teacher_forcing_ratio = max(0.5 * (1 - epoch / num_epochs), 0.0)
use_teacher_forcing = True # 使用教师强迫
for batch_idx in range(0, len(encoder_input_train), batch_size):
batch_encoder_input = torch.stack(encoder_input_train[batch_idx:batch_idx + batch_size], dim=0).to(device)
batch_decoder_input = torch.stack(decoder_input_train[batch_idx:batch_idx + batch_size], dim=0).to(device)
# 打印每个 batch 的形状
#print(f"Batch encoder_input shape: {batch_encoder_input.shape}") # 应该是 [batch_size, seq_len, feature_dim]
#print(f"Batch decoder_input shape: {batch_decoder_input.shape}") # 应该是 [batch_size, seq_len, feature_dim]
# 检查目标的形状
batch_decoder_target = torch.stack(decoder_target_train[batch_idx:batch_idx + batch_size], dim=0).to(device)
#print(f"Batch decoder_target shape: {batch_decoder_target.shape}") # 应该是 [batch_size, seq_len, feature_dim]
optimizer.zero_grad()
outputs = model(batch_encoder_input, batch_decoder_input, use_teacher_forcing)
loss = criterion(outputs, batch_decoder_target)
loss.backward()
optimizer.step()
optimizer.zero_grad()
outputs = model(batch_encoder_input, batch_decoder_input, use_teacher_forcing).to(device)
loss = criterion(outputs, batch_decoder_target)
loss.backward()
optimizer.step()
epoch_loss += loss.item()
train_losses.append(epoch_loss / (train_size / batch_size))
if (epoch + 1) % 20 == 0:
mean_mse = epoch_loss / (train_size / batch_size)
rmse = mean_mse ** 0.5
print(f"Epoch [{epoch + 1}/{num_epochs}], Loss: {mean_mse:.8f}, RMSE: {rmse}")
# 绘制训练损失图
plt.plot(train_losses, label='Training loss')
plt.xlabel('Epochs')
plt.ylabel('MSE Loss')
plt.legend()
plt.show()
Epoch [20/1500], Loss: 0.00167714, RMSE: 0.04095286200796598 Epoch [40/1500], Loss: 0.00162265, RMSE: 0.04028215439732387 Epoch [60/1500], Loss: 0.00153249, RMSE: 0.03914706917199841 Epoch [80/1500], Loss: 0.00151570, RMSE: 0.03893194305537772 Epoch [100/1500], Loss: 0.00145348, RMSE: 0.03812456504015348 Epoch [120/1500], Loss: 0.00145639, RMSE: 0.03816265232283357 Epoch [140/1500], Loss: 0.00145280, RMSE: 0.03811561865863891 Epoch [160/1500], Loss: 0.00146424, RMSE: 0.03826544355273072 Epoch [180/1500], Loss: 0.00145403, RMSE: 0.038131706533742385 Epoch [200/1500], Loss: 0.00144737, RMSE: 0.03804426260136165 Epoch [720/1500], Loss: 0.00144733, RMSE: 0.03804385476784955 Epoch [740/1500], Loss: 0.00145215, RMSE: 0.03810709144747651 Epoch [760/1500], Loss: 0.00144412, RMSE: 0.03800152735297806
代码
文本
[ ]
model.eval().to (device)
test_losses = []
for batch_idx in range(0, test_size, batch_size):
batch_encoder_input = torch.cat(encoder_input_test[batch_idx:batch_idx+batch_size], dim=0).to (device)
batch_decoder_input = torch.cat(decoder_input_test[batch_idx:batch_idx+batch_size], dim=0).to (device)
batch_decoder_target = torch.cat(decoder_target_test[batch_idx:batch_idx+batch_size], dim=0).to (device)
with torch.no_grad():
outputs = model(batch_encoder_input, batch_decoder_input, use_teacher_forcing).to (device)
loss = criterion(outputs, batch_decoder_target).to (device)
test_losses.append(loss.item())
mean_mse = np.mean(test_losses)
test_rmse = mean_mse ** 0.5
print(f"Test Loss: {mean_mse:.4f} Test RMSE: {test_rmse:.4f}")
代码
文本
[ ]
predict_outputs = outputs[0].view(1, 240, 2).tolist()
predict_data = np.array(predict_outputs).squeeze().T
decoder_target = batch_decoder_target[0].view(1, 240, 2).tolist()
target_data = np.array(decoder_target).squeeze().T
代码
文本
[ ]
for i in range(4):
if i == 1:
label_p = 'Predict EIS'
label_t = 'Target EIS'
else:
label_p = None
label_t = None
plt.plot(predict_data[0][60 * i:60 * (i + 1)], predict_data[1][60 * i:60 * (i + 1)], 'ro-', label=label_p)
plt.plot(target_data[0][60 * i:60 * (i + 1)], target_data[1][60 * i:60 * (i + 1)], 'bo-', label=label_t)
plt.xlabel('Z_re')
plt.ylabel('Z_im')
plt.legend()
plt.show()
代码
文本
[ ]
plt.figure(figsize=(11, 25))
title_name = [1, 4, 5, 9]
for i in range(4):
label_p = 'Predict EIS'
label_t = 'Target EIS'
plt.subplot(5, 2, i + 1)
plt.plot(predict_data[0][60 * i:60 * (i + 1)], predict_data[1][60 * i:60 * (i + 1)], 'ro-', label=label_p)
plt.plot(target_data[0][60 * i:60 * (i + 1)], target_data[1][60 * i:60 * (i + 1)], 'bo-', label=label_t)
titname = title_name[i]
plt.title(f"state {titname}")
plt.xlabel('Z_re')
plt.ylabel('Z_im')
plt.legend()
plt.show()
代码
文本
[ ]
def r2_score(y_true, y_pred):
ss_total = np.sum((y_true - np.mean(y_true)) ** 2)
ss_res = np.sum((y_true - y_pred) ** 2)
r2 = 1 - (ss_res / ss_total)
return r2
r2_r = 0
r2_i = 0
for i in range(4):
r2_r += r2_score(target_data[0][60 * i:60 * (i + 1)], predict_data[0][60 * i:60 * (i + 1)]) / 4
r2_i += r2_score(target_data[1][60 * i:60 * (i + 1)], predict_data[1][60 * i:60 * (i + 1)]) / 4
print(f"R2 real score:{r2_r.item():.4f}", f"R2 imaginary score:{r2_i.item():.4f}")
for i in range(4):
if i == 0:
plt.plot(target_data[0][60 * i:60 * (i + 1)], target_data[0][60 * i:60 * (i + 1)], 'c-', linewidth=2,
label='ground truth')
label_p = f'R2_real score:{r2_r:.4f}'
else:
label_p = None
plt.plot(target_data[0][60 * i:60 * (i + 1)], predict_data[0][60 * i:60 * (i + 1)], 'mo', markerfacecolor='none',
label=label_p)
plt.xlabel('real_true')
plt.ylabel('real_pred')
plt.title('Z real R2')
plt.legend()
plt.show()
for i in range(4):
if i == 0:
plt.plot(target_data[1][60 * i:60 * (i + 1)], target_data[1][60 * i:60 * (i + 1)], 'c-', linewidth=2,
label='ground truth')
label_p = f'R2_image score:{r2_i:.4f}'
else:
label_p = None
plt.plot(target_data[1][60 * i:60 * (i + 1)], predict_data[1][60 * i:60 * (i + 1)], 'mo', markerfacecolor='none',
label=label_p)
plt.xlabel('real_true')
plt.ylabel('real_pred')
plt.title('Z image R2')
plt.legend()
plt.show()
代码
文本
点个赞吧