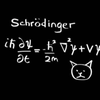
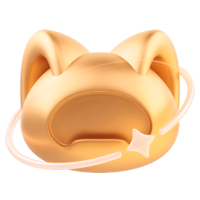
硫族化合物固态电解质通用力场横向测试
1. 通用力场整体介绍 Overview of Universal Force Fields
在探索固态电解质的性能和稳定性时,准确的原子间势能模型(IAP)是至关重要的。硫族化合物作为固态电解质的一种重要类型,因其在能源存储和转换设备中的应用潜力而受到广泛关注。为了有效地模拟这些材料的性质和行为,需要一个能够广泛适用于硫族化合物的通用力场。
- CHGNet (Crystal Hamiltonian Graph Neural Network)
CHGNet是一种基于图神经网络(GNN)的机器学习原子间势能模型(MLIP),由加州大学伯克利分校的研究团队开发。它专为解决原子建模中的电子相互作用问题而设计,特别是在处理含有异价离子的固态材料时。CHGNet在训练过程中明确包含了磁矩信息,这使得模型能够学习和准确地表示电子的轨道占据情况,从而增强了描述原子和电子自由度的能力。CHGNet通过结合磁矩作为电荷状态的约束,提供了对材料建模中额外电子自由度的洞察,这些自由度在以往的MLIPs中是无法观察到的。
- M3GNet (Materials Graph Network with Many-Body Interactions):
M3GNet是由Materials Virtual Lab开发的基于图神经网络的通用原子间势能模型,旨在为周期表中的所有元素提供一种通用的IAP。M3GNet通过结合传统IAPs中的多体特征和灵活的图材料表示,使用Materials Project数据库中的大量结构弛豫数据进行训练。该模型能够准确预测能量、力和应力,并且在晶体结构的声子和弹性计算、结构弛豫等方面具有广泛的应用。M3GNet的一个关键特点是它的可扩展性,可以轻松地应用于多组分化学体系,而不需要重新训练。此外,M3GNet还能够作为其他结构探索技术(如进化算法和生成模型)的替代模型,用于生成更多样化和不受限制的候选结构。
尽管CHGNet和M3GNet都是在原子尺度模拟领域的重要进展,为材料科学研究提供了强大的工具,但它们各自也有一些局限性。例如,CHGNet在处理氧化态的表示时,依赖于预先定义的氧化态,这可能限制了它在处理复杂材料系统中的适用性。而M3GNet虽然在预测能量、力和应力方面表现出色,但其训练数据主要集中在优化轨迹和平衡结构上,可能无法充分捕捉到高温下材料动态行为的复杂性。
相比之下,DPA-2模型通过采用先进的深度学习技术,能够更全面地捕捉材料系统的多体相互作用和动态性质。DPA-2的设计理念是在保持计算效率的同时,提供与第一性原理计算相当的精度,这使得它在模拟长时间尺度和大规模系统的动态行为时具有显著优势。此外,DPA-2模型的泛化能力较强,能够适应更广泛的温度和压力条件,为研究者提供了一个更为灵活和全面的材料模拟平台。
总的来说,CHGNet和M3GNet在特定领域内展现了强大的性能,但在某些方面仍有提升空间。而DPA-2模型则在这些方面提供了改进的潜力,为未来的材料模拟研究开辟了新的可能性。随着模型的进一步发展和优化,我们期待DPA-2能够在材料科学和计算化学领域发挥更大的作用。
Accurate interatomic potential models (IAPs) are critical when exploring the performance and stability of solid-state electrolytes. Chalcogenides, as an important type of solid-state electrolytes, have attracted widespread attention due to their potential applications in energy storage and conversion devices. In order to effectively simulate the properties and behaviors of these materials, a general force field that can be widely applicable to chalcogenides is needed.
- CHGNet
CHGNet is a machine learning interatomic potential model (MLIP) based on graph neural networks (GNNs), developed by a research team at the University of California, Berkeley. It is designed to address the problem of electron interactions in atomistic modeling, especially when dealing with solid-state materials containing heterovalent ions. CHGNet explicitly includes magnetic moment information during training, which enables the model to learn and accurately represent the orbital occupancy of electrons, thereby enhancing the ability to describe atomic and electronic degrees of freedom. By incorporating magnetic moments as constraints on charge states, CHGNet provides insights into additional electronic degrees of freedom in material modeling that are not observable in previous MLIPs.
- M3GNet
M3GNet is a universal interatomic potential model based on graph neural networks developed by Materials Virtual Lab, aiming to provide a universal IAP for all elements in the periodic table. M3GNet combines the multi-body features in traditional IAPs with flexible graph material representations, and is trained using a large amount of structural relaxation data from the Materials Project database. The model can accurately predict energies, forces, and stresses, and has a wide range of applications in phonon and elastic calculations of crystal structures, structural relaxation, etc. A key feature of M3GNet is its scalability, which can be easily applied to multi-component chemical systems without retraining. In addition, M3GNet can also serve as an alternative model to other structural exploration techniques (such as evolutionary algorithms and generative models) for generating more diverse and unrestricted candidate structures.
Although both CHGNet and M3GNet are important advances in the field of atomic-scale simulations and provide powerful tools for materials science research, they each have some limitations. For example, CHGNet relies on pre-defined oxidation states when dealing with the representation of oxidation states, which may limit its applicability in dealing with complex material systems. While M3GNet performs well in predicting energy, force, and stress, its training data is mainly focused on optimizing trajectories and equilibrium structures, which may not fully capture the complexity of the dynamic behavior of materials at high temperatures.
In contrast, the DPA-2 model can more comprehensively capture the multi-body interactions and dynamic properties of material systems by adopting advanced deep learning techniques. The design concept of DPA-2 is to provide accuracy comparable to first-principles calculations while maintaining computational efficiency, which gives it a significant advantage in simulating the dynamic behavior of long time scales and large-scale systems. In addition, the DPA-2 model has strong generalization ability and can adapt to a wider range of temperature and pressure conditions, providing researchers with a more flexible and comprehensive material simulation platform.
In general, CHGNet and M3GNet have shown strong performance in specific areas, but there is still room for improvement in some aspects. The DPA-2 model provides the potential for improvement in these aspects, opening up new possibilities for future material simulation research. With further development and optimization of the model, we expect DPA-2 to play a greater role in materials science and computational chemistry.
- 参考文献 References
- Chen, C., Ong, S.P. A universal graph deep learning interatomic potential for the periodic table. Nat Comput Sci 2, 718–728 (2022). https://doi.org/10.1038/s43588-022-00349-3
- Deng, B., Zhong, P., Jun, K. et al. CHGNet as a pretrained universal neural network potential for charge-informed atomistic modelling. Nat Mach Intell 5, 1031–1041 (2023). https://doi.org/10.1038/s42256-023-00716-3
2. 通用力场精度评测 Precision Evaluation of Universal Force Fields
2.1 平衡态数据集 Near-Equilibrium Datasets
由于通用力场往往只有平衡态附近的结构作为初始训练集,为了保证测试的公开公正,增加了平衡态构型作为测试集进行比较。 直接采用MP的结构,采用PBE/PBEsol分别run 10 步AIMD (50 K) 得到6个体系(LXPS,LPSX)的测试集,分别采用DPA-2,CHGNET和M3GNET测试 DPMD相比于通用力场,能量精度高1到2个数量级,力精度高2到5倍
下表中平衡位置附近结构中,DPA-2与CHGNET和M3GNET的对比
CHGNET | LGPS | LPSBr | LPSCl | LPSI | LSiPS | LSnPS |
---|---|---|---|---|---|---|
Energy MAE (meV/atom) | 240.7 | 220.2 | 233.1 | 201.1 | 232.2 | 244.8 |
Force MAE (meV/Å) | 27.9 | 31.9 | 29.8 | 39.4 | 26.1 | 35.8 |
Energy RMSE (meV/atom) | 240.7 | 220.2 | 233.1 | 201.1 | 232.2 | 244.8 |
Force RMSE (meV/Å) | 28.1 | 55.2 | 51.6 | 68.2 | 26.4 | 39.0 |
M3GNET | LGPS | LPSBr | LPSCl | LPSI | LSiPS | LSnPS |
---|---|---|---|---|---|---|
Energy MAE (meV/atom) | 4.9 | 2.5 | 14.5 | 0.6 | 7.9 | 3.5 |
Force MAE (meV/Å) | 18.6 | 18.7 | 19.8 | 22.9 | 34.7 | 37 |
Energy RMSE (meV/atom) | 4.9 | 2.5 | 14.5 | 0.6 | 7.9 | 3.5 |
Force RMSE (meV/Å) | 23.9 | 32.3 | 34.3 | 39.7 | 39.3 | 38.2 |
DPA-2 | LGPS | LPSBr | LPSCl | LPSI | LSiPS | LSnPS |
---|---|---|---|---|---|---|
Energy MAE (meV/atom) | 1.0 | 0.6 | 0.1 | 1.1 | 1.0 | 1.5 |
Force MAE (meV/Å) | 5.8 | 4.9 | 4.1 | 5.3 | 6.9 | 9.2 |
Energy RMSE (meV/atom) | 1.0 | 0.6 | 0.1 | 1.1 | 1.0 | 1.5 |
Force RMSE (meV/Å) | 8.3 | 6.5 | 5.5 | 8.6 | 10.0 | 14.2 |
为了notebook的简洁,下面我们用Li-Ge-P-S体系进行展示通用力场的计算。
M3GNET和CHGNET的特点都是内部嵌入了ase的部分模块。而我们的数据集是deepmd/npy格式的,因此需要用dpdata读出并记录DFT的标签,然后转成ase的格式并且调用M3GNET/CHGNET进行能量、受力的预测
For the simplicity of the notebook, we will use the Li-Ge-P-S system to demonstrate the calculation of the general force field.
The characteristics of M3GNET and CHGNET are that some modules of ase are embedded internally. Our data set is in deepmd/npy format, so we need to use dpdata to read and record the DFT label, then convert it to ase format and call M3GNET/CHGNET to predict energy and force.
. ├── LGePS ├── chgnet_test.json └── m3gnet_test.json 1 directory, 2 files
0
M3GNET
| Dataset | MAE (Energy)[meV/atom] | MAE (Force)[meV/ang.] | RMSE (Energy)[meV/atom] | RMSE (Force)[meV/ang.] | |:----------|-------------------------:|------------------------:|--------------------------:|-------------------------:| | LGePS | 4.9 | 18.6 | 4.9 | 23.9 |
CHGNET
| Dataset | MAE (Energy)[meV/atom] | MAE (Force)[meV/ang.] | RMSE (Energy)[meV/atom] | RMSE (Force)[meV/ang.] | |:----------|-------------------------:|------------------------:|--------------------------:|-------------------------:| | LGePS | 240.7 | 27.9 | 240.7 | 28.1 |
2.2 DPMD升温轨迹数据集 Heating Trajectories form DPMD
在DPMD的升温轨迹(150~1150 K)中挑选100帧结构,进行单点计算(PBE-sol/PBE),得到6个体系(LXPS,LPSX)的测试集,进行测试。
100 frames were selected from the DPMD heating trajectory (150-1150 K) for single-point calculations (PBE-sol for DP and PBE for CHGNET/M3GNET) to obtain a test set of 6 systems (LXPS, LPSX) for testing.
CHGNET | LGPS | LPSBr | LPSCl | LPSI | LSiPS | LSnPS |
---|---|---|---|---|---|---|
Energy MAE (meV/atom) | 288.2 | 261.9 | 270.9 | 242.2 | 277.5 | 288.7 |
Force MAE (meV/Å) | 118.6 | 105.3 | 23.8 | 119.3 | 44.6 | 53.3 |
Energy RMSE (meV/atom) | 288.9 | 262.6 | 271.5 | 243.1 | 278.1 | 289.3 |
Force RMSE (meV/Å) | 133.1 | 105.6 | 31.1 | 141 | 52.5 | 59.5 |
M3GNET | LGPS | LPSBr | LPSCl | LPSI | LSiPS | LSnPS |
---|---|---|---|---|---|---|
Energy MAE (meV/atom) | 48.6 | 34.7 | 33.9 | 30.4 | 40.4 | 52.7 |
Force MAE (meV/Å) | 83.1 | 109.4 | 44.5 | 115.7 | 38.4 | 65.9 |
Energy RMSE (meV/atom) | 53.5 | 41.3 | 39.5 | 37.3 | 45.6 | 57.2 |
Force RMSE (meV/Å) | 95.6 | 109.5 | 45.4 | 135.2 | 53.8 | 85.7 |
DPA-2 | LGPS | LPSBr | LPSCl | LPSI | LSiPS | LSnPS |
---|---|---|---|---|---|---|
Energy MAE (meV/atom) | 1.6 | 0.9 | 1.6 | 0.7 | 1.4 | 2.7 |
Force MAE (meV/Å) | 27.8 | 27.9 | 30.3 | 24.2 | 27.1 | 32.2 |
Energy RMSE (meV/atom) | 1.9 | 1.2 | 1.8 | 0.8 | 1.6 | 3 |
Force RMSE (meV/Å) | 38 | 37.6 | 41.1 | 32.9 | 36.9 | 43.9 |
. ├── LGPS ├── chgnet_test.json └── m3gnet_test.json 1 directory, 2 files
0
M3GNET
| Dataset | MAE (Energy)[meV/atom] | MAE (Force)[meV/ang.] | RMSE (Energy)[meV/atom] | RMSE (Force)[meV/ang.] | |:----------|-------------------------:|------------------------:|--------------------------:|-------------------------:| | LGPS | 48.6 | 83.1 | 53.5 | 95.6 |
CHGNET
| Dataset | MAE (Energy)[meV/atom] | MAE (Force)[meV/ang.] | RMSE (Energy)[meV/atom] | RMSE (Force)[meV/ang.] | |:----------|-------------------------:|------------------------:|--------------------------:|-------------------------:| | LGPS | 288.2 | 118.6 | 288.9 | 133.1 |
3. 扩散行为计算 Calculation of Diffusion Performance
3.1 基本原理和计算过程 Basic Principles and Calculation Procedure
(1) 均方根位移MSD:计算t时间内Li离子移动距离平方的平均 (2) 扩散系数D:求解t时间内的MSD在每个维度上的平均 (3) 离子电导率:根据Nernst-Einstein Equation计算 (4) 扩散系数和温度之间满足阿伦尼乌斯关系: (5) 因此,离子电导率和温度之间满足:
在研究LXPS体系的扩散系数时,我们将通用力场与DPA-2和AIMD的结果进行了比较。结果表明,使用DPA势函数计算得到的扩散系数与AIMD的结果具有很好的一致性。在室温条件下,实验测得的锂离子扩散系数通常在的数量级。相比之下,通用力场往往会高估锂离子的扩散系数,误差达到2到3个数量级,因此无法实现准确的预测。
(1) Root mean square displacement MSD: calculate the average of the square of the distance moved by Li ions in time t (2) Diffusion coefficient D: solve the average of MSD in each dimension in time t (3) Ionic conductivity : calculated according to Nernst-Einstein Equation (4) The diffusion coefficient and temperature satisfy the Arrhenius relationship: (5) Therefore, the relationship between ionic conductivity and temperature satisfies:
When studying the diffusion coefficient of the LXPS system, we compared the results of the universal force field with those of DPA-2 and AIMD. The results show that the diffusion coefficient calculated using the DPA potential function is in good agreement with the results of AIMD. At room temperature, the experimentally measured diffusion coefficient of lithium ions is usually on the order of . In contrast, the universal force field tends to overestimate the diffusion coefficient of lithium ions by 2 to 3 orders of magnitude, making it impossible to achieve accurate predictions.
3.2 分子动力学模拟 MD Simulation
如前所述,正因为M3GNET和CHGNET的特点都是内部嵌入了ase的部分模块,所以主体的代码结构非常相似,只不过调用模型的具体方式有所不同。我们已经在能量、受力精度评测的部分展示完整的计算代码,为了节省篇幅,下面分子动力学部分将只展示CHGNET在NVT系综下,控温于500K的计算.
As mentioned above, because both M3GNET and CHGNET have some modules of ase embedded inside, the main code structure is very similar, but the specific way of calling the model is different. We have shown the complete calculation code in the energy and force accuracy evaluation part. In order to save space, the following molecular dynamics part will only show the calculation of CHGNET under NVT ensemble and temperature control at 500K.
. ├── LXPS-structure │ └── LGPS │ └── CONTCAR ├── chgnet │ └── LGPS_order │ └── 500k ├── chgnet_merged.json ├── m3gnet │ └── LGPS_order │ └── 500k ├── m3gnet_merged.json └── summary.txt 8 directories, 4 files
0
CHGNET
../LXPS-structure中存储了Li-Ge-P-S的POSCAR格式文件;
下面的代码是将../LXPS-structure中的结构文件复制进文件夹中.
In ../LXPS-structure stores the POSCAR format file of Li-Ge-P-S;
The following code is to copy the structure file in ../LXPS-structure into the folder.
批量复制、修改输入文件
The following code is to batch copy and modify the input file.
下面将POSCAR转成带有氧化数信息的cif文件。
Next, convert POSCAR into a cif file with oxidation number information.
下面是lbg的模板json文件。
The following is the template json of lbg submission.
下面是CHGNET的分子动力学参数模板脚本.
Below is the molecular dynamics parameter template script for CHGNET.
批量提交任务
M3GNET
用M3GNET的计算流程与CHGNET十分相似,只不过调用模型的代码有所区别,因此下面只展示m3gnet_md.py
的代码。
The calculation procedure of M3GNET is very similar to that of CHGNET, except that the code for calling the model is different, so only the code of m3gnet_md.py
is shown below.
3.2 离子扩散系数 Ionic Diffusion Coefficient
['LGPS_order/500k/chgnet_500_nvt.traj']
S: 1.0660e-12 m²/s P: 7.4648e-13 m²/s Li: 2.0311e-09 m²/s Ge: 1.6669e-12 m²/s 2.0311e-09 | disorder | temperature | Li_diffusion_coefficient | |:-----------|--------------:|---------------------------:| | LGPS_order | 500 | 2.0311e-09 |
'\n# Save the nested dictionary to a JSON file with the desired structure\nwith open("../chgnet_merged.json", "w") as outfile:\n json.dump(results, outfile, indent=2)\n\n# Inform the user that the data has been saved\nprint("Data has been successfully saved to ../chgnet_merged.json.")'
等回传完成后,用以下代码计算扩散系数.
After the return is completed, use the following code to calculate the diffusion coefficient.
3.3 离子电导率 Ionic Conductivity
CHGNET data results: LGPS, 500, 1608.8 ± 0.0 mS/cm M3GNET data results: LGPS, 500, 3479.9 ± 0.0 mS/cm
4. 总结 Summarization
基于硫族化合物固态电解质的基本物质组成,我们对比了DPA-2预训练模型和通用力场(CHGNET、M3GNET)在能量受力的推理和扩散性质预测的表现,我们发现DPA模型精度更高, 能更准确预测动力学性质(扩散系数和离子电导率),有助于辅助实验设计/改性。
Based on the basic material composition of chalcogenide solid electrolytes, we compared the performance of the DPA-2 pre-trained model and the general force field (CHGNET, M3GNET) in energy force reasoning and diffusion property prediction. We found that the DPA model has higher accuracy and can more accurately predict kinetic properties (diffusion coefficient and ionic conductivity), which helps to assist experimental design/modification.
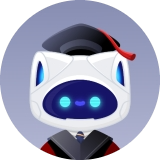
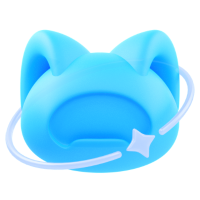
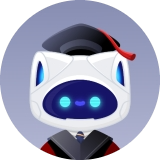
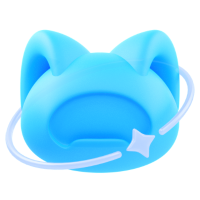
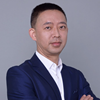
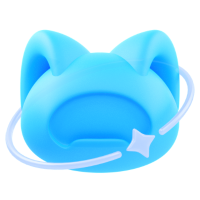
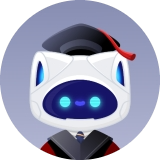
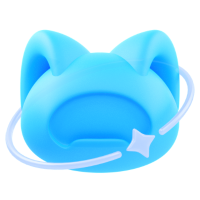