
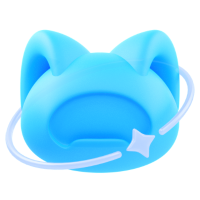
多分辨率反卷积模型DestVI
DestVI论文:https://www.nature.com/articles/s41587-022-01272-8
本教程搬运和修改自:https://docs.scvi-tools.org/en/stable/tutorials/notebooks/spatial/DestVI_tutorial.html
在本教程中,我们将介绍使用单细胞RNA测序数据应用DestVI对10x Visium空间转录组学谱进行反卷积的步骤。
📖 上手指南
本文档可在 Bohrium Notebook 上直接运行。如果是在案例广场,你可以直接运行;如果是保存到自己的空间中,你可以点击界面上方按钮 开始连接,选择 scvi-tools:v1.0 镜像及 c4_m30_1 * NVIDIA P100 节点配置,稍等片刻即可运行。
背景介绍
目前,空间转录组学技术受到限制,因为大部分的空转分辨率仅限于远远超过单个细胞大小的微环境(spot)。尽管有几种流程建议与单细胞RNA测序(scRNA-seq)联合分析以缓解此问题,但它们仅限于每个spot内细胞类型比例离散的视图。这种限制在常见情况下变得至关重要,即使在一种细胞类型中,也存在连续的细胞状态。作者提出了 使用变分推断 (DestVI) 对空间转录组学概况进行反卷积,这是一种用于空间转录组学多分辨率分析的概率方法,可明确模拟细胞类型内的连续变化。该方法于2022年发表在Nature Biotechnology上。
DestVI使用两种不同的潜在变量模型(LVMs)来推断ctp(cell type proportion)和细胞类型特定的连续子状态。DestVI以一对数据集作为输入:来自同一组织的查询ST数据和参考scRNA-seq数据,用细胞类型标签注释。输出包括每个点的ctp和每个点中每个细胞类型的细胞状态的连续估计。为了对参考scRNA-seq数据进行建模,假设DestVI的第一个LVM(单细胞隐变量模型),对于每个基因g和细胞n,观察到的转录本的数量遵循一个负二项分布。为了对ST数据进行建模,假设DestVI的第二个LVM(空间转录组隐变量模型stLVM),对于每个基因g和每个点s,观察到的转录本数量也遵循负二项分布。
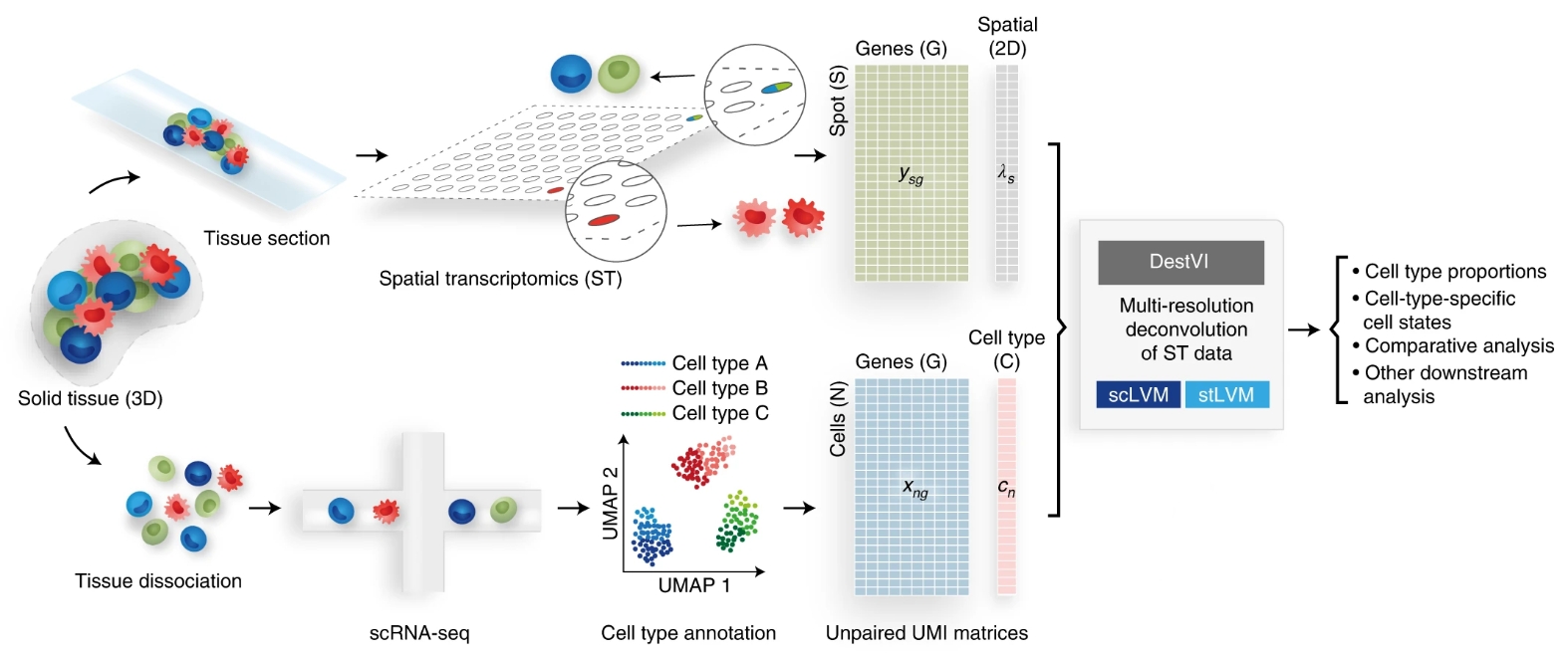
具体到这两个模型而言(如下图),scRNA-seq数据中的RNA counts和细胞类型信息通过一个编码器神经网络联合转换成的近似后验参数,是细胞类型特异性细胞状态的低维表示。接下来,一个解码器神经网络将从的近似后验中采的样以及细胞类型信息,映射到每个基因计数分布的参数。上标符号表示向量的第个条目。而ST数据中的RNA counts通过一个编码器神经网络转换成细胞类型特异性嵌入的参数。自由参数编码了在点中细胞类型的丰度,并可以归一化为CTP 。scLVM模型的解码器将细胞类型特异性嵌入映射到细胞类型特异性基因表达的估计值。这些值在所有细胞类型上加权求和,由丰度参数加权,以获得近似表示点的基因表达的参数。训练后,解码器可用于在所有点上执行细胞类型特异性的基因表达插补。
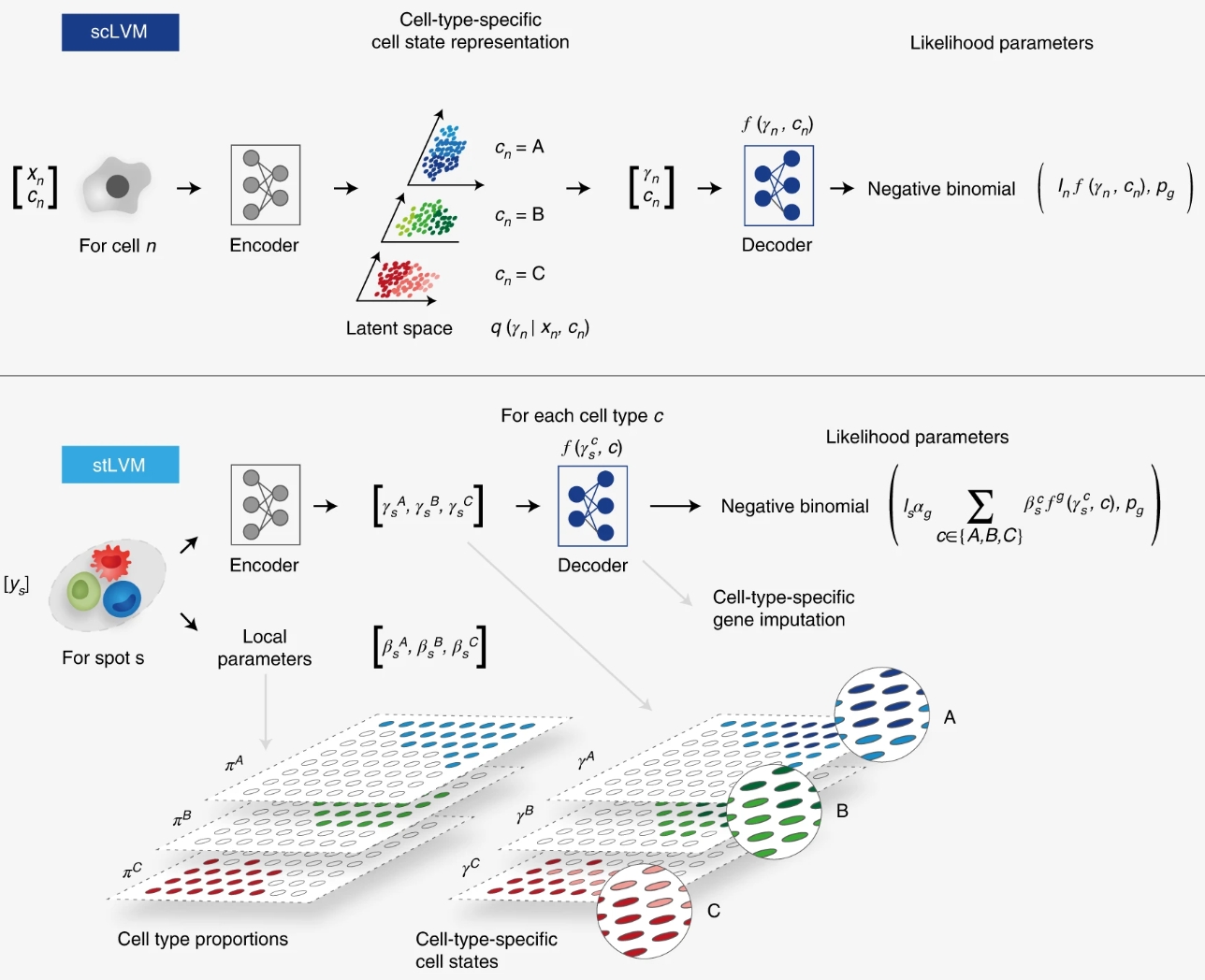
本教程将会涵盖以下内容
- 加载数据
- 训练单细胞模型 (scLVM) 以了解 scRNA-seq 数据上基因表达的基础
- 训练空间模型 (stLVM) 以执行反卷积
- 可视化学习到的细胞类型比例
- 运行自动化pipeline
- 深入研究细胞类型内信息
- 运行细胞类型特定的差异表达分析
前置准备
加载必要的库
Seed set to 0 Last run with scvi-tools version: 1.1.6
设置全局参数
你可以更改下面的
save_dir
来决定本notebook产生的结果文件的存储路径。
让我们使用一项小鼠淋巴结比较研究中的数据,比较野生型与注射分枝杆菌刺激的类型。数据下载大约需要30min,这里我们已经提前准备好了:一个10x Visium数据集以及来自同一组织的匹配scRNA-seq数据集。
加载和准备数据
首先加载单细胞数据。原文作者从可重复性存储库中提供了预处理的数据,包含的是原始计数(DestVI始终将原始计数作为输入)。
我们根据主要的免疫细胞类型对单细胞数据进行聚类。DestVI可以解析超越离散簇的数据,但需要与已有的聚类水平配合使用。聚类时要记住的一个经验法则是,DestVI假设每个点中每种细胞类型只有一个状态。例如,根据假设,静息单核细胞和发炎单核细胞不能共存于一个点。使用时可以对数据进行聚类,以使此建模假设尽可能准确。
现在,让我们加载空间数据并选择一个共同的基因子集。注意到,拥有一个共同的基因集是该方法的先决条件。
建立scLVM模型
为了了解细胞状态特定的基因表达模式,我们将单细胞潜在变量模型(scLVM)与来自同一组织的单细胞RNA测序数据相匹配。
第一步,我们将数据输入细胞类型条件VAE,传递包含原始计数和标签键的层。训练此模型时并不根据细胞类型丰度重加权损失。在有GPU的情况下,训练大约需要5分钟。
Anndata setup with scvi-tools version 1.1.6. ,
,
Setup via `CondSCVI.setup_anndata` with arguments: ,
{'labels_key': 'broad_cell_types', 'layer': 'counts'} ,
,
Summary Statistics ,┏━━━━━━━━━━━━━━━━━━┳━━━━━━━┓ ,┃ Summary Stat Key ┃ Value ┃ ,┡━━━━━━━━━━━━━━━━━━╇━━━━━━━┩ ,│ n_cells │ 14989 │ ,│ n_labels │ 12 │ ,│ n_vars │ 1888 │ ,└──────────────────┴───────┘ ,
Data Registry ,┏━━━━━━━━━━━━━━┳━━━━━━━━━━━━━━━━━━━━━━━━━━━┓ ,┃ Registry Key ┃ scvi-tools Location ┃ ,┡━━━━━━━━━━━━━━╇━━━━━━━━━━━━━━━━━━━━━━━━━━━┩ ,│ X │ adata.layers['counts'] │ ,│ labels │ adata.obs['_scvi_labels'] │ ,└──────────────┴───────────────────────────┘ ,
labels State Registry ,┏━━━━━━━━━━━━━━━━━━━━━━━━━━━━━━━┳━━━━━━━━━━━━━━━┳━━━━━━━━━━━━━━━━━━━━━┓ ,┃ Source Location ┃ Categories ┃ scvi-tools Encoding ┃ ,┡━━━━━━━━━━━━━━━━━━━━━━━━━━━━━━━╇━━━━━━━━━━━━━━━╇━━━━━━━━━━━━━━━━━━━━━┩ ,│ adata.obs['broad_cell_types'] │ B cells │ 0 │ ,│ │ CD4 T cells │ 1 │ ,│ │ CD8 T cells │ 2 │ ,│ │ GD T cells │ 3 │ ,│ │ Macrophages │ 4 │ ,│ │ Migratory DCs │ 5 │ ,│ │ Monocytes │ 6 │ ,│ │ NK cells │ 7 │ ,│ │ Tregs │ 8 │ ,│ │ cDC1s │ 9 │ ,│ │ cDC2s │ 10 │ ,│ │ pDCs │ 11 │ ,└───────────────────────────────┴───────────────┴─────────────────────┘ ,
GPU available: True (cuda), used: True TPU available: False, using: 0 TPU cores IPU available: False, using: 0 IPUs HPU available: False, using: 0 HPUs LOCAL_RANK: 0 - CUDA_VISIBLE_DEVICES: [0] /opt/mamba/lib/python3.10/site-packages/lightning/pytorch/trainer/connectors/data_connector.py:441: The 'train_dataloader' does not have many workers which may be a bottleneck. Consider increasing the value of the `num_workers` argument` to `num_workers=7` in the `DataLoader` to improve performance. Epoch 300/300: 100%|██████████| 300/300 [05:42<00:00, 1.14s/it, v_num=1, train_loss_step=697, train_loss_epoch=800] `Trainer.fit` stopped: `max_epochs=300` reached. Epoch 300/300: 100%|██████████| 300/300 [05:42<00:00, 1.14s/it, v_num=1, train_loss_step=697, train_loss_epoch=800]
注意到,模型收敛速度很快,大幅减少epoch数量会导致性能下降,推荐使用max_epochs>200。
使用stLVM进行反卷积
第二步,我们训练反卷积模型:空间转录组学潜在变量模型 (stLVM)。
我们使用包含原始计数的 st_adata
中的 counts
层设置 DestVI 模型。然后,我们传递经过训练的 CondSCVI
模型,并使用 DestVI.from_rna_model
基于 st_adata
和 sc_model
生成新模型。
解码器网络架构将从 sc_model
生成。启动两个神经网络,用于细胞类型比例和伽马值分配。在有GPU情况下,训练大约需要5分钟。
DestVI.from_rna_model
的潜在适应性包括:
- 增加
vamp_prior_p
会导致伽马值的变化不那么渐进。 - 值更加离散。增加
l1_sparsity
将导致细胞类型比例的结果更稀疏。 - 虽然建议对两种assay使用类似的测序技术,但如果不同请考虑更改
beta_weighting_prior
。
Anndata setup with scvi-tools version 1.1.6. ,
,
Setup via `DestVI.setup_anndata` with arguments: ,
{'layer': 'counts'} ,
,
Summary Statistics ,┏━━━━━━━━━━━━━━━━━━┳━━━━━━━┓ ,┃ Summary Stat Key ┃ Value ┃ ,┡━━━━━━━━━━━━━━━━━━╇━━━━━━━┩ ,│ n_cells │ 1092 │ ,│ n_vars │ 1888 │ ,└──────────────────┴───────┘ ,
Data Registry ,┏━━━━━━━━━━━━━━┳━━━━━━━━━━━━━━━━━━━━━━━━┓ ,┃ Registry Key ┃ scvi-tools Location ┃ ,┡━━━━━━━━━━━━━━╇━━━━━━━━━━━━━━━━━━━━━━━━┩ ,│ X │ adata.layers['counts'] │ ,│ ind_x │ adata.obs['_indices'] │ ,└──────────────┴────────────────────────┘ ,
GPU available: True (cuda), used: True TPU available: False, using: 0 TPU cores IPU available: False, using: 0 IPUs HPU available: False, using: 0 HPUs LOCAL_RANK: 0 - CUDA_VISIBLE_DEVICES: [0] /opt/mamba/lib/python3.10/site-packages/lightning/pytorch/trainer/connectors/data_connector.py:441: The 'train_dataloader' does not have many workers which may be a bottleneck. Consider increasing the value of the `num_workers` argument` to `num_workers=7` in the `DataLoader` to improve performance. /opt/mamba/lib/python3.10/site-packages/lightning/pytorch/loops/fit_loop.py:293: The number of training batches (9) is smaller than the logging interval Trainer(log_every_n_steps=10). Set a lower value for log_every_n_steps if you want to see logs for the training epoch. Epoch 1/2500: 0%| | 0/2500 [00:00<?, ?it/s]Epoch 2500/2500: 100%|██████████| 2500/2500 [05:00<00:00, 8.32it/s, v_num=1, train_loss_step=1.89e+6, train_loss_epoch=1.94e+6]`Trainer.fit` stopped: `max_epochs=2500` reached. Epoch 2500/2500: 100%|██████████| 2500/2500 [05:00<00:00, 8.31it/s, v_num=1, train_loss_step=1.89e+6, train_loss_epoch=1.94e+6]
从结果来看,建议不要将max_epochs设置为<1000。
DestVI的输出有两种分辨率。在更粗略的分辨率下,DestVI返回每个点的细胞类型比例。在更精细的分辨率下,DestVI可以估算每个点中特定细胞类型的基因表达。
推断细胞类型比例
我们提取计算出的细胞类型比例并将其显示在空间嵌入中。这些值是通过对 stLVM 模型中的点级参数进行归一化而直接计算得出的。
B cells | CD4 T cells | CD8 T cells | GD T cells | Macrophages | Migratory DCs | Monocytes | NK cells | Tregs | cDC1s | cDC2s | pDCs | |
---|---|---|---|---|---|---|---|---|---|---|---|---|
AAACCGGGTAGGTACC-1-0 | 0.633301 | 0.040610 | 0.013512 | 0.003582 | 0.036714 | 0.032418 | 0.011457 | 0.002981 | 0.064497 | 0.051010 | 0.093252 | 0.016668 |
AAACCTCATGAAGTTG-1-0 | 0.576852 | 0.087551 | 0.024790 | 0.004386 | 0.027783 | 0.064477 | 0.016833 | 0.002004 | 0.045020 | 0.034356 | 0.106308 | 0.009639 |
AAAGACTGGGCGCTTT-1-0 | 0.551651 | 0.077436 | 0.025004 | 0.005209 | 0.027974 | 0.084043 | 0.006686 | 0.002224 | 0.071786 | 0.050665 | 0.095821 | 0.001499 |
AAAGGGCAGCTTGAAT-1-0 | 0.088732 | 0.203654 | 0.287289 | 0.017000 | 0.029057 | 0.116767 | 0.032214 | 0.003603 | 0.098834 | 0.070529 | 0.047927 | 0.004393 |
AAAGTCGACCCTCAGT-1-0 | 0.794044 | 0.030890 | 0.001612 | 0.002178 | 0.022524 | 0.031548 | 0.009031 | 0.002439 | 0.019728 | 0.047512 | 0.035980 | 0.002516 |
由于当细胞类型不存在于某个点时,推断细胞类型特异性基因表达很容易出错,并且由于 stLVM 估计的细胞类型比例值并不稀疏,因此这里使用一种自动阈值化方法。对于后续分析,建议检查这些阈值并针对每种细胞类型进行调整。这一功能不是直接在 scvi-tools 中提供的,而是在程序包“destvi_utils”中提供的。
100%|██████████| 100/100 [00:00<00:00, 148.09it/s]
100%|██████████| 100/100 [00:00<00:00, 14356.68it/s]
100%|██████████| 100/100 [00:00<00:00, 15189.60it/s]
在细胞类型定位方面,可以观察到淋巴结中细胞类型(B 细胞/T 细胞)的强烈区域化,另外我们还观察到单核细胞的差异定位。
细胞类型内信息
DestVI 的核心是大量潜在变量(每个细胞类型在每个点都有5个)。我们将它们称为“gamma”,我们可以手动检查它们以进行下游分析
0 | 1 | 2 | 3 | 4 | |
---|---|---|---|---|---|
AAACCGGGTAGGTACC-1-0 | -0.141003 | 0.092833 | 0.433705 | 0.002089 | -0.693667 |
AAACCTCATGAAGTTG-1-0 | -1.504918 | -1.650834 | 0.403841 | 0.161406 | -0.916408 |
AAAGACTGGGCGCTTT-1-0 | -2.028534 | -1.087074 | 0.487336 | 0.100378 | -0.677558 |
AAAGGGCAGCTTGAAT-1-0 | -0.362801 | 0.184882 | 0.286093 | -0.187601 | -0.046159 |
AAAGTCGACCCTCAGT-1-0 | 0.376768 | -0.131472 | 0.644372 | -0.728894 | -0.233547 |
考虑到这些值可能难以手动检查,原文中介绍了几种优先的研究不同细胞类型的方法(基于空间加权PCA和Hotspot)。下面介绍使用空间加权 PCA 的自动化流程的结果。
更准确地说,为了从头检测空间模式,我们研究伽马空间并使用空间信息 PCA 来查找此伽马空间中的空间变异轴。我们使用 EnrichR 对这些基因进行功能注释。具体来说,我们进行了单核细胞和 B 细胞中 IFN 基因的富集
函数 explore_gamma_space
针对每种细胞类型分别按如下方式运行:
- 选择所有比例超过幅度阈值的点,
- 计算点特定的细胞类型特定的伽马embedding,
- 计算这些伽马值的前两个主向量,并按空间坐标加权,
- 将所有嵌入(考虑的点和单细胞轮廓)投影到这个 2D 空间上,
- 使用
cmap2d
包通过其 2d 坐标将每个点(或细胞)映射到特定颜色 - 绘制(A)空间坐标中每个点的颜色(B)sPC 空间中每个点的颜色(C)sPC 空间中每个单细胞的颜色
- 计算每个方向富集的基因并使用
EnrichR
分组到通路中
/opt/mamba/lib/python3.10/site-packages/cmap2d/util.py:32: FutureWarning: `rcond` parameter will change to the default of machine precision times ``max(M, N)`` where M and N are the input matrix dimensions. To use the future default and silence this warning we advise to pass `rcond=None`, to keep using the old, explicitly pass `rcond=-1`. bary_coords = la.lstsq(self._a,b)[0]
Genes associated with SpatialPC1
,
/opt/mamba/lib/python3.10/site-packages/gseapy/enrichr.py:387: FutureWarning: The frame.append method is deprecated and will be removed from pandas in a future version. Use pandas.concat instead. self.results = self.results.append(res, ignore_index=True)
, ,
Positively
,
--------------------------------------------------------------------------------------- ,
Ifit3, Stat1, Slfn5, Rtp4, Zbp1, Irf7, Ifi47, Usp18, Ifit3b, Trim30a, Ifit1, Oasl2, Ifit2, Parp14, Isg15, Rsad2, ,Igtp, Serpina3g, Gbp4, Rnf213, Oas3, Bst2, Gbp7, Phf11b, Ifi27l2a, Isg20, Jun, Pkib, Hspa1b, Eif2ak2, Malat1, ,Ms4a4c, Herc6, Ifitm3, Irf1, Lgals3bp, Cmpk2, Socs1, Ly6a, Serpina3f, Trafd1, Hsp90aa1, Sdc3, Psmb9, Gbp2, Ms4a4b, ,Cybb, Phf11a, B2m, Gbp5 ,
--------------------------------------------------------------------------------------- ,
Interferon signaling*, Interferon alpha/beta signaling*, Immune system signaling by interferons, interleukins, ,prolactin, and growth hormones*, Immune system*, Interferon-gamma signaling pathway*, Type II interferon signaling ,(interferon-gamma)*, Antiviral mechanism by interferon-stimulated genes*, Toll-like receptor signaling pathway ,regulation*, Interferon alpha signaling regulation*, Prolactin activation of MAPK signaling* ,
/opt/mamba/lib/python3.10/site-packages/gseapy/enrichr.py:387: FutureWarning: The frame.append method is deprecated and will be removed from pandas in a future version. Use pandas.concat instead. self.results = self.results.append(res, ignore_index=True)
, ,
Negatively
,
--------------------------------------------------------------------------------------- ,
Rpsa, Rpl41, Rplp0, Gm26532, Rps2, Tmsb4x, Rplp1, Nr4a1, Hs3st1, Rps12, Junb, Actg1, Cd83, S100a11, Tubb4b, Crip1, ,Tuba1a, Npm1, Dennd4a, Ftl1, Hnrnpa1, Chchd10, Kdm6b, Fth1, Hmgb1, Vps37b, Sec61b, Rps27l, Bcl2a1b, Ybx1, Bri3, ,Plaur, Gadd45a, Ppia, Nfkbid, Cebpb, Bambi, H2afv, Tuba1c, Fos, Slc25a4, Impdh2, Ube2s, Gapdh, Gpx1, BC028528, ,H2afz, Calm1, Gpr183, Slc25a5 ,
--------------------------------------------------------------------------------------- ,
Disease*, Protein metabolism*, Translation*, T cell receptor regulation of apoptosis*, Cytoplasmic ribosomal ,proteins*, Influenza viral RNA transcription and replication*, Influenza infection*, Gene expression*, ,Post-chaperonin tubulin folding pathway*, Pathogenic Escherichia coli infection* ,
, , , ,
Genes associated with SpatialPC2
,
/opt/mamba/lib/python3.10/site-packages/gseapy/enrichr.py:387: FutureWarning: The frame.append method is deprecated and will be removed from pandas in a future version. Use pandas.concat instead. self.results = self.results.append(res, ignore_index=True)
, ,
Positively
,
--------------------------------------------------------------------------------------- ,
Rplp0, Rpsa, Rps2, Rpl41, Rplp1, Rps12, Nme2, Npm1, Ppia, Gapdh, Hspe1, Plac8, Hsp90ab1, Mif, Hnrnpa1, Ybx1, Ifi47, ,Impdh2, C1qbp, Psmb9, Pgk1, Ppa1, Nme1, Tubb5, Psme2, Atp5g1, Actg1, Eif5a, Mettl1, Hspa8, Ftl1, Cdk4, ,B930036N10Rik, Cct8, Pkm, Nhp2, Phgdh, Aprt, Pomp, Ranbp1, Snrpd1, Fbl, Tkt, Psma7, Tuba1b, Apex1, Ran, Dbi, Stat1, ,Prdx1 ,
--------------------------------------------------------------------------------------- ,
T cell receptor regulation of apoptosis*, Disease*, Gene expression*, HIV factor interactions with host*, Influenza ,infection*, Protein metabolism*, Cytoplasmic ribosomal proteins*, HIV infection*, Destabilization of mRNA by AUF1 ,(hnRNP D0)*, Influenza viral RNA transcription and replication* ,
/opt/mamba/lib/python3.10/site-packages/gseapy/enrichr.py:387: FutureWarning: The frame.append method is deprecated and will be removed from pandas in a future version. Use pandas.concat instead. self.results = self.results.append(res, ignore_index=True)
, ,
Negatively
,
--------------------------------------------------------------------------------------- ,
Malat1, Dennd4a, Junb, Kdm6b, Fos, Gm26532, Gm42418, Nr4a1, Fosb, Pim1, Dusp1, Myadm, Klf4, Vim, Dusp5, Birc3, ,Vps37b, Ahnak, Flna, Nfkbid, Atp2b1, Osbpl3, Cd69, Lyst, Tnfaip3, Rel, Bcl11a, Kcnq1ot1, Gpr183, Vpreb3, Gata3, ,Crmp1, Ccr2, Zbtb20, Il18r1, Egr1, S100a4, Neat1, Fut7, H2-Ab1, Hmmr, Ttc39c, Plk1, Cebpb, Anln, Ddhd1, Icos, Smtn, ,Cd83, Atp2b4 ,
--------------------------------------------------------------------------------------- ,
T cell receptor regulation of apoptosis*, BDNF signaling pathway*, Interleukin-2 signaling pathway*, Interleukin-5 ,regulation of apoptosis*, TSH regulation of gene expression*, AP-1 transcription factor network*, C-Myb ,transcription factor network*, Regulation of NFAT transcription factors*, ATF2 transcription factor network*, ,TNF-alpha effects on cytokine activity, cell motility, and apoptosis* ,
, , , ,
/opt/mamba/lib/python3.10/site-packages/cmap2d/util.py:32: FutureWarning: `rcond` parameter will change to the default of machine precision times ``max(M, N)`` where M and N are the input matrix dimensions. To use the future default and silence this warning we advise to pass `rcond=None`, to keep using the old, explicitly pass `rcond=-1`. bary_coords = la.lstsq(self._a,b)[0]
Genes associated with SpatialPC1
,
/opt/mamba/lib/python3.10/site-packages/gseapy/enrichr.py:387: FutureWarning: The frame.append method is deprecated and will be removed from pandas in a future version. Use pandas.concat instead. self.results = self.results.append(res, ignore_index=True)
, ,
Positively
,
--------------------------------------------------------------------------------------- ,
Ccr9, Malat1, Rgcc, Ramp1, Stat1, Itgae, Iigp1, Ifi27l2a, Slfn5, Zbp1, Isg15, Igtp, Ifit1, Ifit3, Cd3g, B2m, Gbp2, ,Igfbp4, Gbp7, Isg20, Sox4, Gbp4, Gbp8, Rtp4, Actb, Pmepa1, Oasl2, Usp18, Actn2, Lztfl1, Cd226, Ifit3b, Bcl11b, ,Rsad2, Slfn1, Ifi47, Cd3e, Lef1, Parp14, Trib2, Cd274, Inpp4b, Irf7, Ddx60, Npc2, Rnf213, Gsn, Cd8b1, Tesc, Ift80 ,
--------------------------------------------------------------------------------------- ,
Interferon signaling*, Interferon alpha/beta signaling*, Immune system signaling by interferons, interleukins, ,prolactin, and growth hormones*, Immune system*, Interferon-gamma signaling pathway*, CTL mediated immune response ,against target cells*, Interleukin-12-mediated signaling events*, Antiviral mechanism by interferon-stimulated ,genes*, PD-1 signaling*, HIV-induced T cell apoptosis* ,
/opt/mamba/lib/python3.10/site-packages/gseapy/enrichr.py:387: FutureWarning: The frame.append method is deprecated and will be removed from pandas in a future version. Use pandas.concat instead. self.results = self.results.append(res, ignore_index=True)
, ,
Negatively
,
--------------------------------------------------------------------------------------- ,
Ccl5, Ly6c2, Rps12, Rpsa, Cxcr3, Ctla2a, Xcl1, Samd3, Hopx, Rps2, Rplp0, Il2rb, 1700025G04Rik, Gzmm, Cd44, Rplp1, ,Rpl41, Il18rap, Ahnak, Plek, Ifitm10, Ifngr1, Ctla2b, Ccr5, Pglyrp1, Sidt1, Ms4a4c, S100a6, Eomes, Il18r1, Slamf7, ,Lrrk1, Klrd1, H2afz, Gpr183, Bbc3, Zyx, Klre1, Klrc2, Klra7, Npm1, Dennd4a, Il7r, Klrc1, Itm2a, Runx2, Myo1f, ,Itgb1, Ctsw, Ccr2 ,
--------------------------------------------------------------------------------------- ,
Interleukin-2 signaling pathway*, Cytokine-cytokine receptor interaction*, T cell receptor regulation of ,apoptosis*, Binding of chemokines to chemokine receptors*, Cytoplasmic ribosomal proteins*, Ras-independent pathway ,in NK cell-mediated cytotoxicity*, Selective expression of chemokine receptors during T-cell polarization*, ,Interleukin-12-mediated signaling events*, Influenza viral RNA transcription and replication*, Influenza infection* ,
, , , ,
Genes associated with SpatialPC2
,
/opt/mamba/lib/python3.10/site-packages/gseapy/enrichr.py:387: FutureWarning: The frame.append method is deprecated and will be removed from pandas in a future version. Use pandas.concat instead. self.results = self.results.append(res, ignore_index=True)
, ,
Positively
,
--------------------------------------------------------------------------------------- ,
Ly6c2, Ppa1, Isg15, Ms4a4c, Ifit3, Ccl5, Bst2, Hopx, Srm, Xcl1, Ifit1, Plac8, Cxcr3, Hsp90ab1, Nme1, Rsad2, Il2rb, ,Usp18, Eomes, Slamf7, Ifit3b, Pa2g4, Eif5a, Mif, Oas3, Rtp4, Ly6a, Samd3, Lgals3bp, Nolc1, Ranbp1, Isg20, Oasl2, ,C1qbp, Nhp2, Trim30a, Fbl, Ptma, Npm1, Shmt1, Dkc1, Shmt2, Gnl3, Il18rap, Ppp1r14b, Irf7, Igtp, Mettl1, Mybbp1a, ,Nop58 ,
--------------------------------------------------------------------------------------- ,
Interferon alpha/beta signaling*, Interferon signaling*, T cell receptor regulation of apoptosis*, Immune system
,signaling by interferons, interleukins, prolactin, and growth hormones*, Interleukin-2 signaling pathway*, Myc
,active pathway*, Telomere extension by telomerase*, Cyanoamino acid metabolism*, Binding of chemokines to chemokine
,receptors*, Cytokine-cytokine receptor interaction*
,
/opt/mamba/lib/python3.10/site-packages/gseapy/enrichr.py:387: FutureWarning: The frame.append method is deprecated and will be removed from pandas in a future version. Use pandas.concat instead. self.results = self.results.append(res, ignore_index=True)
, ,
Negatively
,
--------------------------------------------------------------------------------------- ,
Malat1, Ccr9, Npc2, Actn1, Rgcc, Tmsb4x, Itgae, Lef1, Cd8b1, Actn2, Cd3g, Ramp1, Saraf, Selplg, Itm2b, Cd3d, Trbc2, ,Cd3e, S100a10, Fam189b, Smc4, Mxd4, Igfbp4, Tdrp, Bcl11b, Thy1, Cd226, Plekho1, Gm14085, H2afv, Gpr68, Themis, ,Ift80, Cd8a, Fth1, Gm42418, Arl5c, Trat1, Cenpa, Gria3, Sox4, Cd247, Lztfl1, Fyb, Trib2, Timp2, Klrd1, Crip1, Tcf7, ,Gm2a ,
--------------------------------------------------------------------------------------- ,
T helper cell surface molecules*, Interleukin-17 signaling pathway*, HIV-induced T cell apoptosis*, ,Immunoregulatory interactions between a lymphoid and a non-lymphoid cell*, Lck and Fyn tyrosine kinases in ,initiation of T cell receptor activation*, CTL mediated immune response against target cells*, Generation of second ,messenger molecules*, NO2-dependent IL-12 pathway in NK cells*, Tob role in T-cell activation*, Inhibition of T ,cell receptor signaling by activated Csk* ,
, , , ,
/opt/mamba/lib/python3.10/site-packages/cmap2d/util.py:32: FutureWarning: `rcond` parameter will change to the default of machine precision times ``max(M, N)`` where M and N are the input matrix dimensions. To use the future default and silence this warning we advise to pass `rcond=None`, to keep using the old, explicitly pass `rcond=-1`. bary_coords = la.lstsq(self._a,b)[0]
Genes associated with SpatialPC1
,
/opt/mamba/lib/python3.10/site-packages/gseapy/enrichr.py:387: FutureWarning: The frame.append method is deprecated and will be removed from pandas in a future version. Use pandas.concat instead. self.results = self.results.append(res, ignore_index=True)
, ,
Positively
,
--------------------------------------------------------------------------------------- ,
Itgax, Csrp1, Dnase1l3, Plxnc1, Anpep, Mif, Ppia, Tuba1c, Tubb5, Clec4n, Mybbp1a, Tuba1a, H2-Eb1, Spint1, Tspan3, ,Procr, Bcl2a1b, Sbf2, Fabp5, Ccnd1, Espl1, Atox1, Gpr68, Vegfa, H2-Aa, Myo1e, H2-Ab1, Tuba1b, Chchd10, Mt1, ,Hnrnpll, Ptma, Epb41l2, Rgl1, Tarm1, Cd63, Traf1, Nr4a3, Nrp2, Relb, Ffar2, Avpi1, Bcl2l1, Rps2, Rogdi, Runx3, ,Rplp1, Hsp90ab1, Odc1, Cks1b ,
--------------------------------------------------------------------------------------- ,
Post-chaperonin tubulin folding pathway*, Fibroblast growth factor 1*, Cooperation of prefoldin and TriC/CCT in ,actin and tubulin folding*, Small cell lung cancer*, Neurophilin interactions with VEGF and VEGF receptor*, ,Pathways in cancer*, Interleukin-2 receptor beta chain in T cell activation*, Protein folding*, Signaling by VEGF*, ,Pathogenic Escherichia coli infection* ,
/opt/mamba/lib/python3.10/site-packages/gseapy/enrichr.py:387: FutureWarning: The frame.append method is deprecated and will be removed from pandas in a future version. Use pandas.concat instead. self.results = self.results.append(res, ignore_index=True)
, ,
Negatively
,
--------------------------------------------------------------------------------------- ,
Ms4a6b, Cybb, Hacd4, Ms4a6c, Hp, Ccl9, Ifi204, Ccl6, Fcgr1, Cst3, Rassf4, Rtp4, Mgst1, Oasl2, Ms4a4c, F13a1, Fn1, ,Ifit3, Ifi47, Wfdc17, Lsp1, Mpeg1, Anxa1, Tiam1, Ifitm3, Msrb1, Trf, Apoe, Slfn1, Ifit2, Ctsb, Fcer1g, Chil3, ,Ly6c2, Ifitm6, Alox5ap, Prr5l, Oas3, Cmpk2, Clec12a, Ifit3b, Sp140, Ms4a4b, Slpi, S100a10, Clec4e, Emilin2, Lpl, ,Cx3cr1, Adgre1 ,
--------------------------------------------------------------------------------------- ,
Interferon alpha/beta signaling*, Interleukin-4 regulation of apoptosis*, TGF-beta regulation of extracellular ,matrix*, Leptin influence on immune response*, T cell receptor regulation of apoptosis, Chylomicron-mediated lipid ,transport, Interferon signaling, Oncostatin M, Alpha-9 beta-1 integrin pathway, Lipoprotein metabolism ,
, , , ,
Genes associated with SpatialPC2
,
/opt/mamba/lib/python3.10/site-packages/gseapy/enrichr.py:387: FutureWarning: The frame.append method is deprecated and will be removed from pandas in a future version. Use pandas.concat instead. self.results = self.results.append(res, ignore_index=True)
, ,
Positively
,
--------------------------------------------------------------------------------------- ,
Itgal, Nr4a1, Cybb, Atf3, Cst3, Ace, Fn1, Hp, Cebpb, Msrb1, Actg1, Ccl6, Lsp1, Flna, Junb, Ahnak, Nxpe4, Nabp1, ,Ear2, Anxa2, Emilin2, Tppp3, F10, Rassf4, Ifitm6, Crip1, Gpx1, F13a1, Lilr4b, Ccl9, Nfam1, Spn, Napsa, Plac8, Ncf2, ,Tnfrsf1b, Mgst1, Trem2, Fcer1g, Sat1, Fgd4, Tyrobp, Tpd52, Adgre4, Nadk, Samsn1, Metrnl, Gpr141, Rab8b, Slpi ,
--------------------------------------------------------------------------------------- ,
T cell receptor regulation of apoptosis*, Alpha-9 beta-1 integrin pathway*, Cell surface interactions at the ,vascular wall*, Hemostasis pathway*, Cross-presentation of particulate exogenous antigens (phagosomes)*, Leukocyte ,transendothelial migration*, Coagulation common pathway, Other semaphorin interactions, Oncostatin M, Interleukin-2 ,signaling pathway ,
/opt/mamba/lib/python3.10/site-packages/gseapy/enrichr.py:387: FutureWarning: The frame.append method is deprecated and will be removed from pandas in a future version. Use pandas.concat instead. self.results = self.results.append(res, ignore_index=True)
, ,
Negatively
,
--------------------------------------------------------------------------------------- ,
H2-Eb1, H2-Ab1, H2-Aa, Dnase1l3, Cd74, Pdlim4, C1qb, C1qa, C1qc, Hebp1, H2-DMb1, Ifi30, Vcam1, Tmem86a, Tmem176a, ,Aif1, Celf4, Cd38, Spint1, Dst, Gbp8, Nr1h3, Ciita, Cd81, P2ry6, Ptms, Basp1, Clec4n, Tmem176b, Kcnj10, Cfb, Pak1, ,Cfp, C1qbp, Spon1, Ctsc, Mafb, Ptma, Ly6a, P2ry12, Gpr68, Gpr162, Pltp, Cox5a, Sdf2l1, Tubb5, Ctsh, Itga9, Asb2, ,Mmp14 ,
--------------------------------------------------------------------------------------- ,
Immune system*, Complement activation, classical pathway*, Complement and coagulation cascades*, Complement ,cascade*, Prion diseases*, Mitochondrial pathway of apoptosis: BH3-only Bcl-2 family*, Adaptive immune system*, MHC ,class II antigen presentation*, Alternative complement pathway*, Beta-1 integrin cell surface interactions ,
, , , ,
B细胞差异表达示例
首先,我们使用 DestVI 插补的 B 细胞特异性基因表达值,显示通过pipeline和Hotspot识别的基因。
其次,我们对生成的计数使用 Kolmogorov-Smirnov 检验,以研究暴露淋巴结中滤泡间区域 (IFA) 和其余区域之间的 B 细胞差异表达。我们在火山图中显示已识别的 IFN 基因,并看到暴露淋巴结的 IFA 区域显著上调。
log2FC | score | pval | |
---|---|---|---|
Irf7 | 3.201532 | 0.949654 | 0.0 |
Rnf213 | 2.159257 | 0.942165 | 0.0 |
Trafd1 | 2.053140 | 0.924055 | 0.0 |
Trim30a | 2.051405 | 0.923377 | 0.0 |
Zbp1 | 2.870445 | 0.913665 | 0.0 |
Rtp4 | 2.954665 | 0.910043 | 0.0 |
Parp14 | 2.178657 | 0.909986 | 0.0 |
Oas3 | 3.802542 | 0.909784 | 0.0 |
Ifit3 | 5.265131 | 0.906739 | 0.0 |
Usp18 | 4.049277 | 0.899177 | 0.0 |

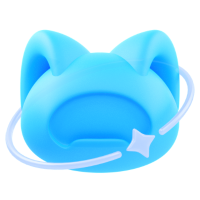
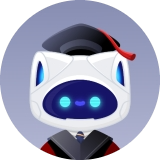
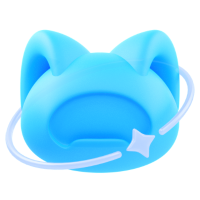