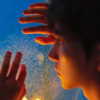
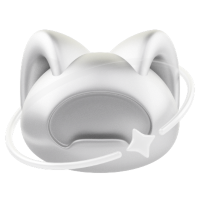

©️ Copyright 2023 @ Authors
Authors: Xinzijian Liu📨 Chengqian Zhang📨
Date: 2023-12-20
License: Attribution-NonCommercial-ShareAlike 4.0 International
Quick start: You can click on the blue button at the top of the page Connect , select `registry.dp.tech/dptech/deepmd-kit:3.0.0b3-cuda12.1` image and `c12_m92_1 * NVIDIA V100` machine, and at the same time mount the `dpa2_data(v6)` and `dpa2-finetune-example-water(v2)` dataset, and wait for a few moments to run.
Introduction
Paper link: https://arxiv.org/abs/2312.15492
The codes, datasets and input scripts are all available on zenodo (https://doi.org/10.5281/zenodo.10428497)
This notebook is updated on 9.14 based on beta3
code version.
The rapid development of artificial intelligence (AI) is driving significant changes in the field of atomic modeling, simulation, and design. AI-based potential energy models have been successfully used to perform large-scale and long-time simulations with the accuracy of ab initio electronic structure methods. However, the model generation process still hinders applications at scale. We envision that the next stage would be a model-centric ecosystem, in which a large atomic model (LAM), pre-trained with as many atomic datasets as possible and can be efficiently fine-tuned and distilled to downstream tasks, would serve the new infrastructure of the field of molecular modeling. We propose DPA-2, a novel architecture for a LAM, and develop a comprehensive pipeline for model fine-tuning, distillation, and application, associated with automatic workflows. We show that DPA-2 can accurately represent a diverse range of chemical systems and materials, enabling high-quality simulations and predictions with significantly reduced efforts compared to traditional methods. Our approach paves the way for a universal large atomic model that can be widely applied in molecular and material simulation research, opening new opportunities for scientific discoveries and industrial applications.
In order to run this notebook successfully, let's do some preparatory work first.
. └── src ├── data │ ├── FerroEle_train │ ├── FerroEle_valid │ ├── H2O-PD_train │ ├── H2O-PD_valid │ ├── SemiCond_train │ └── SemiCond_valid ├── md │ └── water_192 ├── model │ ├── H2O-PD.pt │ └── OpenLAM_2.2.0_27heads_beta3.pt
└── train ├── finetune ├── multitask └── singletask 15 directories, 2 files
- data: This directory contains three datasets FerroEle, H2O-PD and SemiCond. FerroEle is a small subset of the dataset FerroEle_DPA_v1_0. H2O_H2O-PD is a small subset of the dataset H2O-PD_DPA_v1_0. SemiCond is a small subset of the dataset SemiCond_DPA_v1_0.If you want to get the full dataset, you can go to website AIS Square to download these datasets.
- model: This directory contains one singletask model H2O-PD.pt and one multitask model OpenLAM_2.2.0_27heads_beta3.pt. The multitask model OpenLAM_2.2.0_27heads_beta3.pt is trained on 27 different datasets.
- train: This directory contains three modes of training, which are singletask training, multitask training, and finetuning based on pretrained model. In a moment, we're going to demonstrate how to do these trainings in the three folders.
- md: In this directory, we will demonstrate how to perform molecular dynamics simulation using DPA-2 model.
Model Loading
One can download someone else's trained singletask model or multitask model from web AIS Square
/root/src/data /opt/deepmd-kit-3.0.0b3/lib/python3.10/site-packages/IPython/core/magics/osm.py:417: UserWarning: This is now an optional IPython functionality, setting dhist requires you to install the `pickleshare` library. self.shell.db['dhist'] = compress_dhist(dhist)[-100:]
command line interface
The model can be used in many ways. The most straightforward test can be performed using dp test
Firstly we test the valid dataset of H2O_H2O-PD using the singletask model H2O-PD.pt.
where -m
gives the model checkpoint to import, -s
the path to the tested system and -n
the number of tested frames. Several other command line options can be passed to dp test, which can be checked with
usage: dp test [-h] [-v {DEBUG,3,INFO,2,WARNING,1,ERROR,0}] [-l LOG_PATH] [-m MODEL] [-s SYSTEM | -f DATAFILE] [-S SET_PREFIX] [-n NUMB_TEST] [-r RAND_SEED] [--shuffle-test] [-d DETAIL_FILE] [-a] [--head HEAD] options: -h, --help show this help message and exit -v {DEBUG,3,INFO,2,WARNING,1,ERROR,0}, --log-level {DEBUG,3,INFO,2,WARNING,1,ERROR,0} set verbosity level by string or number, 0=ERROR, 1=WARNING, 2=INFO and 3=DEBUG (default: INFO) -l LOG_PATH, --log-path LOG_PATH set log file to log messages to disk, if not specified, the logs will only be output to console (default: None) -m MODEL, --model MODEL Frozen model file (prefix) to import. TensorFlow backend: suffix is .pb; PyTorch backend: suffix is .pth. (default: frozen_model) -s SYSTEM, --system SYSTEM The system dir. Recursively detect systems in this directory (default: .) -f DATAFILE, --datafile DATAFILE The path to the datafile, each line of which is a path to one data system. (default: None) -S SET_PREFIX, --set-prefix SET_PREFIX [DEPRECATED] Deprecated argument. (default: None) -n NUMB_TEST, --numb-test NUMB_TEST The number of data for test. 0 means all data. (default: 0) -r RAND_SEED, --rand-seed RAND_SEED The random seed (default: None) --shuffle-test Shuffle test data (default: False) -d DETAIL_FILE, --detail-file DETAIL_FILE The prefix to files where details of energy, force and virial accuracy/accuracy per atom will be written (default: None) -a, --atomic Test the accuracy of atomic label, i.e. energy / tensor (dipole, polar) (default: False) --head HEAD (Supported backend: PyTorch) Task head to test if in multi-task mode. (default: None) examples: dp test -m graph.pb -s /path/to/system -n 30
We then use the multitask model OpenLAM_2.2.0_27heads_beta3.pt to test the H2O_H2O-PD valid dataset, and it's worth noting that when we use the multitask model to do the testing we need to specify the task head.
- --head: Task head to test if in multi-task mode.
python interface
One can use python interface of DPA-2 to obtain the energy, force, virial of specific structures.
To get the best performance, it is recommended to adjust the number of threads by setting the environment variables OMP_NUM_THREADS, DP_INTRA_OP_PARALLELISM_THREADS, and DP_INTER_OP_PARALLELISM_THREADS. See https://deepmd.rtfd.io/parallelism/ for more information. Predict energy: -490.54547 Label energy: -490.48730
Model Training
We're going to demonstrate how to perform singletask training, multitask training and finetuning based on pretrained model.
singletask
/root/src/train/singletask
Here we use a small subset of the dataset H2O-PD_DPA_v1_0 to train a singletask model. The file input.json is the input script.
The parameters in input.json are the same as in the previous version of DeePMD-kit except for the descriptor part. For more details, you can go to our paper.
The training can be invoked by
During the training, the error of the model is tested every disp_freq training steps. The training error and validation error are printed correspondingly in the file disp_file (default is lcurve.out). The batch size can be set in the input script by the key batch_size in the corresponding sections for the training and validation data set. An example of the output:
The file contains 8 columns, from left to right, which are the training step, the validation loss, training loss, root mean square (RMS) validation error of energy, RMS training error of energy, RMS validation error of force, RMS training error of force and the learning rate. The RMS error (RMSE) of the energy is normalized by the number of atoms in the system. One can visualize this file with a simple Python script:
multitask
/root/src/train/multitask
Training on multiple data sets (each data set contains several data systems) can be performed in multi-task mode, with one common descriptor and multiple specific fitting nets for each data set. One needs to switch some parameters in training input script to perform multi-task mode including:
model –> model_dict, each key of which can be one individual fitting net.
training_data, validation_data –> data_dict, each key of which can be one individual data set contains several data systems for corresponding fitting net, the keys must be consistent with those in model_dict.
loss –> loss_dict, each key of which can be one individual loss setting for corresponding fitting net, the keys must be consistent with those in model_dict.
model_prob, each key of which can be a non-negative integer or float, deciding the chosen probability for corresponding fitting net in training.
Here we use three different datasets(a small subset of the dataset FerroEle_DPA_v1_0, a small subset of the dataset H2O-PD_DPA_v1_0, and a small subset of the dataset SemiCond_DPA_v1_0) to train a multitask model with three task heads.
The training procedure will automatically choose single-task or multi-task mode, based on the above parameters. The training can be invoked by
finetune
/root/src/train/finetune
Pretraining-and-finetuning is a widely used approach in other fields such as Computer Vision (CV) or Natural Language Processing (NLP) to vastly reduce the training cost, while it’s not trivial in potential models. Compositions and configurations of data samples or even computational parameters in upstream software (such as VASP) may be different between the pretrained and target datasets, leading to energy shifts or other diversities of training data.
The multitask training mode can overcome above difficulties. Our DPA-2 model can hopefully learn the common knowledge in the pretrained dataset and thus reduce the computational cost in downstream training tasks.
Here we have a pretrained multitask model multitask_model.pt on a large dataset (eighteen different datasets), a finetuning strategy can be performed by simply running:
The finetune procedure will inherit the neural network parameters of descriptor in pretrained multitask model. The fitting net can either reinit or inherit the fitting net from any branch of the pre-trained model depending on the argument -m.
- -m (--model-branch): Model branch chosen for fine-tuning if multi-task. If not specified, it will re-init the fitting net.
Whether singletask mode, multitask mode or finetune mode, the training set contains H2O-PD, so we can compare the validation error on dataset H2O-PD directly using a python script
dp freeze
The .pth
extension file for molecular dynamics simulations can be obtained by dp freeze
.
To get the best performance, it is recommended to adjust the number of threads by setting the environment variables OMP_NUM_THREADS, DP_INTRA_OP_PARALLELISM_THREADS, and DP_INTER_OP_PARALLELISM_THREADS. See https://deepmd.rtfd.io/parallelism/ for more information. [2024-09-14 11:42:54,054] DEEPMD INFO DeePMD version: 3.0.0b3
Molecular Dynamics
The model can drive molecular dynamics in LAMMPS.
/root/src/md/water_192
data.dpa2* dpa2_in.lammps* dpa2_model.pth*
Here data.dpa2 gives the initial configuration of water MD simulation, and the file dpa2_in.lammps is the LAMMPS input script. One may check dpa2_in.lammps and finds that it is a rather standard LAMMPS input file for a MD simulation, with only two exception lines:
'\n# See https://deepmd.rtfd.io/lammps/ for usage\npair_style\tdeepmd dpa2_model.pth\n# If atom names (O H in this example) are not set in the pair_coeff command, the type_map defined by the training parameter will be used by default.\npair_coeff * *\tO H\n'
where the pair style deepmd is invoked and the model file dpa2_model.pth is provided, which means the atomic interaction will be computed by the DPA-2 model that is stored in the file dpa2_model.pth.
In an environment with a compatible version of LAMMPS, the deep potential molecular dynamics can be performed via
Distillation
Distillation can significantly improve the efficiency of finetuned models in MD simulations for production. Distillation requires DP-Gen2. For detail, you can refer to notebook https://bohrium.dp.tech/notebooks/62585747598 or https://bohrium.dp.tech/notebooks/76262686918
DP-Gen based on a DPA-2 pretrained model
Finetuning based on DPA-2 pretrained model can reduce the amount of data required for training. Running DP-Gen with a DPA-2 pretrained model can also save first-principles labelling. DP-Gen with DPA-2 requires DP-Gen2. For detail, you can refer to notebook https://bohrium.dp.tech/notebooks/62585747598 or https://bohrium.dp.tech/notebooks/76262686918
Tips
Users are welcome to explore the DP Combo web server , which helps users automate operations such as model training and model distillation. Related notebook: DP Combo教程, 借助DP Combo一键丝滑生成半导体势函数 and 固态电解质实战 | DP Combo@APP体验
Current DPA-2 model does not yet support features such as zbl, which we will implement in the near future. If you want to use these features, you can use the previous version of DeePMD-kit(github). Related notebook: DeePMD 使用教程、科研案例、问题收集合集
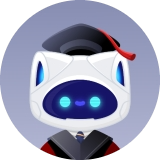
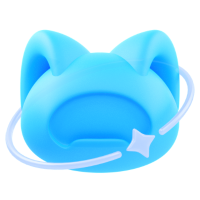
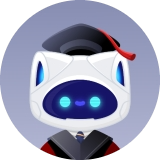
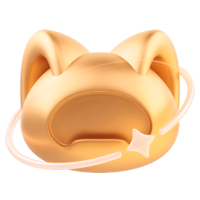
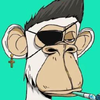
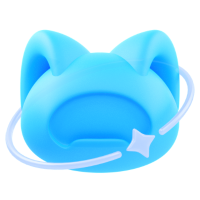

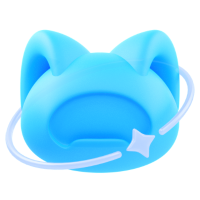
dfzshiwo@163.com
2043899742@qq.com