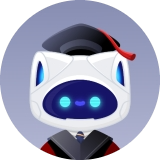
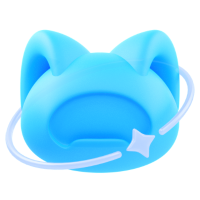
Spatial domains
TL;DR we provide an overview of spatial data analysis methods for the analysis of spatial omics data
Motivation
When analyzing spatial omics dataset, we might be interested in identifying spatial patterns in the data, that is, identifying features that vary in space. Spatial omics data entails not only the usual cell x gene matrix but, additionally orthogonal information that we can use to describe and predict features of interest from the data, such as the tissue image and the spatial coordinates.
The identification of cell types or states is one of the first data analysis tasks, as it allows to formulate key hypotheses from the data. This is usually performed by clustering the data based on some type of similarity between data points in feature space. One of the most popular approaches for this task is to compute a nearest neighbor graph on a (low dimensional) representation of the data and then perform community detection on such a graph. In the case of spatial omics data, such an approach can be easily extended to account for the similarity in coordinate space (and not only feature space) of the data. We can call this task "identification of spatial domains" since it includes both gene and spatial similarity for cluster identification.
Different models have been developed for identifying spatial domains with varying underlying concepts. They can generally be divided into two groups of methods. The first models spatial dependencies of gene expression and the second additionally incorporates information extracted from histological images.
:::{figure-md} domains
Spatial domains are clusters that both account for similarities in gene expression as well as spatial proximity. Methods can additionally incorporate the histological image information. :::
Examples for the first group are:
- Spatial domains in Squidpy {cite}
Palla2022
- Hidden-Markov random field (HMRF) {cite}
Dries2021
- BayesSpace {cite}
Zhao2021-vi
Examples for the second group are:
- spaGCN {cite}
Hu2021-SpaGCN
- stLearn {cite}
pham_stlearn_2020
In this notebook, we will show how to calculate spatial domains in Squidpy and how to apply spaGCN.
Environment setup and data
We first load the packages needed in this tutorial and the dataset.
Looking in indexes: https://pypi.tuna.tsinghua.edu.cn/simple Collecting scanpy Downloading https://pypi.tuna.tsinghua.edu.cn/packages/3b/72/436046ca332b933ca7d09cd45b86154232203e068e8307a102d5349e9444/scanpy-1.9.8-py3-none-any.whl (2.0 MB) ━━━━━━━━━━━━━━━━━━━━━━━━━━━━━━━━━━━━━━━━ 2.0/2.0 MB 20.0 MB/s eta 0:00:00a 0:00:01 Collecting umap-learn>=0.3.10 Downloading https://pypi.tuna.tsinghua.edu.cn/packages/d1/1b/46802a050b1c55d10c4f59fc6afd2b45ac9b4f62b2e12092d3f599286f14/umap_learn-0.5.6-py3-none-any.whl (85 kB) ━━━━━━━━━━━━━━━━━━━━━━━━━━━━━━━━━━━━━━━━ 85.7/85.7 kB 31.3 MB/s eta 0:00:00 Requirement already satisfied: h5py>=3 in /opt/conda/lib/python3.8/site-packages (from scanpy) (3.1.0) Requirement already satisfied: packaging in /opt/conda/lib/python3.8/site-packages (from scanpy) (23.0) Requirement already satisfied: numpy>=1.17.0 in /opt/conda/lib/python3.8/site-packages (from scanpy) (1.22.4) Requirement already satisfied: scipy>=1.4 in /opt/conda/lib/python3.8/site-packages (from scanpy) (1.7.3) Requirement already satisfied: patsy in /opt/conda/lib/python3.8/site-packages (from scanpy) (0.5.3) Requirement already satisfied: numba>=0.41.0 in /opt/conda/lib/python3.8/site-packages (from scanpy) (0.56.4) Collecting get-annotations Downloading https://pypi.tuna.tsinghua.edu.cn/packages/c6/42/9515026b884c7d5877c610082d35bcbad4f8ff4dfbf31aff0fe914f7ff3d/get_annotations-0.1.2-py3-none-any.whl (4.5 kB) Requirement already satisfied: natsort in /opt/conda/lib/python3.8/site-packages (from scanpy) (5.5.0) Collecting anndata>=0.7.4 Downloading https://pypi.tuna.tsinghua.edu.cn/packages/a7/ee/767a05c299d95b438ef9c8ab6dbc15896cfb9121cf4327fe1da160a45343/anndata-0.9.2-py3-none-any.whl (104 kB) ━━━━━━━━━━━━━━━━━━━━━━━━━━━━━━━━━━━━━━ 104.2/104.2 kB 23.0 MB/s eta 0:00:00 Requirement already satisfied: networkx>=2.3 in /opt/conda/lib/python3.8/site-packages (from scanpy) (3.0) Requirement already satisfied: matplotlib>=3.6 in /opt/conda/lib/python3.8/site-packages (from scanpy) (3.7.1) Requirement already satisfied: pandas!=2.1.2,>=1.1.1 in /opt/conda/lib/python3.8/site-packages (from scanpy) (1.5.3) Requirement already satisfied: scikit-learn>=0.24 in /opt/conda/lib/python3.8/site-packages (from scanpy) (1.0.2) Requirement already satisfied: tqdm in /opt/conda/lib/python3.8/site-packages (from scanpy) (4.64.1) Collecting seaborn>=0.13.0 Downloading https://pypi.tuna.tsinghua.edu.cn/packages/83/11/00d3c3dfc25ad54e731d91449895a79e4bf2384dc3ac01809010ba88f6d5/seaborn-0.13.2-py3-none-any.whl (294 kB) ━━━━━━━━━━━━━━━━━━━━━━━━━━━━━━━━━━━━━━ 294.9/294.9 kB 29.9 MB/s eta 0:00:00 Requirement already satisfied: joblib in /opt/conda/lib/python3.8/site-packages (from scanpy) (1.2.0) Collecting session-info Downloading https://pypi.tuna.tsinghua.edu.cn/packages/94/e4/ea615bb8185a298b21df1ac52a4a5db4e3351823a218f47ef3f883def88c/session_info-1.0.0.tar.gz (24 kB) Preparing metadata (setup.py) ... done Requirement already satisfied: statsmodels>=0.10.0rc2 in /opt/conda/lib/python3.8/site-packages (from scanpy) (0.12.2) Requirement already satisfied: fonttools>=4.22.0 in /opt/conda/lib/python3.8/site-packages (from matplotlib>=3.6->scanpy) (4.38.0) Requirement already satisfied: python-dateutil>=2.7 in /opt/conda/lib/python3.8/site-packages (from matplotlib>=3.6->scanpy) (2.8.2) Requirement already satisfied: importlib-resources>=3.2.0 in /opt/conda/lib/python3.8/site-packages (from matplotlib>=3.6->scanpy) (5.2.0) Requirement already satisfied: cycler>=0.10 in /opt/conda/lib/python3.8/site-packages (from matplotlib>=3.6->scanpy) (0.11.0) Requirement already satisfied: pillow>=6.2.0 in /opt/conda/lib/python3.8/site-packages (from matplotlib>=3.6->scanpy) (9.4.0) Requirement already satisfied: kiwisolver>=1.0.1 in /opt/conda/lib/python3.8/site-packages (from matplotlib>=3.6->scanpy) (1.4.4) Requirement already satisfied: contourpy>=1.0.1 in /opt/conda/lib/python3.8/site-packages (from matplotlib>=3.6->scanpy) (1.0.5) Requirement already satisfied: pyparsing>=2.3.1 in /opt/conda/lib/python3.8/site-packages (from matplotlib>=3.6->scanpy) (3.0.9) Requirement already satisfied: llvmlite<0.40,>=0.39.0dev0 in /opt/conda/lib/python3.8/site-packages (from numba>=0.41.0->scanpy) (0.39.1) Requirement already satisfied: importlib-metadata in /opt/conda/lib/python3.8/site-packages (from numba>=0.41.0->scanpy) (6.0.0) Requirement already satisfied: setuptools in /opt/conda/lib/python3.8/site-packages (from numba>=0.41.0->scanpy) (65.6.3) Requirement already satisfied: pytz>=2020.1 in /opt/conda/lib/python3.8/site-packages (from pandas!=2.1.2,>=1.1.1->scanpy) (2022.7) Requirement already satisfied: threadpoolctl>=2.0.0 in /opt/conda/lib/python3.8/site-packages (from scikit-learn>=0.24->scanpy) (3.1.0) Requirement already satisfied: six in /opt/conda/lib/python3.8/site-packages (from patsy->scanpy) (1.16.0) Collecting pynndescent>=0.5 Downloading https://pypi.tuna.tsinghua.edu.cn/packages/d2/53/d23a97e0a2c690d40b165d1062e2c4ccc796be458a1ce59f6ba030434663/pynndescent-0.5.13-py3-none-any.whl (56 kB) ━━━━━━━━━━━━━━━━━━━━━━━━━━━━━━━━━━━━━━━━ 56.9/56.9 kB 21.0 MB/s eta 0:00:00 Collecting stdlib_list Downloading https://pypi.tuna.tsinghua.edu.cn/packages/13/d9/9085375f0d23a4896b307bf14dcc61b49ec8cc67cb33e06cf95bf3af3966/stdlib_list-0.10.0-py3-none-any.whl (79 kB) ━━━━━━━━━━━━━━━━━━━━━━━━━━━━━━━━━━━━━━━━ 79.8/79.8 kB 28.0 MB/s eta 0:00:00 Requirement already satisfied: zipp>=3.1.0 in /opt/conda/lib/python3.8/site-packages (from importlib-resources>=3.2.0->matplotlib>=3.6->scanpy) (3.14.0) Building wheels for collected packages: session-info Building wheel for session-info (setup.py) ... done Created wheel for session-info: filename=session_info-1.0.0-py3-none-any.whl size=8027 sha256=0c3f558b3bb66af4764a8c4d48d5be187f85102bd07e81c4d79c46ef2103fc9f Stored in directory: /root/.cache/pip/wheels/e0/aa/a4/5edeccf7aec07c1fef3da6cca9794c23a3cb6b2048fd87bd5b Successfully built session-info Installing collected packages: stdlib_list, get-annotations, session-info, seaborn, pynndescent, anndata, umap-learn, scanpy Attempting uninstall: seaborn Found existing installation: seaborn 0.11.2 Uninstalling seaborn-0.11.2: Successfully uninstalled seaborn-0.11.2 Successfully installed anndata-0.9.2 get-annotations-0.1.2 pynndescent-0.5.13 scanpy-1.9.8 seaborn-0.13.2 session-info-1.0.0 stdlib_list-0.10.0 umap-learn-0.5.6 WARNING: Running pip as the 'root' user can result in broken permissions and conflicting behaviour with the system package manager. It is recommended to use a virtual environment instead: https://pip.pypa.io/warnings/venv Looking in indexes: https://pypi.tuna.tsinghua.edu.cn/simple Collecting squidpy Downloading https://pypi.tuna.tsinghua.edu.cn/packages/d5/9d/8b7e83bcbb82abf27adc0953b7e71a4ae0a06cf046e8642f18655d81f6de/squidpy-1.2.3-py3-none-any.whl (180 kB) ━━━━━━━━━━━━━━━━━━━━━━━━━━━━━━━━━━━━━━━ 180.5/180.5 kB 5.6 MB/s eta 0:00:00 Requirement already satisfied: numpy>=1.21.6 in /opt/conda/lib/python3.8/site-packages (from squidpy) (1.22.4) Collecting docrep>=0.3.1 Downloading https://pypi.tuna.tsinghua.edu.cn/packages/44/8e/250fab1cafeea43f4eb11f1d64cd6313f639965ff62cb0d9da3883655781/docrep-0.3.2.tar.gz (33 kB) Preparing metadata (setup.py) ... done Collecting numba<0.56.0,>=0.55.0 Downloading https://pypi.tuna.tsinghua.edu.cn/packages/c7/4c/551e2105dcdd7fcd7b27ce6a232e6b082a8d254c09d4690dd54d8ec9356d/numba-0.55.2-cp38-cp38-manylinux2014_x86_64.manylinux_2_17_x86_64.whl (3.4 MB) ━━━━━━━━━━━━━━━━━━━━━━━━━━━━━━━━━━━━━━━━ 3.4/3.4 MB 54.5 MB/s eta 0:00:00a 0:00:01 Requirement already satisfied: anndata>=0.7.4 in /opt/conda/lib/python3.8/site-packages (from squidpy) (0.9.2) Requirement already satisfied: typing-extensions in /opt/conda/lib/python3.8/site-packages (from squidpy) (4.5.0) Requirement already satisfied: tifffile!=2022.4.22 in /opt/conda/lib/python3.8/site-packages (from squidpy) (2023.2.3) Collecting leidenalg>=0.8.2 Downloading https://pypi.tuna.tsinghua.edu.cn/packages/48/bb/28343e7f26cbacf355c754ef6be2f1efa8d6233b56a5be89c5bc8caca1d1/leidenalg-0.10.2-cp38-abi3-manylinux_2_17_x86_64.manylinux2014_x86_64.whl (2.0 MB) ━━━━━━━━━━━━━━━━━━━━━━━━━━━━━━━━━━━━━━━━ 2.0/2.0 MB 77.1 MB/s eta 0:00:00 Requirement already satisfied: scanpy>=1.8.0 in /opt/conda/lib/python3.8/site-packages (from squidpy) (1.9.8) Collecting dask-image>=0.5.0 Downloading https://pypi.tuna.tsinghua.edu.cn/packages/5a/01/fae6fac53a725e068d4b9b09769a301d52d8a6827770fb8fdd45536f1338/dask_image-2023.3.0-py2.py3-none-any.whl (41 kB) ━━━━━━━━━━━━━━━━━━━━━━━━━━━━━━━━━━━━━━━━ 41.9/41.9 kB 14.6 MB/s eta 0:00:00 Requirement already satisfied: fsspec>=2021.11.0 in /opt/conda/lib/python3.8/site-packages (from squidpy) (2023.1.0) Collecting zarr>=2.6.1 Downloading https://pypi.tuna.tsinghua.edu.cn/packages/ba/55/0f5ec28561a1698ac5c11edc5724f8c6d48d01baecf740ffd62107d95e7f/zarr-2.16.1-py3-none-any.whl (206 kB) ━━━━━━━━━━━━━━━━━━━━━━━━━━━━━━━━━━━━━━ 206.9/206.9 kB 54.2 MB/s eta 0:00:00 Requirement already satisfied: xarray>=0.16.1 in /opt/conda/lib/python3.8/site-packages (from squidpy) (2022.12.0) Requirement already satisfied: matplotlib!=3.6.1,>=3.3 in /opt/conda/lib/python3.8/site-packages (from squidpy) (3.7.1) Collecting matplotlib-scalebar>=0.8.0 Downloading https://pypi.tuna.tsinghua.edu.cn/packages/a9/9e/22930e3deb2c374f47c6633aff9f6f379f8c421ab868fff3b4f85eac8b8a/matplotlib_scalebar-0.8.1-py2.py3-none-any.whl (17 kB) Collecting omnipath>=1.0.5 Downloading https://pypi.tuna.tsinghua.edu.cn/packages/5d/f3/00971472499c8a76a8bfd38b987736a7eb06d6c2f20d537363b6b28dfcec/omnipath-1.0.8-py3-none-any.whl (68 kB) ━━━━━━━━━━━━━━━━━━━━━━━━━━━━━━━━━━━━━━━━ 68.9/68.9 kB 24.0 MB/s eta 0:00:00 Requirement already satisfied: cycler>=0.11.0 in /opt/conda/lib/python3.8/site-packages (from squidpy) (0.11.0) Requirement already satisfied: dask[array]>=2021.02.0 in /opt/conda/lib/python3.8/site-packages (from squidpy) (2022.2.1) Requirement already satisfied: aiohttp>=3.8.1 in /opt/conda/lib/python3.8/site-packages (from squidpy) (3.8.4) Requirement already satisfied: Pillow>=8.0.0 in /opt/conda/lib/python3.8/site-packages (from squidpy) (9.4.0) Requirement already satisfied: validators>=0.18.2 in /opt/conda/lib/python3.8/site-packages (from squidpy) (0.20.0) Requirement already satisfied: scikit-image>=0.19 in /opt/conda/lib/python3.8/site-packages (from squidpy) (0.19.3) Requirement already satisfied: scikit-learn>=0.24.0 in /opt/conda/lib/python3.8/site-packages (from squidpy) (1.0.2) Requirement already satisfied: tqdm>=4.50.2 in /opt/conda/lib/python3.8/site-packages (from squidpy) (4.64.1) Requirement already satisfied: statsmodels>=0.12.0 in /opt/conda/lib/python3.8/site-packages (from squidpy) (0.12.2) Requirement already satisfied: networkx>=2.6.0 in /opt/conda/lib/python3.8/site-packages (from squidpy) (3.0) Requirement already satisfied: pandas>=1.5.0 in /opt/conda/lib/python3.8/site-packages (from squidpy) (1.5.3) Requirement already satisfied: multidict<7.0,>=4.5 in /opt/conda/lib/python3.8/site-packages (from aiohttp>=3.8.1->squidpy) (6.0.4) Requirement already satisfied: frozenlist>=1.1.1 in /opt/conda/lib/python3.8/site-packages (from aiohttp>=3.8.1->squidpy) (1.3.3) Requirement already satisfied: attrs>=17.3.0 in /opt/conda/lib/python3.8/site-packages (from aiohttp>=3.8.1->squidpy) (22.1.0) Requirement already satisfied: yarl<2.0,>=1.0 in /opt/conda/lib/python3.8/site-packages (from aiohttp>=3.8.1->squidpy) (1.8.2) Requirement already satisfied: aiosignal>=1.1.2 in /opt/conda/lib/python3.8/site-packages (from aiohttp>=3.8.1->squidpy) (1.3.1) Requirement already satisfied: async-timeout<5.0,>=4.0.0a3 in /opt/conda/lib/python3.8/site-packages (from aiohttp>=3.8.1->squidpy) (4.0.2) Requirement already satisfied: charset-normalizer<4.0,>=2.0 in /opt/conda/lib/python3.8/site-packages (from aiohttp>=3.8.1->squidpy) (3.0.1) Requirement already satisfied: scipy>1.4 in /opt/conda/lib/python3.8/site-packages (from anndata>=0.7.4->squidpy) (1.7.3) Requirement already satisfied: packaging>=20 in /opt/conda/lib/python3.8/site-packages (from anndata>=0.7.4->squidpy) (23.0) Requirement already satisfied: natsort in /opt/conda/lib/python3.8/site-packages (from anndata>=0.7.4->squidpy) (5.5.0) Requirement already satisfied: h5py>=3 in /opt/conda/lib/python3.8/site-packages (from anndata>=0.7.4->squidpy) (3.1.0) Collecting pims>=0.4.1 Downloading https://pypi.tuna.tsinghua.edu.cn/packages/b8/02/5bf3639f5b77e9b183011c08541c5039ba3d04f5316c70312b48a8e003a9/pims-0.7.tar.gz (87 kB) ━━━━━━━━━━━━━━━━━━━━━━━━━━━━━━━━━━━━━━━━ 87.8/87.8 kB 31.8 MB/s eta 0:00:00 Preparing metadata (setup.py) ... done Requirement already satisfied: cloudpickle>=1.1.1 in /opt/conda/lib/python3.8/site-packages (from dask[array]>=2021.02.0->squidpy) (2.2.1) Requirement already satisfied: partd>=0.3.10 in /opt/conda/lib/python3.8/site-packages (from dask[array]>=2021.02.0->squidpy) (1.3.0) Requirement already satisfied: pyyaml>=5.3.1 in /opt/conda/lib/python3.8/site-packages (from dask[array]>=2021.02.0->squidpy) (6.0) Requirement already satisfied: toolz>=0.8.2 in /opt/conda/lib/python3.8/site-packages (from dask[array]>=2021.02.0->squidpy) (0.12.0) Requirement already satisfied: six in /opt/conda/lib/python3.8/site-packages (from docrep>=0.3.1->squidpy) (1.16.0) Collecting igraph<0.12,>=0.10.0 Downloading https://pypi.tuna.tsinghua.edu.cn/packages/37/c4/a74b406e98b8f6a91e291bb16d11ea85999414ad89033a77b28484bdb07a/igraph-0.11.6-cp38-cp38-manylinux_2_17_x86_64.manylinux2014_x86_64.whl (3.1 MB) ━━━━━━━━━━━━━━━━━━━━━━━━━━━━━━━━━━━━━━━━ 3.1/3.1 MB 51.9 MB/s eta 0:00:00:00:01 Requirement already satisfied: fonttools>=4.22.0 in /opt/conda/lib/python3.8/site-packages (from matplotlib!=3.6.1,>=3.3->squidpy) (4.38.0) Requirement already satisfied: contourpy>=1.0.1 in /opt/conda/lib/python3.8/site-packages (from matplotlib!=3.6.1,>=3.3->squidpy) (1.0.5) Requirement already satisfied: pyparsing>=2.3.1 in /opt/conda/lib/python3.8/site-packages (from matplotlib!=3.6.1,>=3.3->squidpy) (3.0.9) Requirement already satisfied: importlib-resources>=3.2.0 in /opt/conda/lib/python3.8/site-packages (from matplotlib!=3.6.1,>=3.3->squidpy) (5.2.0) Requirement already satisfied: kiwisolver>=1.0.1 in /opt/conda/lib/python3.8/site-packages (from matplotlib!=3.6.1,>=3.3->squidpy) (1.4.4) Requirement already satisfied: python-dateutil>=2.7 in /opt/conda/lib/python3.8/site-packages (from matplotlib!=3.6.1,>=3.3->squidpy) (2.8.2) Collecting llvmlite<0.39,>=0.38.0rc1 Downloading https://pypi.tuna.tsinghua.edu.cn/packages/36/93/6e1026f0640d413805064a2bd48fb4a1c103fafb545936efb2d1a604e68d/llvmlite-0.38.1-cp38-cp38-manylinux_2_17_x86_64.manylinux2014_x86_64.whl (34.5 MB) ━━━━━━━━━━━━━━━━━━━━━━━━━━━━━━━━━━━━━━━━ 34.5/34.5 MB 10.9 MB/s eta 0:00:0000:0100:01 Requirement already satisfied: setuptools in /opt/conda/lib/python3.8/site-packages (from numba<0.56.0,>=0.55.0->squidpy) (65.6.3) Requirement already satisfied: wrapt>=1.12.0 in /opt/conda/lib/python3.8/site-packages (from omnipath>=1.0.5->squidpy) (1.14.1) Requirement already satisfied: urllib3>=1.26.0 in /opt/conda/lib/python3.8/site-packages (from omnipath>=1.0.5->squidpy) (1.26.14) Requirement already satisfied: requests>=2.24.0 in /opt/conda/lib/python3.8/site-packages (from omnipath>=1.0.5->squidpy) (2.28.2) Collecting inflect>=4.1.0 Downloading https://pypi.tuna.tsinghua.edu.cn/packages/f7/e0/c5684d7c058d8f2a9210c322dee32bd025c11d19e5ba23c82ac9188253f9/inflect-7.4.0-py3-none-any.whl (34 kB) Requirement already satisfied: pytz>=2020.1 in /opt/conda/lib/python3.8/site-packages (from pandas>=1.5.0->squidpy) (2022.7) Requirement already satisfied: get-annotations in /opt/conda/lib/python3.8/site-packages (from scanpy>=1.8.0->squidpy) (0.1.2) Requirement already satisfied: session-info in /opt/conda/lib/python3.8/site-packages (from scanpy>=1.8.0->squidpy) (1.0.0) Requirement already satisfied: patsy in /opt/conda/lib/python3.8/site-packages (from scanpy>=1.8.0->squidpy) (0.5.3) Requirement already satisfied: joblib in /opt/conda/lib/python3.8/site-packages (from scanpy>=1.8.0->squidpy) (1.2.0) Requirement already satisfied: seaborn>=0.13.0 in /opt/conda/lib/python3.8/site-packages (from scanpy>=1.8.0->squidpy) (0.13.2) Requirement already satisfied: umap-learn>=0.3.10 in /opt/conda/lib/python3.8/site-packages (from scanpy>=1.8.0->squidpy) (0.5.6) Requirement already satisfied: imageio>=2.4.1 in /opt/conda/lib/python3.8/site-packages (from scikit-image>=0.19->squidpy) (2.9.0) Requirement already satisfied: PyWavelets>=1.1.1 in /opt/conda/lib/python3.8/site-packages (from scikit-image>=0.19->squidpy) (1.4.1) Requirement already satisfied: threadpoolctl>=2.0.0 in /opt/conda/lib/python3.8/site-packages (from scikit-learn>=0.24.0->squidpy) (3.1.0) Requirement already satisfied: decorator>=3.4.0 in /opt/conda/lib/python3.8/site-packages (from validators>=0.18.2->squidpy) (5.1.1) Collecting fasteners Downloading https://pypi.tuna.tsinghua.edu.cn/packages/61/bf/fd60001b3abc5222d8eaa4a204cd8c0ae78e75adc688f33ce4bf25b7fafa/fasteners-0.19-py3-none-any.whl (18 kB) Collecting numcodecs>=0.10.0 Downloading https://pypi.tuna.tsinghua.edu.cn/packages/22/c1/f0dfd6447e0abaa59a86e86a7227bb8dac21d1423952595b392fd2def2d0/numcodecs-0.12.1-cp38-cp38-manylinux_2_17_x86_64.manylinux2014_x86_64.whl (7.8 MB) ━━━━━━━━━━━━━━━━━━━━━━━━━━━━━━━━━━━━━━━━ 7.8/7.8 MB 25.3 MB/s eta 0:00:0000:0100:01 Collecting asciitree Downloading https://pypi.tuna.tsinghua.edu.cn/packages/2d/6a/885bc91484e1aa8f618f6f0228d76d0e67000b0fdd6090673b777e311913/asciitree-0.3.3.tar.gz (4.0 kB) Preparing metadata (setup.py) ... done Collecting texttable>=1.6.2 Downloading https://pypi.tuna.tsinghua.edu.cn/packages/24/99/4772b8e00a136f3e01236de33b0efda31ee7077203ba5967fcc76da94d65/texttable-1.7.0-py2.py3-none-any.whl (10 kB) Requirement already satisfied: zipp>=3.1.0 in /opt/conda/lib/python3.8/site-packages (from importlib-resources>=3.2.0->matplotlib!=3.6.1,>=3.3->squidpy) (3.14.0) Collecting typeguard>=4.0.1 Downloading https://pypi.tuna.tsinghua.edu.cn/packages/eb/de/be0ba39ee73760bf33329b7c6f95bc67e96593c69c881671e312538e24bb/typeguard-4.3.0-py3-none-any.whl (35 kB) Requirement already satisfied: more-itertools>=8.5.0 in /opt/conda/lib/python3.8/site-packages (from inflect>=4.1.0->omnipath>=1.0.5->squidpy) (9.0.0) Requirement already satisfied: locket in /opt/conda/lib/python3.8/site-packages (from partd>=0.3.10->dask[array]>=2021.02.0->squidpy) (1.0.0) Collecting slicerator>=0.9.8 Downloading https://pypi.tuna.tsinghua.edu.cn/packages/e8/ae/fa6cd331b364ad2bbc31652d025f5747d89cbb75576733dfdf8efe3e4d62/slicerator-1.1.0-py3-none-any.whl (10 kB) Requirement already satisfied: certifi>=2017.4.17 in /opt/conda/lib/python3.8/site-packages (from requests>=2.24.0->omnipath>=1.0.5->squidpy) (2022.12.7) Requirement already satisfied: idna<4,>=2.5 in /opt/conda/lib/python3.8/site-packages (from requests>=2.24.0->omnipath>=1.0.5->squidpy) (3.4) Requirement already satisfied: pynndescent>=0.5 in /opt/conda/lib/python3.8/site-packages (from umap-learn>=0.3.10->scanpy>=1.8.0->squidpy) (0.5.13) Requirement already satisfied: stdlib-list in /opt/conda/lib/python3.8/site-packages (from session-info->scanpy>=1.8.0->squidpy) (0.10.0) Collecting typing-extensions Downloading https://pypi.tuna.tsinghua.edu.cn/packages/26/9f/ad63fc0248c5379346306f8668cda6e2e2e9c95e01216d2b8ffd9ff037d0/typing_extensions-4.12.2-py3-none-any.whl (37 kB) Requirement already satisfied: importlib-metadata>=3.6 in /opt/conda/lib/python3.8/site-packages (from typeguard>=4.0.1->inflect>=4.1.0->omnipath>=1.0.5->squidpy) (6.0.0) Building wheels for collected packages: docrep, pims, asciitree Building wheel for docrep (setup.py) ... done Created wheel for docrep: filename=docrep-0.3.2-py3-none-any.whl size=19877 sha256=16ff22c7a17a79ca874ebf4bc180a4161f19af504e82c36a75d273d36bb29227 Stored in directory: /root/.cache/pip/wheels/a5/81/5b/c755b46823ca1550ef5d8bd9cda5261997890f614e9f4582e4 Building wheel for pims (setup.py) ... done Created wheel for pims: filename=PIMS-0.7-py3-none-any.whl size=84594 sha256=2a414b4955c734cce5e255f693a1ce2375f185219544556680e715d0b7f1e193 Stored in directory: /root/.cache/pip/wheels/ef/81/10/2b27a5dd4fe4003603540651e302eb72b5b87f79484c593405 Building wheel for asciitree (setup.py) ... done Created wheel for asciitree: filename=asciitree-0.3.3-py3-none-any.whl size=5034 sha256=0e5cc357af2d0e91dd82d177e990d41a1c0d158f59086da68aa882ba16a5db62 Stored in directory: /root/.cache/pip/wheels/1c/87/ff/03781f9931ea8e5e4e39b09971d3ce10a09aa4fd4cf94004e2 Successfully built docrep pims asciitree Installing collected packages: texttable, slicerator, asciitree, typing-extensions, numcodecs, llvmlite, igraph, fasteners, docrep, zarr, typeguard, pims, numba, leidenalg, matplotlib-scalebar, inflect, omnipath, dask-image, squidpy Attempting uninstall: typing-extensions Found existing installation: typing_extensions 4.5.0 Uninstalling typing_extensions-4.5.0: Successfully uninstalled typing_extensions-4.5.0 Attempting uninstall: llvmlite Found existing installation: llvmlite 0.39.1 Uninstalling llvmlite-0.39.1: Successfully uninstalled llvmlite-0.39.1 Attempting uninstall: typeguard Found existing installation: typeguard 2.7.1 Uninstalling typeguard-2.7.1: Successfully uninstalled typeguard-2.7.1 Attempting uninstall: numba Found existing installation: numba 0.56.4 Uninstalling numba-0.56.4: Successfully uninstalled numba-0.56.4 Attempting uninstall: inflect Found existing installation: inflect 2.1.0 Uninstalling inflect-2.1.0: Successfully uninstalled inflect-2.1.0 ERROR: pip's dependency resolver does not currently take into account all the packages that are installed. This behaviour is the source of the following dependency conflicts. rich 13.3.1 requires pygments<3.0.0,>=2.14.0, but you have pygments 2.11.2 which is incompatible. Successfully installed asciitree-0.3.3 dask-image-2023.3.0 docrep-0.3.2 fasteners-0.19 igraph-0.11.6 inflect-7.4.0 leidenalg-0.10.2 llvmlite-0.38.1 matplotlib-scalebar-0.8.1 numba-0.55.2 numcodecs-0.12.1 omnipath-1.0.8 pims-0.7 slicerator-1.1.0 squidpy-1.2.3 texttable-1.7.0 typeguard-4.3.0 typing-extensions-4.12.2 zarr-2.16.1 WARNING: Running pip as the 'root' user can result in broken permissions and conflicting behaviour with the system package manager. It is recommended to use a virtual environment instead: https://pip.pypa.io/warnings/venv
The dataset used in this tutorial consists of 1 tissue slides from 1 mouse and is provided by 10x Genomics Space Ranger 1.1.0. The dataset was pre-processed in Squidpy, which provides a loading function for this dataset. We shortly inspect the returned AnnData object.
Creating directory `/root/.cache/squidpy` try downloading from url https://ndownloader.figshare.com/files/26098397 ... this may take a while but only happens once
AnnData object with n_obs × n_vars = 2688 × 18078 obs: 'in_tissue', 'array_row', 'array_col', 'n_genes_by_counts', 'log1p_n_genes_by_counts', 'total_counts', 'log1p_total_counts', 'pct_counts_in_top_50_genes', 'pct_counts_in_top_100_genes', 'pct_counts_in_top_200_genes', 'pct_counts_in_top_500_genes', 'total_counts_mt', 'log1p_total_counts_mt', 'pct_counts_mt', 'n_counts', 'leiden', 'cluster' var: 'gene_ids', 'feature_types', 'genome', 'mt', 'n_cells_by_counts', 'mean_counts', 'log1p_mean_counts', 'pct_dropout_by_counts', 'total_counts', 'log1p_total_counts', 'n_cells', 'highly_variable', 'highly_variable_rank', 'means', 'variances', 'variances_norm' uns: 'cluster_colors', 'hvg', 'leiden', 'leiden_colors', 'neighbors', 'pca', 'rank_genes_groups', 'spatial', 'umap' obsm: 'X_pca', 'X_umap', 'spatial' varm: 'PCs' obsp: 'connectivities', 'distances'
Spatial domains in Squidpy
In this section, we will illustrate this approach with a pedagogical example using Squidpy, and then point to a more advanced algorithm to accomplish this task.
Let's work with the Visium dataset for the purpose of this example. In this case, we will be using the term "spot" referring to observations stored in the rows of the AnnData object.
First off, we want an algorithm that encodes similarity between observations in some coordinate space, such as gene expression space and spatial coordinates. A nearest neighbor graph is a reliable representation for this task. Let's compute the nearest neighbor graph in spatial coordinates and the nearest neighbor graph in PCA coordinates. As we can see based on adata.obsm['X_pca']
the PCA was already performed on the dataset, hence we can directly compute the KNN graph.
computing neighbors using 'X_pca' with n_pcs = 50 2024-10-06 12:25:47.172031: I tensorflow/core/platform/cpu_feature_guard.cc:193] This TensorFlow binary is optimized with oneAPI Deep Neural Network Library (oneDNN) to use the following CPU instructions in performance-critical operations: AVX2 AVX512F FMA To enable them in other operations, rebuild TensorFlow with the appropriate compiler flags. 2024-10-06 12:25:49.756254: W tensorflow/compiler/xla/stream_executor/platform/default/dso_loader.cc:64] Could not load dynamic library 'libnvinfer.so.7'; dlerror: libnvinfer.so.7: cannot open shared object file: No such file or directory; LD_LIBRARY_PATH: /usr/mpi/gcc/openmpi-4.1.0rc5/lib:/usr/local/nccl-rdma-sharp-plugins/lib:/usr/local/nvidia/lib64:/usr/local/cuda/lib64:/usr/local/nvidia/lib:/usr/local/nvidia/lib64 2024-10-06 12:25:49.757155: W tensorflow/compiler/xla/stream_executor/platform/default/dso_loader.cc:64] Could not load dynamic library 'libnvinfer_plugin.so.7'; dlerror: libnvinfer_plugin.so.7: cannot open shared object file: No such file or directory; LD_LIBRARY_PATH: /usr/mpi/gcc/openmpi-4.1.0rc5/lib:/usr/local/nccl-rdma-sharp-plugins/lib:/usr/local/nvidia/lib64:/usr/local/cuda/lib64:/usr/local/nvidia/lib:/usr/local/nvidia/lib64 2024-10-06 12:25:49.757171: W tensorflow/compiler/tf2tensorrt/utils/py_utils.cc:38] TF-TRT Warning: Cannot dlopen some TensorRT libraries. If you would like to use Nvidia GPU with TensorRT, please make sure the missing libraries mentioned above are installed properly. finished: added to `.uns['neighbors']` `.obsp['distances']`, distances for each pair of neighbors `.obsp['connectivities']`, weighted adjacency matrix (0:00:15) Creating graph using `grid` coordinates and `None` transform and `1` libraries. Adding `adata.obsp['spatial_connectivities']` `adata.obsp['spatial_distances']` `adata.uns['spatial_neighbors']` Finish (0:00:00)
Second, we want to identify communities (clusters) in both representations jointly. One straightforward way to do this is by simply adding the two graphs and compute leiden on the joint graph. We can also weight the importance of each graph based on an hyperparameters alpha
.
running Leiden clustering finished: found 17 clusters and added 'squidpy_domains', the cluster labels (adata.obs, categorical) (0:00:00)
Let's visualize the results with Squidpy. The first annotation (cluster
) is a cluster annotation based only on gene expression similarity.
We can see that such an approach is essentially "smoothing" the cluster annotations based on spatial distances. Despite it being a purely pedagogical approach, it has been used in practice {cite}Chen2022-oa
. We invite the reader to check out more principled approaches.
SpaGCN
The second approach we are showing in this tutorial is SpaGCN {cite}Hu2021-SpaGCN
. SpaGCN is a graph convolutional network approach that leverages gene expression, spatial location and histology in spatial omics data analysis. SpaGCN combines gene expression, spatial information and the histological images in an undirected weighted graph. This graph represents the overall spatial dependencies present in the data, which can be used in a graph convolutional approach to identify spatial domains.
We are now showing how to use SpaGCN in practice. We first load the respective additional packages:
Looking in indexes: https://pypi.tuna.tsinghua.edu.cn/simple Collecting SpaGCN Downloading https://pypi.tuna.tsinghua.edu.cn/packages/00/be/cb2977960965bd36dc38e7e61e8e43269af5e9eb6fe355a58fd46f1db098/SpaGCN-1.2.7-py3-none-any.whl (15 kB) Collecting louvain Downloading https://pypi.tuna.tsinghua.edu.cn/packages/a8/e9/a2f5402500d63cb903e84c26a916237383de07b53c3b135062010eabc83a/louvain-0.8.2-cp38-cp38-manylinux_2_17_x86_64.manylinux2014_x86_64.whl (1.1 MB) ━━━━━━━━━━━━━━━━━━━━━━━━━━━━━━━━━━━━━━━━ 1.1/1.1 MB 17.9 MB/s eta 0:00:00a 0:00:01 Requirement already satisfied: numba in /opt/conda/lib/python3.8/site-packages (from SpaGCN) (0.55.2) Requirement already satisfied: torch in /opt/conda/lib/python3.8/site-packages (from SpaGCN) (1.13.1+cu116) Requirement already satisfied: anndata in /opt/conda/lib/python3.8/site-packages (from SpaGCN) (0.9.2) Requirement already satisfied: scipy in /opt/conda/lib/python3.8/site-packages (from SpaGCN) (1.7.3) Requirement already satisfied: scikit-learn in /opt/conda/lib/python3.8/site-packages (from SpaGCN) (1.0.2) Collecting python-igraph Downloading https://pypi.tuna.tsinghua.edu.cn/packages/81/e6/03acdc349320e2ff3ed1e257d56c67907e66a6d0b442e54dc75e02ca108c/python_igraph-0.11.6-py3-none-any.whl (9.1 kB) Requirement already satisfied: pandas in /opt/conda/lib/python3.8/site-packages (from SpaGCN) (1.5.3) Requirement already satisfied: numpy in /opt/conda/lib/python3.8/site-packages (from SpaGCN) (1.22.4) Requirement already satisfied: scanpy in /opt/conda/lib/python3.8/site-packages (from SpaGCN) (1.9.8) Requirement already satisfied: natsort in /opt/conda/lib/python3.8/site-packages (from anndata->SpaGCN) (5.5.0) Requirement already satisfied: packaging>=20 in /opt/conda/lib/python3.8/site-packages (from anndata->SpaGCN) (23.0) Requirement already satisfied: h5py>=3 in /opt/conda/lib/python3.8/site-packages (from anndata->SpaGCN) (3.1.0) Requirement already satisfied: python-dateutil>=2.8.1 in /opt/conda/lib/python3.8/site-packages (from pandas->SpaGCN) (2.8.2) Requirement already satisfied: pytz>=2020.1 in /opt/conda/lib/python3.8/site-packages (from pandas->SpaGCN) (2022.7) Requirement already satisfied: igraph<0.12,>=0.10.0 in /opt/conda/lib/python3.8/site-packages (from louvain->SpaGCN) (0.11.6) Requirement already satisfied: setuptools in /opt/conda/lib/python3.8/site-packages (from numba->SpaGCN) (65.6.3) Requirement already satisfied: llvmlite<0.39,>=0.38.0rc1 in /opt/conda/lib/python3.8/site-packages (from numba->SpaGCN) (0.38.1) Requirement already satisfied: texttable>=1.6.2 in /opt/conda/lib/python3.8/site-packages (from igraph<0.12,>=0.10.0->louvain->SpaGCN) (1.7.0) Requirement already satisfied: seaborn>=0.13.0 in /opt/conda/lib/python3.8/site-packages (from scanpy->SpaGCN) (0.13.2) Requirement already satisfied: tqdm in /opt/conda/lib/python3.8/site-packages (from scanpy->SpaGCN) (4.64.1) Requirement already satisfied: joblib in /opt/conda/lib/python3.8/site-packages (from scanpy->SpaGCN) (1.2.0) Requirement already satisfied: patsy in /opt/conda/lib/python3.8/site-packages (from scanpy->SpaGCN) (0.5.3) Requirement already satisfied: session-info in /opt/conda/lib/python3.8/site-packages (from scanpy->SpaGCN) (1.0.0) Requirement already satisfied: umap-learn>=0.3.10 in /opt/conda/lib/python3.8/site-packages (from scanpy->SpaGCN) (0.5.6) Requirement already satisfied: statsmodels>=0.10.0rc2 in /opt/conda/lib/python3.8/site-packages (from scanpy->SpaGCN) (0.12.2) Requirement already satisfied: get-annotations in /opt/conda/lib/python3.8/site-packages (from scanpy->SpaGCN) (0.1.2) Requirement already satisfied: networkx>=2.3 in /opt/conda/lib/python3.8/site-packages (from scanpy->SpaGCN) (3.0) Requirement already satisfied: matplotlib>=3.6 in /opt/conda/lib/python3.8/site-packages (from scanpy->SpaGCN) (3.7.1) Requirement already satisfied: threadpoolctl>=2.0.0 in /opt/conda/lib/python3.8/site-packages (from scikit-learn->SpaGCN) (3.1.0) Requirement already satisfied: typing-extensions in /opt/conda/lib/python3.8/site-packages (from torch->SpaGCN) (4.12.2) Requirement already satisfied: contourpy>=1.0.1 in /opt/conda/lib/python3.8/site-packages (from matplotlib>=3.6->scanpy->SpaGCN) (1.0.5) Requirement already satisfied: importlib-resources>=3.2.0 in /opt/conda/lib/python3.8/site-packages (from matplotlib>=3.6->scanpy->SpaGCN) (5.2.0) Requirement already satisfied: kiwisolver>=1.0.1 in /opt/conda/lib/python3.8/site-packages (from matplotlib>=3.6->scanpy->SpaGCN) (1.4.4) Requirement already satisfied: pillow>=6.2.0 in /opt/conda/lib/python3.8/site-packages (from matplotlib>=3.6->scanpy->SpaGCN) (9.4.0) Requirement already satisfied: pyparsing>=2.3.1 in /opt/conda/lib/python3.8/site-packages (from matplotlib>=3.6->scanpy->SpaGCN) (3.0.9) Requirement already satisfied: cycler>=0.10 in /opt/conda/lib/python3.8/site-packages (from matplotlib>=3.6->scanpy->SpaGCN) (0.11.0) Requirement already satisfied: fonttools>=4.22.0 in /opt/conda/lib/python3.8/site-packages (from matplotlib>=3.6->scanpy->SpaGCN) (4.38.0) Requirement already satisfied: six>=1.5 in /opt/conda/lib/python3.8/site-packages (from python-dateutil>=2.8.1->pandas->SpaGCN) (1.16.0) Requirement already satisfied: pynndescent>=0.5 in /opt/conda/lib/python3.8/site-packages (from umap-learn>=0.3.10->scanpy->SpaGCN) (0.5.13) Requirement already satisfied: stdlib-list in /opt/conda/lib/python3.8/site-packages (from session-info->scanpy->SpaGCN) (0.10.0) Requirement already satisfied: zipp>=3.1.0 in /opt/conda/lib/python3.8/site-packages (from importlib-resources>=3.2.0->matplotlib>=3.6->scanpy->SpaGCN) (3.14.0) Installing collected packages: python-igraph, louvain, SpaGCN Successfully installed SpaGCN-1.2.7 louvain-0.8.2 python-igraph-0.11.6 WARNING: Running pip as the 'root' user can result in broken permissions and conflicting behaviour with the system package manager. It is recommended to use a virtual environment instead: https://pip.pypa.io/warnings/venv /opt/conda/lib/python3.8/site-packages/statsmodels/tsa/base/tsa_model.py:7: FutureWarning: pandas.Int64Index is deprecated and will be removed from pandas in a future version. Use pandas.Index with the appropriate dtype instead. from pandas import (to_datetime, Int64Index, DatetimeIndex, Period, /opt/conda/lib/python3.8/site-packages/statsmodels/tsa/base/tsa_model.py:7: FutureWarning: pandas.Float64Index is deprecated and will be removed from pandas in a future version. Use pandas.Index with the appropriate dtype instead. from pandas import (to_datetime, Int64Index, DatetimeIndex, Period,
As already mentioned, SpaGCN takes as additional input the histological image of the spatial dataset. For this purpose, we additionally load the high-resolution tif from the 10X Genomics website into our notebook. SpaGCN can also be used without histology information. We will refer to it at a later point.
/opt/conda/lib/python3.8/site-packages/PIL/Image.py:3167: DecompressionBombWarning: Image size (132748287 pixels) exceeds limit of 89478485 pixels, could be decompression bomb DOS attack. warnings.warn(
The spatial AnnData object in this tutorial was already processed. To ensure we are applying the correct data processing needed for SpaGCN, we are resetting adata.X
to the raw counts.
Integrate gene expression and histology into a Graph
SpaGCN requires passing the spatial array coordinates as well as the pixel coordinates to the model. The array coordinates are typically stored in adata.obs["array_row"]
and adata.obs["array_col"]
. The pixel coordinates are stored in adata.obsm["spatial"]
.
First SpaGCN aggregates the gene expression and histology information into a joint graph in the form of an adjacency matrix. Two spots are considered connected if they are physically close and they have similar histological features extracted from the image. The respective function requires the user to pass the x and y pixel spaces, the image and additionally two parameters: beta
and alpha
.
beta
determines the area of each spot when extracting the color intensity. This value can typically be obtained fromadata.uns['spatial']
. Typically, Visium spots have a size of 55 to 100 .alpha
determines the weight given to the histology image when calculating the Euclidean distance between spots.alpha=1
means the histology pixel intensity value has the same scale variance as the (x,y) coordinate.
Calculateing adj matrix using histology image... Var of c0,c1,c2 = 96.93674686223055 519.0133178897761 37.20274924909862 Var of x,y,z = 2928460.011122931 4665090.578837907 4665090.578837907
Preprocessing of gene expression data
Next, we perform a basic preprocessing strategy on the gene expression data by filtering genes that are expressed in fewer than three spots. Additionally, the counts are normalized and log transformed.
normalizing counts per cell finished (0:00:00)
Hyperparameters of SpaGCN
As a first step, SpaGCN finds the characteristic length scale . This parameter determines how rapidly the weight decays as a function of distance. To find , one first has to specify the parameter which describes the percentage of total expression contributed by neighborhoods. For Visium data, SpaGCN recommends p=0.5
. For data with smaller capture areas like Slide-seq V2 or MERFISH, it is recommended to choose a higher contribution value.
Run 1: l [0.01, 1000], p [0.0, 176.04695830342547] Run 2: l [0.01, 500.005], p [0.0, 38.50406265258789] Run 3: l [0.01, 250.0075], p [0.0, 7.22906494140625] Run 4: l [0.01, 125.00874999999999], p [0.0, 1.119886875152588] Run 5: l [62.509375, 125.00874999999999], p [0.07394278049468994, 1.119886875152588] Run 6: l [93.7590625, 125.00874999999999], p [0.4443991184234619, 1.119886875152588] Run 7: l [93.7590625, 109.38390625], p [0.4443991184234619, 0.7433689832687378] Run 8: l [93.7590625, 101.571484375], p [0.4443991184234619, 0.5843360424041748] Run 9: l [93.7590625, 97.66527343749999], p [0.4443991184234619, 0.5119975805282593] Run 10: l [95.71216796875, 97.66527343749999], p [0.47760796546936035, 0.5119975805282593] recommended l = 96.688720703125
If the number of spatial domains in the tissue is known, SpaGCN can calculate a suitable resolution to generate the respective number. This might be for example the case in brain samples, where one wants to find a certain number of cortex layers in the spatial slide. If the number of domains is not known, SpaGCN varies the resolution parameter from 0.2 to 0.1 and uses a resolution that results in the highest Silhouette score.
We will specify the number of clusters to the number of cell types present in our example dataset and set n_clusters=15
.
Start at res = 0.4 step = 0.1 Initializing cluster centers with louvain, resolution = 0.4 computing neighbors using data matrix X directly finished: added to `.uns['neighbors']` `.obsp['distances']`, distances for each pair of neighbors `.obsp['connectivities']`, weighted adjacency matrix (0:00:00) running Louvain clustering using the "louvain" package of Traag (2017) finished: found 10 clusters and added 'louvain', the cluster labels (adata.obs, categorical) (0:00:00) Epoch 0 Res = 0.4 Num of clusters = 10 Initializing cluster centers with louvain, resolution = 0.5 computing neighbors using data matrix X directly finished: added to `.uns['neighbors']` `.obsp['distances']`, distances for each pair of neighbors `.obsp['connectivities']`, weighted adjacency matrix (0:00:00) running Louvain clustering using the "louvain" package of Traag (2017) finished: found 13 clusters and added 'louvain', the cluster labels (adata.obs, categorical) (0:00:00) Epoch 0 Res = 0.5 Num of clusters = 13 Res changed to 0.5 Initializing cluster centers with louvain, resolution = 0.6 computing neighbors using data matrix X directly finished: added to `.uns['neighbors']` `.obsp['distances']`, distances for each pair of neighbors `.obsp['connectivities']`, weighted adjacency matrix (0:00:00) running Louvain clustering using the "louvain" package of Traag (2017) finished: found 14 clusters and added 'louvain', the cluster labels (adata.obs, categorical) (0:00:00) Epoch 0 Res = 0.6 Num of clusters = 14 Res changed to 0.6 Initializing cluster centers with louvain, resolution = 0.7 computing neighbors using data matrix X directly finished: added to `.uns['neighbors']` `.obsp['distances']`, distances for each pair of neighbors `.obsp['connectivities']`, weighted adjacency matrix (0:00:00) running Louvain clustering using the "louvain" package of Traag (2017) finished: found 16 clusters and added 'louvain', the cluster labels (adata.obs, categorical) (0:00:00) Epoch 0 Res = 0.7 Num of clusters = 16 Step changed to 0.05 Initializing cluster centers with louvain, resolution = 0.65 computing neighbors using data matrix X directly finished: added to `.uns['neighbors']` `.obsp['distances']`, distances for each pair of neighbors `.obsp['connectivities']`, weighted adjacency matrix (0:00:00) running Louvain clustering using the "louvain" package of Traag (2017) finished: found 15 clusters and added 'louvain', the cluster labels (adata.obs, categorical) (0:00:00) Epoch 0 Res = 0.65 Num of clusters = 15 recommended res = 0.65
We have now computed all required parameters and can initialize SpaGCN and set the hyperparameter.
Next, we train the model with the suited resolution to identify 15 spatial domains.
Initializing cluster centers with louvain, resolution = 0.65 computing neighbors using data matrix X directly finished: added to `.uns['neighbors']` `.obsp['distances']`, distances for each pair of neighbors `.obsp['connectivities']`, weighted adjacency matrix (0:00:00) running Louvain clustering using the "louvain" package of Traag (2017) finished: found 16 clusters and added 'louvain', the cluster labels (adata.obs, categorical) (0:00:00) Epoch 0 Epoch 10 Epoch 20 Epoch 30 Epoch 40 Epoch 50 Epoch 60 Epoch 70 delta_label 0.000744047619047619 < tol 0.001 Reach tolerance threshold. Stopping training. Total epoch: 79
We now predict the respective spatial domain for each cell in the dataset. Additionally, the model returns the probability of each cell belonging to one of the domains. We will not leverage this information in this tutorial.
We are saving the spatial domains now into adata.obs
and save it as a categorical for convenient plotting.
Let us inspect the result in a spatial scatter plot and compare it to the original annotations in the dataset.
As we can see, the method quite accurately identified spatial domains. Interestingly, these domains correspond quite well to the original annotation. However, we can observe a few outliers as some spots are still spread across the dataset and are not assigned to the same domain. SpaGCN provides a function to refine the spatial domains, which we will show now.
Refining the detected spatial domains
SpaGCN includes an optional refinement step to enhance the clustering result which inspects the domain assignment of each spot and its neighboring spots. In cases where more than half of the neighboring spots have been assigned to a different domain, the spot will be relabeled to the main domain of its neighboring spots. The refinement step will only impact a few spots. Generally, SpaGCN only recommends the refinement when the dataset is expected to have clear domain boundaries.
For the refinement, SpaGCN first calculated an adjacency matrix without accounting for the histological image.
Calculateing adj matrix using xy only...
This adjacency matrix is now used in the refinement together with the previously computed domains.
We are saving the refined spatial domains now into adata.obs
and save it as a categorical for convenient plotting.
Let us inspect the refined spatial domains compared to the original spatial domains.
As we can see the refined spatial domains do not show outliers, but clear boundaries between the different domains. As a next step, one could now annotate the identified spatial domains or use them to calculate spatially variable genes.
Key takeaways
Spatial domains are clusters that both reflecting similarity of spots or cells in terms of gene expression as well as spatial proximity
Methods for identifying spatial domains can also incorporate the histological information available through spatial omics technologies
We presented how to identify spatial domains in Squidpy by combining the nearest neighbor graph and the spatial proximity graph, as well as the usage of SpaGCN
References
:filter: docname in docnames
:labelprefix: spatial
Contributors
Authors
- Giovanni Palla
- Anna Schaar
Reviewers
- Lukas Heumos
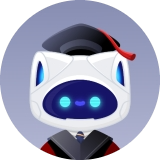
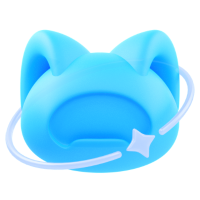
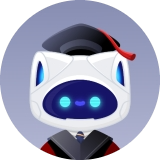
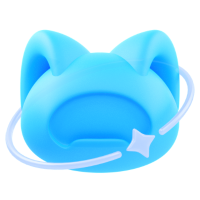