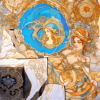
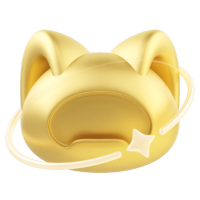
EIS 自动化拟合等效电路模型 ECM
Abstract
电化学系统的电化学阻抗谱 (EIS) 数据分析通常包括使用专家知识定义等效电路模型 (ECM),然后优化模型参数以解卷积各种电阻、电容、电感或扩散响应。对于小型数据集,此过程可以手动进行;但是,对于具有广泛 EIS 响应的大量数据集,手动定义适当的 ECM 是不可行的。自动识别 ECM 将大大加快大量 EIS 数据的分析。我们展示了机器学习方法,用于对 QuantumScape 为 BatteryDEV 黑客马拉松提供的 9,300 个阻抗谱的 ECM 进行分类。
本文分为两个模型的实现,第一个是 RF 模型,第二个是 CNN 模型
关于阻抗 EIS 预测 ECM 的benchmark文章参考:Machine Learning Benchmarks for the Classification of Equivalent Circuit Models from Electrochemical Impedance Spectra
Introduction
关于EIS以及等效电路模型有以下背景需要了解:
电化学阻抗谱(EIS)是一种常用于表征电池的非破坏性方法,它揭示了LiB内部的动态电极动力学过程。基于不同电化学过程表现出的时间常数,可以通过将阻抗谱的变化与固体电解质界面(SEI)生长、电荷转移和锂离子扩散等内部机制联系起来来表征电池性能。辅助研究工具包括等效电路模型(ECM)、弛豫时间分布(DRT)、阻抗机制模型等。 可以区分和量化多时间尺度特征,以更好地了解电池状态。已有工作显示2,借助机器学习方法,在电芯筛选和老化监测这两种情况下,根据阻抗可以可靠地量化关键的物理化学参数。 结果表明,电芯筛选时可以EIS准确估算电池电极的弯曲度、孔隙率和活性物质含量,误差小于2%;在老化监测方面,SEI电阻和电荷转移电阻可以准确估计,误差小于5% 。 上述应用表明EIS提供了对电池更全面的理解。
EIS 可以用于多种电话学系统的评估,如例如锂电池、燃料电池、超级电容器、腐蚀 或生物系统。对于电池,利用 EIS 的特定研究领域包括等效电路模型 (ECM) 表征、阻断电极实验以研究纯离子或电子行为、扩散过程建模、多孔电极表征、通过传输线建模进行电极表征以及电池性能监测。然而,为了分析这些光谱并指定特定的机制(如电子电阻、电荷转移、质量传输等),电化学家通常使用 ECM 来表示电池中的不同物理化学过程,通过电感、电阻、电容或它们的组合等电路元件对其进行参数化。定义 ECM 的结构通常需要专家判断,这意味着对大量 EIS 测量的评估是一个难以自动化的过程。
机器学习方法在电化学领域日益流行,特别是在EIS数据分析中,已成功应用于电池寿命预测和各种光谱数据分析。近期发展包括基于贝叶斯推理的EIS数据模型选择。尽管已有一些开源工具和数据集,但仍有巨大发展空间。本文通过分享由QuantumScape提供的大型合成EIS数据集和未标记数据集,为开源电池数据和软件做出贡献,重点使用ML方法准确分类等效电路模型(ECM)。这些数据集用于BatteryDEV黑客马拉松,旨在寻找ECM识别问题的跨学科解决方案。
加载工具包
设置随机数种子
<matplotlib.pyplot._IonContext at 0x7f07ff4df4c0>
RF model
构建估计函数
构建模型
运行 RF 模型
[0.42032517 0.41422605 0.39457023 0.40045123 0.40953975]
F1 score train: 1.000 F1 score test: 0.399 precision recall f1-score support L-R-RCPE 0.3399 0.3444 0.3421 151 L-R-RCPE-RCPE 0.2131 0.2021 0.2074 193 L-R-RCPE-RCPE-RCPE 0.2609 0.1842 0.2159 228 RC-G-G 0.6557 0.8734 0.7491 229 RC-RC-RCPE-RCPE 0.5833 0.7061 0.6389 228 RCPE-RCPE 0.2731 0.2731 0.2731 216 RCPE-RCPE-RCPE 0.2431 0.1947 0.2162 226 RCPE-RCPE-RCPE-RCPE 0.2648 0.2601 0.2624 223 Rs_Ws 0.6866 0.6866 0.6866 67 accuracy 0.3981 1761 macro avg 0.3912 0.4139 0.3991 1761 weighted avg 0.3714 0.3981 0.3808 1761 Done
CNN 模型
加载工具包
构建 CNN 架构
定义执行函数
定义多状态运行函数
执行模型运行
2.11.0 Filter: 1 Repeating the training 1 times. Found 7040 files belonging to 9 classes. Using 6336 files for training. Found 7040 files belonging to 9 classes. Using 704 files for validation. Found 1761 files belonging to 9 classes. Model: "sequential_1" _________________________________________________________________ Layer (type) Output Shape Param # ================================================================= rescaling_1 (Rescaling) (None, 58, 58, 1) 0 conv2d_5 (Conv2D) (None, 27, 27, 64) 2368 conv2d_6 (Conv2D) (None, 25, 25, 64) 36928 max_pooling2d_3 (MaxPooling (None, 12, 12, 64) 0 2D) batch_normalization_3 (Batc (None, 12, 12, 64) 256 hNormalization) conv2d_7 (Conv2D) (None, 10, 10, 128) 73856 conv2d_8 (Conv2D) (None, 8, 8, 128) 147584 max_pooling2d_4 (MaxPooling (None, 4, 4, 128) 0 2D) batch_normalization_4 (Batc (None, 4, 4, 128) 512 hNormalization) conv2d_9 (Conv2D) (None, 2, 2, 256) 295168 max_pooling2d_5 (MaxPooling (None, 1, 1, 256) 0 2D) batch_normalization_5 (Batc (None, 1, 1, 256) 1024 hNormalization) flatten_1 (Flatten) (None, 256) 0 dense_2 (Dense) (None, 512) 131584 dense_3 (Dense) (None, 9) 4617 ================================================================= Total params: 693,897 Trainable params: 693,001 Non-trainable params: 896 _________________________________________________________________ Epoch 1/200 198/198 [==============================] - 5s 9ms/step - loss: 2.1449 - accuracy: 0.2061 - val_loss: 2.2997 - val_accuracy: 0.1364 - lr: 0.0010 Epoch 2/200 198/198 [==============================] - 2s 7ms/step - loss: 2.0010 - accuracy: 0.2367 - val_loss: 2.1737 - val_accuracy: 0.1562 - lr: 0.0010 Epoch 3/200 198/198 [==============================] - 2s 7ms/step - loss: 1.8845 - accuracy: 0.2677 - val_loss: 1.8519 - val_accuracy: 0.2912 - lr: 0.0010 Epoch 4/200 198/198 [==============================] - 1s 7ms/step - loss: 1.8112 - accuracy: 0.2936 - val_loss: 1.7922 - val_accuracy: 0.3054 - lr: 0.0010 Epoch 5/200 198/198 [==============================] - 1s 7ms/step - loss: 1.7492 - accuracy: 0.3086 - val_loss: 1.7895 - val_accuracy: 0.3026 - lr: 0.0010 Epoch 6/200 198/198 [==============================] - 1s 7ms/step - loss: 1.6989 - accuracy: 0.3278 - val_loss: 1.7652 - val_accuracy: 0.3111 - lr: 0.0010 Epoch 7/200 198/198 [==============================] - 1s 7ms/step - loss: 1.6562 - accuracy: 0.3445 - val_loss: 1.7682 - val_accuracy: 0.3310 - lr: 0.0010 Epoch 8/200 198/198 [==============================] - 1s 7ms/step - loss: 1.6211 - accuracy: 0.3568 - val_loss: 1.7493 - val_accuracy: 0.3324 - lr: 0.0010 Epoch 9/200 198/198 [==============================] - 1s 7ms/step - loss: 1.5755 - accuracy: 0.3804 - val_loss: 1.8102 - val_accuracy: 0.3224 - lr: 0.0010 Epoch 10/200 198/198 [==============================] - 1s 7ms/step - loss: 1.5216 - accuracy: 0.3979 - val_loss: 2.0015 - val_accuracy: 0.2670 - lr: 0.0010 Epoch 11/200 193/198 [============================>.] - ETA: 0s - loss: 1.4858 - accuracy: 0.4137 Epoch 11: ReduceLROnPlateau reducing learning rate to 0.00020000000949949026. 198/198 [==============================] - 1s 7ms/step - loss: 1.4875 - accuracy: 0.4121 - val_loss: 1.8725 - val_accuracy: 0.3111 - lr: 0.0010 Epoch 12/200 198/198 [==============================] - 1s 7ms/step - loss: 1.3166 - accuracy: 0.4923 - val_loss: 2.2674 - val_accuracy: 0.2244 - lr: 2.0000e-04 Epoch 13/200 198/198 [==============================] - 1s 7ms/step - loss: 1.2452 - accuracy: 0.5180 - val_loss: 1.9390 - val_accuracy: 0.3210 - lr: 2.0000e-04 Epoch 14/200 193/198 [============================>.] - ETA: 0s - loss: 1.1811 - accuracy: 0.5492 Epoch 14: ReduceLROnPlateau reducing learning rate to 4.0000001899898055e-05. 198/198 [==============================] - 2s 7ms/step - loss: 1.1784 - accuracy: 0.5507 - val_loss: 1.8511 - val_accuracy: 0.3310 - lr: 2.0000e-04 Epoch 15/200 198/198 [==============================] - 2s 7ms/step - loss: 1.1248 - accuracy: 0.5735 - val_loss: 1.7421 - val_accuracy: 0.3580 - lr: 4.0000e-05 Epoch 16/200 198/198 [==============================] - 1s 7ms/step - loss: 1.0990 - accuracy: 0.5859 - val_loss: 1.7609 - val_accuracy: 0.3551 - lr: 4.0000e-05 Epoch 17/200 198/198 [==============================] - 1s 7ms/step - loss: 1.0782 - accuracy: 0.5939 - val_loss: 1.7784 - val_accuracy: 0.3480 - lr: 4.0000e-05 Epoch 18/200 192/198 [============================>.] - ETA: 0s - loss: 1.0690 - accuracy: 0.5972 Epoch 18: ReduceLROnPlateau reducing learning rate to 1e-05. 198/198 [==============================] - 1s 7ms/step - loss: 1.0648 - accuracy: 0.5993 - val_loss: 1.7926 - val_accuracy: 0.3494 - lr: 4.0000e-05 Epoch 19/200 198/198 [==============================] - 1s 7ms/step - loss: 1.0411 - accuracy: 0.6128 - val_loss: 1.7753 - val_accuracy: 0.3537 - lr: 1.0000e-05 Epoch 20/200 198/198 [==============================] - 2s 7ms/step - loss: 1.0294 - accuracy: 0.6184 - val_loss: 1.7799 - val_accuracy: 0.3594 - lr: 1.0000e-05 Epoch 21/200 198/198 [==============================] - 1s 7ms/step - loss: 1.0230 - accuracy: 0.6203 - val_loss: 1.7848 - val_accuracy: 0.3580 - lr: 1.0000e-05 Epoch 22/200 198/198 [==============================] - 1s 7ms/step - loss: 1.0194 - accuracy: 0.6228 - val_loss: 1.7880 - val_accuracy: 0.3509 - lr: 1.0000e-05 Epoch 23/200 198/198 [==============================] - 1s 7ms/step - loss: 1.0126 - accuracy: 0.6181 - val_loss: 1.7933 - val_accuracy: 0.3509 - lr: 1.0000e-05 Epoch 24/200 198/198 [==============================] - 1s 7ms/step - loss: 1.0123 - accuracy: 0.6269 - val_loss: 1.7965 - val_accuracy: 0.3494 - lr: 1.0000e-05 Epoch 25/200 198/198 [==============================] - 1s 7ms/step - loss: 1.0128 - accuracy: 0.6217 - val_loss: 1.8002 - val_accuracy: 0.3551 - lr: 1.0000e-05 Epoch 26/200 198/198 [==============================] - 2s 7ms/step - loss: 1.0011 - accuracy: 0.6312 - val_loss: 1.8028 - val_accuracy: 0.3551 - lr: 1.0000e-05 Epoch 27/200 198/198 [==============================] - 1s 7ms/step - loss: 0.9958 - accuracy: 0.6266 - val_loss: 1.8041 - val_accuracy: 0.3594 - lr: 1.0000e-05 Epoch 28/200 198/198 [==============================] - 2s 7ms/step - loss: 0.9896 - accuracy: 0.6365 - val_loss: 1.8085 - val_accuracy: 0.3537 - lr: 1.0000e-05 Epoch 29/200 198/198 [==============================] - 2s 7ms/step - loss: 0.9915 - accuracy: 0.6386 - val_loss: 1.8143 - val_accuracy: 0.3608 - lr: 1.0000e-05 Epoch 30/200 198/198 [==============================] - 2s 7ms/step - loss: 0.9815 - accuracy: 0.6370 - val_loss: 1.8165 - val_accuracy: 0.3622 - lr: 1.0000e-05 Epoch 31/200 198/198 [==============================] - 1s 7ms/step - loss: 0.9812 - accuracy: 0.6400 - val_loss: 1.8207 - val_accuracy: 0.3636 - lr: 1.0000e-05 Epoch 32/200 198/198 [==============================] - 1s 7ms/step - loss: 0.9765 - accuracy: 0.6373 - val_loss: 1.8205 - val_accuracy: 0.3636 - lr: 1.0000e-05 Epoch 33/200 198/198 [==============================] - 1s 7ms/step - loss: 0.9730 - accuracy: 0.6411 - val_loss: 1.8257 - val_accuracy: 0.3594 - lr: 1.0000e-05 Epoch 34/200 198/198 [==============================] - 2s 7ms/step - loss: 0.9683 - accuracy: 0.6447 - val_loss: 1.8304 - val_accuracy: 0.3651 - lr: 1.0000e-05 Epoch 35/200 198/198 [==============================] - 2s 7ms/step - loss: 0.9600 - accuracy: 0.6509 - val_loss: 1.8354 - val_accuracy: 0.3622 - lr: 1.0000e-05 Epoch 36/200 198/198 [==============================] - 1s 7ms/step - loss: 0.9566 - accuracy: 0.6471 - val_loss: 1.8387 - val_accuracy: 0.3594 - lr: 1.0000e-05 Epoch 37/200 198/198 [==============================] - 1s 7ms/step - loss: 0.9504 - accuracy: 0.6525 - val_loss: 1.8416 - val_accuracy: 0.3551 - lr: 1.0000e-05 Epoch 38/200 198/198 [==============================] - 1s 7ms/step - loss: 0.9483 - accuracy: 0.6533 - val_loss: 1.8458 - val_accuracy: 0.3608 - lr: 1.0000e-05 Epoch 39/200 198/198 [==============================] - 2s 7ms/step - loss: 0.9404 - accuracy: 0.6588 - val_loss: 1.8507 - val_accuracy: 0.3565 - lr: 1.0000e-05 Epoch 40/200 198/198 [==============================] - 1s 7ms/step - loss: 0.9408 - accuracy: 0.6577 - val_loss: 1.8532 - val_accuracy: 0.3565 - lr: 1.0000e-05 Epoch 41/200 198/198 [==============================] - 1s 7ms/step - loss: 0.9328 - accuracy: 0.6588 - val_loss: 1.8585 - val_accuracy: 0.3594 - lr: 1.0000e-05 Epoch 42/200 198/198 [==============================] - 1s 7ms/step - loss: 0.9278 - accuracy: 0.6610 - val_loss: 1.8623 - val_accuracy: 0.3608 - lr: 1.0000e-05 Epoch 43/200 198/198 [==============================] - 1s 7ms/step - loss: 0.9253 - accuracy: 0.6594 - val_loss: 1.8663 - val_accuracy: 0.3594 - lr: 1.0000e-05 Epoch 44/200 198/198 [==============================] - 1s 7ms/step - loss: 0.9193 - accuracy: 0.6637 - val_loss: 1.8664 - val_accuracy: 0.3594 - lr: 1.0000e-05 Epoch 45/200 198/198 [==============================] - 1s 7ms/step - loss: 0.9127 - accuracy: 0.6664 - val_loss: 1.8735 - val_accuracy: 0.3594 - lr: 1.0000e-05 Epoch 46/200 198/198 [==============================] - 1s 7ms/step - loss: 0.9110 - accuracy: 0.6690 - val_loss: 1.8764 - val_accuracy: 0.3565 - lr: 1.0000e-05 Epoch 47/200 198/198 [==============================] - 1s 7ms/step - loss: 0.9090 - accuracy: 0.6675 - val_loss: 1.8820 - val_accuracy: 0.3551 - lr: 1.0000e-05 Epoch 48/200 198/198 [==============================] - 1s 7ms/step - loss: 0.9090 - accuracy: 0.6730 - val_loss: 1.8864 - val_accuracy: 0.3494 - lr: 1.0000e-05 Epoch 49/200 198/198 [==============================] - 1s 7ms/step - loss: 0.8978 - accuracy: 0.6771 - val_loss: 1.8935 - val_accuracy: 0.3523 - lr: 1.0000e-05 Epoch 50/200 198/198 [==============================] - 1s 7ms/step - loss: 0.8965 - accuracy: 0.6753 - val_loss: 1.8995 - val_accuracy: 0.3452 - lr: 1.0000e-05 Epoch 51/200 198/198 [==============================] - 1s 7ms/step - loss: 0.8913 - accuracy: 0.6757 - val_loss: 1.9026 - val_accuracy: 0.3565 - lr: 1.0000e-05 Epoch 52/200 198/198 [==============================] - 1s 7ms/step - loss: 0.8865 - accuracy: 0.6791 - val_loss: 1.9083 - val_accuracy: 0.3452 - lr: 1.0000e-05 Epoch 53/200 198/198 [==============================] - 1s 7ms/step - loss: 0.8780 - accuracy: 0.6834 - val_loss: 1.9111 - val_accuracy: 0.3480 - lr: 1.0000e-05 Epoch 54/200 198/198 [==============================] - 1s 7ms/step - loss: 0.8734 - accuracy: 0.6843 - val_loss: 1.9166 - val_accuracy: 0.3480 - lr: 1.0000e-05 Epoch 55/200 198/198 [==============================] - 1s 7ms/step - loss: 0.8750 - accuracy: 0.6875 - val_loss: 1.9207 - val_accuracy: 0.3509 - lr: 1.0000e-05 Epoch 56/200 198/198 [==============================] - 1s 7ms/step - loss: 0.8720 - accuracy: 0.6880 - val_loss: 1.9271 - val_accuracy: 0.3494 - lr: 1.0000e-05 Epoch 57/200 198/198 [==============================] - 1s 7ms/step - loss: 0.8637 - accuracy: 0.6872 - val_loss: 1.9311 - val_accuracy: 0.3523 - lr: 1.0000e-05 Epoch 58/200 198/198 [==============================] - 1s 7ms/step - loss: 0.8599 - accuracy: 0.6869 - val_loss: 1.9357 - val_accuracy: 0.3537 - lr: 1.0000e-05 Epoch 59/200 198/198 [==============================] - 1s 7ms/step - loss: 0.8602 - accuracy: 0.6919 - val_loss: 1.9388 - val_accuracy: 0.3537 - lr: 1.0000e-05 Epoch 60/200 198/198 [==============================] - 1s 7ms/step - loss: 0.8539 - accuracy: 0.6866 - val_loss: 1.9399 - val_accuracy: 0.3523 - lr: 1.0000e-05 Epoch 61/200 198/198 [==============================] - 1s 7ms/step - loss: 0.8505 - accuracy: 0.6940 - val_loss: 1.9484 - val_accuracy: 0.3480 - lr: 1.0000e-05 Epoch 62/200 198/198 [==============================] - 1s 7ms/step - loss: 0.8446 - accuracy: 0.6963 - val_loss: 1.9534 - val_accuracy: 0.3480 - lr: 1.0000e-05 Epoch 63/200 198/198 [==============================] - 1s 7ms/step - loss: 0.8392 - accuracy: 0.7009 - val_loss: 1.9591 - val_accuracy: 0.3480 - lr: 1.0000e-05 Epoch 64/200 198/198 [==============================] - 1s 7ms/step - loss: 0.8407 - accuracy: 0.7025 - val_loss: 1.9635 - val_accuracy: 0.3466 - lr: 1.0000e-05 Epoch 65/200 198/198 [==============================] - 1s 7ms/step - loss: 0.8317 - accuracy: 0.7038 - val_loss: 1.9682 - val_accuracy: 0.3494 - lr: 1.0000e-05 Epoch 66/200 198/198 [==============================] - 1s 7ms/step - loss: 0.8245 - accuracy: 0.7098 - val_loss: 1.9708 - val_accuracy: 0.3452 - lr: 1.0000e-05 Epoch 67/200 198/198 [==============================] - 1s 7ms/step - loss: 0.8246 - accuracy: 0.7077 - val_loss: 1.9767 - val_accuracy: 0.3494 - lr: 1.0000e-05 Epoch 68/200 198/198 [==============================] - 1s 7ms/step - loss: 0.8164 - accuracy: 0.7121 - val_loss: 1.9843 - val_accuracy: 0.3438 - lr: 1.0000e-05 Epoch 69/200 198/198 [==============================] - 1s 7ms/step - loss: 0.8128 - accuracy: 0.7128 - val_loss: 1.9858 - val_accuracy: 0.3466 - lr: 1.0000e-05 Epoch 70/200 198/198 [==============================] - 1s 7ms/step - loss: 0.8135 - accuracy: 0.7094 - val_loss: 1.9941 - val_accuracy: 0.3438 - lr: 1.0000e-05 Epoch 71/200 198/198 [==============================] - 1s 7ms/step - loss: 0.8060 - accuracy: 0.7153 - val_loss: 1.9960 - val_accuracy: 0.3452 - lr: 1.0000e-05 Epoch 72/200 198/198 [==============================] - 1s 7ms/step - loss: 0.8025 - accuracy: 0.7145 - val_loss: 2.0003 - val_accuracy: 0.3438 - lr: 1.0000e-05 Epoch 73/200 198/198 [==============================] - 1s 7ms/step - loss: 0.7939 - accuracy: 0.7173 - val_loss: 2.0047 - val_accuracy: 0.3438 - lr: 1.0000e-05 Epoch 74/200 198/198 [==============================] - 1s 7ms/step - loss: 0.7944 - accuracy: 0.7164 - val_loss: 2.0107 - val_accuracy: 0.3409 - lr: 1.0000e-05 Epoch 75/200 198/198 [==============================] - 1s 7ms/step - loss: 0.7919 - accuracy: 0.7183 - val_loss: 2.0178 - val_accuracy: 0.3452 - lr: 1.0000e-05 Epoch 76/200 198/198 [==============================] - 1s 7ms/step - loss: 0.7853 - accuracy: 0.7244 - val_loss: 2.0250 - val_accuracy: 0.3395 - lr: 1.0000e-05 Epoch 77/200 198/198 [==============================] - 1s 7ms/step - loss: 0.7788 - accuracy: 0.7259 - val_loss: 2.0272 - val_accuracy: 0.3423 - lr: 1.0000e-05 Epoch 78/200 198/198 [==============================] - 1s 7ms/step - loss: 0.7839 - accuracy: 0.7229 - val_loss: 2.0356 - val_accuracy: 0.3409 - lr: 1.0000e-05 Epoch 79/200 198/198 [==============================] - 1s 7ms/step - loss: 0.7741 - accuracy: 0.7287 - val_loss: 2.0390 - val_accuracy: 0.3423 - lr: 1.0000e-05 Epoch 80/200 198/198 [==============================] - 1s 7ms/step - loss: 0.7696 - accuracy: 0.7276 - val_loss: 2.0442 - val_accuracy: 0.3423 - lr: 1.0000e-05 Epoch 81/200 198/198 [==============================] - 1s 7ms/step - loss: 0.7638 - accuracy: 0.7304 - val_loss: 2.0492 - val_accuracy: 0.3452 - lr: 1.0000e-05 Epoch 82/200 198/198 [==============================] - 1s 7ms/step - loss: 0.7576 - accuracy: 0.7334 - val_loss: 2.0502 - val_accuracy: 0.3438 - lr: 1.0000e-05 Epoch 83/200 198/198 [==============================] - 1s 7ms/step - loss: 0.7557 - accuracy: 0.7393 - val_loss: 2.0601 - val_accuracy: 0.3438 - lr: 1.0000e-05 Epoch 84/200 198/198 [==============================] - 1s 7ms/step - loss: 0.7557 - accuracy: 0.7366 - val_loss: 2.0622 - val_accuracy: 0.3452 - lr: 1.0000e-05 Epoch 85/200 198/198 [==============================] - 1s 7ms/step - loss: 0.7437 - accuracy: 0.7413 - val_loss: 2.0712 - val_accuracy: 0.3409 - lr: 1.0000e-05 Epoch 86/200 198/198 [==============================] - 1s 7ms/step - loss: 0.7436 - accuracy: 0.7369 - val_loss: 2.0714 - val_accuracy: 0.3494 - lr: 1.0000e-05 Epoch 87/200 198/198 [==============================] - 1s 7ms/step - loss: 0.7346 - accuracy: 0.7465 - val_loss: 2.0790 - val_accuracy: 0.3438 - lr: 1.0000e-05 Epoch 88/200 198/198 [==============================] - 1s 7ms/step - loss: 0.7347 - accuracy: 0.7473 - val_loss: 2.0897 - val_accuracy: 0.3452 - lr: 1.0000e-05 Epoch 89/200 198/198 [==============================] - 1s 7ms/step - loss: 0.7295 - accuracy: 0.7442 - val_loss: 2.0915 - val_accuracy: 0.3452 - lr: 1.0000e-05 Epoch 90/200 198/198 [==============================] - 1s 7ms/step - loss: 0.7300 - accuracy: 0.7451 - val_loss: 2.0987 - val_accuracy: 0.3423 - lr: 1.0000e-05 Epoch 91/200 198/198 [==============================] - 2s 8ms/step - loss: 0.7197 - accuracy: 0.7511 - val_loss: 2.1026 - val_accuracy: 0.3423 - lr: 1.0000e-05 Epoch 92/200 198/198 [==============================] - 1s 7ms/step - loss: 0.7160 - accuracy: 0.7516 - val_loss: 2.1100 - val_accuracy: 0.3409 - lr: 1.0000e-05 Epoch 93/200 198/198 [==============================] - 2s 8ms/step - loss: 0.7131 - accuracy: 0.7551 - val_loss: 2.1166 - val_accuracy: 0.3409 - lr: 1.0000e-05 Epoch 94/200 194/198 [============================>.] - ETA: 0s - loss: 0.7071 - accuracy: 0.7542Restoring model weights from the end of the best epoch: 34. 198/198 [==============================] - 1s 7ms/step - loss: 0.7074 - accuracy: 0.7538 - val_loss: 2.1234 - val_accuracy: 0.3452 - lr: 1.0000e-05 Epoch 94: early stopping 1/1 [==============================] - 0s 358ms/step 198/198 [==============================] - 1s 4ms/step
Test Accuracy: 0.36838940113856067 F1 score train: 0.107 F1 score test: 0.368 precision recall f1-score support L-R-RCPE 0.3598 0.3907 0.3746 151 L-R-RCPE-RCPE 0.2000 0.2435 0.2196 193 L-R-RCPE-RCPE-RCPE 0.1887 0.1316 0.1550 228 RC-G-G 0.6543 0.6943 0.6737 229 RC-RC-RCPE-RCPE 0.4868 0.5658 0.5233 228 RCPE-RCPE 0.2767 0.2639 0.2701 216 RCPE-RCPE-RCPE 0.1596 0.1327 0.1449 226 RCPE-RCPE-RCPE-RCPE 0.2028 0.1928 0.1977 223 Rs_Ws 0.6629 0.8806 0.7564 67 accuracy 0.3481 1761 macro avg 0.3546 0.3884 0.3684 1761 weighted avg 0.3306 0.3481 0.3372 1761 Done
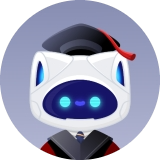
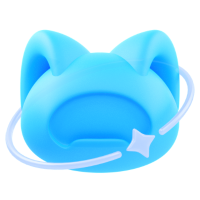
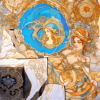
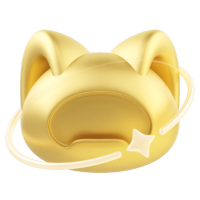
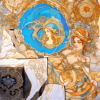
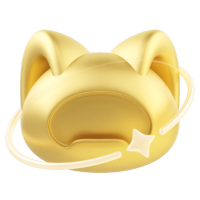