新建
使用PiFold进行蛋白质逆折叠
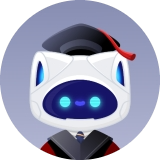
ABOWLofFish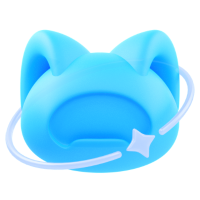
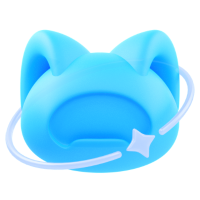
推荐镜像 :Basic Image:ubuntu18.04-py3.10-mamba-cuda10.2
推荐机型 :c12_m92_1 * NVIDIA V100
赞 1
目录
引言
代码
文本
蛋白质逆折叠(Inverse Folding),是指通过给定蛋白质的折叠结构,预测其对应的氨基酸序列。与蛋白质折叠任务相反,蛋白质逆折叠任务是根据已知的蛋白质结构来推断其组成的氨基酸序列。这个任务对于理解蛋白质的结构与功能之间的关系以及设计新的蛋白质具有重要意义。
代码
文本
相关工作
代码
文本
大多数现有工作沿用了端到端的模型架构,使用图神经网络(GNN)来对蛋白质空间结构进行编码,然后使用自回归的方式进行解码
代码
文本
相对之前的工作,新推出的PiFold 模型的一大特点就是:
Effciency & Accuracy Banlancing
PiFold通过多层感知机的方式对蛋白质图的节点和边进行更新,在预测时摒弃了先前自回归的方式直接进行预测,且模型参数量保持在5.5M,大大减少了推理阶段的时间。PiFold相对于先前的SOTA,将三个测试集上的序列恢复率分别提高了4~5%
本文将复现其CATH4.2数据集上的结果
代码
文本
参考colab链接 PiFold Benchmark
github链接 https://github.com/A4Bio/PiFold
代码
文本
1. 环境配置
代码
文本
[1]
! nvidia-smi
Tue Nov 7 05:51:26 2023 +-----------------------------------------------------------------------------+ | NVIDIA-SMI 525.105.17 Driver Version: 525.105.17 CUDA Version: 12.0 | |-------------------------------+----------------------+----------------------+ | GPU Name Persistence-M| Bus-Id Disp.A | Volatile Uncorr. ECC | | Fan Temp Perf Pwr:Usage/Cap| Memory-Usage | GPU-Util Compute M. | | | | MIG M. | |===============================+======================+======================| | 0 Tesla T4 Off | 00000000:00:04.0 Off | 0 | | N/A 68C P8 12W / 70W | 0MiB / 15360MiB | 0% Default | | | | N/A | +-------------------------------+----------------------+----------------------+ +-----------------------------------------------------------------------------+ | Processes: | | GPU GI CI PID Type Process name GPU Memory | | ID ID Usage | |=============================================================================| | No running processes found | +-----------------------------------------------------------------------------+
代码
文本
[2]
# Add this in a Google Colab cell to install the correct version of Pytorch Geometric.
import torch
def format_pytorch_version(version):
return version.split('+')[0]
TORCH_version = torch.__version__
TORCH = format_pytorch_version(TORCH_version)
def format_cuda_version(version):
return 'cu' + version.replace('.', '')
CUDA_version = torch.version.cuda
CUDA = format_cuda_version(CUDA_version)
!pip install torch-scatter -f https://pytorch-geometric.com/whl/torch-{TORCH}+{CUDA}.html
!pip install torch-sparse -f https://pytorch-geometric.com/whl/torch-{TORCH}+{CUDA}.html
!pip install torch-cluster -f https://pytorch-geometric.com/whl/torch-{TORCH}+{CUDA}.html
!pip install torch-spline-conv -f https://pytorch-geometric.com/whl/torch-{TORCH}+{CUDA}.html
!pip install torch-geometric
Looking in links: https://pytorch-geometric.com/whl/torch-2.1.0+cu118.html Collecting torch-scatter Downloading https://data.pyg.org/whl/torch-2.1.0%2Bcu118/torch_scatter-2.1.2%2Bpt21cu118-cp310-cp310-linux_x86_64.whl (10.2 MB) ━━━━━━━━━━━━━━━━━━━━━━━━━━━━━━━━━━━━━━━━ 10.2/10.2 MB 99.6 MB/s eta 0:00:00 Installing collected packages: torch-scatter Successfully installed torch-scatter-2.1.2+pt21cu118 Looking in links: https://pytorch-geometric.com/whl/torch-2.1.0+cu118.html Collecting torch-sparse Downloading https://data.pyg.org/whl/torch-2.1.0%2Bcu118/torch_sparse-0.6.18%2Bpt21cu118-cp310-cp310-linux_x86_64.whl (4.9 MB) ━━━━━━━━━━━━━━━━━━━━━━━━━━━━━━━━━━━━━━━━ 4.9/4.9 MB 33.0 MB/s eta 0:00:00 Requirement already satisfied: scipy in /usr/local/lib/python3.10/dist-packages (from torch-sparse) (1.11.3) Requirement already satisfied: numpy<1.28.0,>=1.21.6 in /usr/local/lib/python3.10/dist-packages (from scipy->torch-sparse) (1.23.5) Installing collected packages: torch-sparse Successfully installed torch-sparse-0.6.18+pt21cu118 Looking in links: https://pytorch-geometric.com/whl/torch-2.1.0+cu118.html Collecting torch-cluster Downloading https://data.pyg.org/whl/torch-2.1.0%2Bcu118/torch_cluster-1.6.3%2Bpt21cu118-cp310-cp310-linux_x86_64.whl (3.3 MB) ━━━━━━━━━━━━━━━━━━━━━━━━━━━━━━━━━━━━━━━━ 3.3/3.3 MB 30.1 MB/s eta 0:00:00 Requirement already satisfied: scipy in /usr/local/lib/python3.10/dist-packages (from torch-cluster) (1.11.3) Requirement already satisfied: numpy<1.28.0,>=1.21.6 in /usr/local/lib/python3.10/dist-packages (from scipy->torch-cluster) (1.23.5) Installing collected packages: torch-cluster Successfully installed torch-cluster-1.6.3+pt21cu118 Looking in links: https://pytorch-geometric.com/whl/torch-2.1.0+cu118.html Collecting torch-spline-conv Downloading https://data.pyg.org/whl/torch-2.1.0%2Bcu118/torch_spline_conv-1.2.2%2Bpt21cu118-cp310-cp310-linux_x86_64.whl (887 kB) ━━━━━━━━━━━━━━━━━━━━━━━━━━━━━━━━━━━━━━━━ 887.8/887.8 kB 16.8 MB/s eta 0:00:00 Installing collected packages: torch-spline-conv Successfully installed torch-spline-conv-1.2.2+pt21cu118 Collecting torch-geometric Downloading torch_geometric-2.4.0-py3-none-any.whl (1.0 MB) ━━━━━━━━━━━━━━━━━━━━━━━━━━━━━━━━━━━━━━━━ 1.0/1.0 MB 10.7 MB/s eta 0:00:00 Requirement already satisfied: tqdm in /usr/local/lib/python3.10/dist-packages (from torch-geometric) (4.66.1) Requirement already satisfied: numpy in /usr/local/lib/python3.10/dist-packages (from torch-geometric) (1.23.5) Requirement already satisfied: scipy in /usr/local/lib/python3.10/dist-packages (from torch-geometric) (1.11.3) Requirement already satisfied: jinja2 in /usr/local/lib/python3.10/dist-packages (from torch-geometric) (3.1.2) Requirement already satisfied: requests in /usr/local/lib/python3.10/dist-packages (from torch-geometric) (2.31.0) Requirement already satisfied: pyparsing in /usr/local/lib/python3.10/dist-packages (from torch-geometric) (3.1.1) Requirement already satisfied: scikit-learn in /usr/local/lib/python3.10/dist-packages (from torch-geometric) (1.2.2) Requirement already satisfied: psutil>=5.8.0 in /usr/local/lib/python3.10/dist-packages (from torch-geometric) (5.9.5) Requirement already satisfied: MarkupSafe>=2.0 in /usr/local/lib/python3.10/dist-packages (from jinja2->torch-geometric) (2.1.3) Requirement already satisfied: charset-normalizer<4,>=2 in /usr/local/lib/python3.10/dist-packages (from requests->torch-geometric) (3.3.1) Requirement already satisfied: idna<4,>=2.5 in /usr/local/lib/python3.10/dist-packages (from requests->torch-geometric) (3.4) Requirement already satisfied: urllib3<3,>=1.21.1 in /usr/local/lib/python3.10/dist-packages (from requests->torch-geometric) (2.0.7) Requirement already satisfied: certifi>=2017.4.17 in /usr/local/lib/python3.10/dist-packages (from requests->torch-geometric) (2023.7.22) Requirement already satisfied: joblib>=1.1.1 in /usr/local/lib/python3.10/dist-packages (from scikit-learn->torch-geometric) (1.3.2) Requirement already satisfied: threadpoolctl>=2.0.0 in /usr/local/lib/python3.10/dist-packages (from scikit-learn->torch-geometric) (3.2.0) Installing collected packages: torch-geometric Successfully installed torch-geometric-2.4.0
代码
文本
[3]
import json, time, os, sys, glob
import numpy as np
if not os.path.isdir("ProDesign"):
os.system("git clone -q https://github.com/A4Bio/ProDesign.git")
sys.path.append('/content/ProDesign')
代码
文本
[4]
!mkdir -p ProDesign/data/cath
!mkdir -p ProDesign/data/ts
!wget -O ProDesign/data/cath.zip https://github.com/A4Bio/PiFold/releases/download/Training%26Data/cath4.2.zip
!unzip ProDesign/data/cath.zip -d ProDesign/data/cath
!mv ProDesign/data/cath/cath4.2/* ProDesign/data/cath/
!wget -O ProDesign/data/ts.zip https://github.com/A4Bio/PiFold/releases/download/Training%26Data/ts.zip
!unzip ProDesign/data/ts.zip -d ProDesign/data/
!mkdir -p ProDesign/results/ProDesign
!wget -O ProDesign/results/ProDesign/checkpoint.pth https://github.com/A4Bio/PiFold/releases/download/Training%26Data/checkpoint.pth
--2023-11-07 05:52:01-- https://github.com/A4Bio/PiFold/releases/download/Training%26Data/cath4.2.zip Resolving github.com (github.com)... 140.82.121.3 Connecting to github.com (github.com)|140.82.121.3|:443... connected. HTTP request sent, awaiting response... 302 Found Location: https://objects.githubusercontent.com/github-production-release-asset-2e65be/538405150/36fd2f8c-5e3b-4a07-9741-dc881be4a4e5?X-Amz-Algorithm=AWS4-HMAC-SHA256&X-Amz-Credential=AKIAIWNJYAX4CSVEH53A%2F20231107%2Fus-east-1%2Fs3%2Faws4_request&X-Amz-Date=20231107T055201Z&X-Amz-Expires=300&X-Amz-Signature=5f1938c826837e08ac56930a5c0b4e582912694e95f6a786bee0a557732af9cd&X-Amz-SignedHeaders=host&actor_id=0&key_id=0&repo_id=538405150&response-content-disposition=attachment%3B%20filename%3Dcath4.2.zip&response-content-type=application%2Foctet-stream [following] --2023-11-07 05:52:01-- https://objects.githubusercontent.com/github-production-release-asset-2e65be/538405150/36fd2f8c-5e3b-4a07-9741-dc881be4a4e5?X-Amz-Algorithm=AWS4-HMAC-SHA256&X-Amz-Credential=AKIAIWNJYAX4CSVEH53A%2F20231107%2Fus-east-1%2Fs3%2Faws4_request&X-Amz-Date=20231107T055201Z&X-Amz-Expires=300&X-Amz-Signature=5f1938c826837e08ac56930a5c0b4e582912694e95f6a786bee0a557732af9cd&X-Amz-SignedHeaders=host&actor_id=0&key_id=0&repo_id=538405150&response-content-disposition=attachment%3B%20filename%3Dcath4.2.zip&response-content-type=application%2Foctet-stream Resolving objects.githubusercontent.com (objects.githubusercontent.com)... 185.199.108.133, 185.199.110.133, 185.199.109.133, ... Connecting to objects.githubusercontent.com (objects.githubusercontent.com)|185.199.108.133|:443... connected. HTTP request sent, awaiting response... 200 OK Length: 188153738 (179M) [application/octet-stream] Saving to: ‘ProDesign/data/cath.zip’ ProDesign/data/cath 100%[===================>] 179.44M 48.4MB/s in 4.0s 2023-11-07 05:52:05 (44.7 MB/s) - ‘ProDesign/data/cath.zip’ saved [188153738/188153738] Archive: ProDesign/data/cath.zip inflating: ProDesign/data/cath/cath4.2/chain_set.jsonl inflating: ProDesign/data/cath/cath4.2/chain_set_splits.json inflating: ProDesign/data/cath/cath4.2/download_cath.sh inflating: ProDesign/data/cath/cath4.2/ollikainen_set.jsonl inflating: ProDesign/data/cath/cath4.2/remove.json inflating: ProDesign/data/cath/cath4.2/test_split_L100.json inflating: ProDesign/data/cath/cath4.2/test_split_sc.json --2023-11-07 05:52:13-- https://github.com/A4Bio/PiFold/releases/download/Training%26Data/ts.zip Resolving github.com (github.com)... 140.82.121.3 Connecting to github.com (github.com)|140.82.121.3|:443... connected. HTTP request sent, awaiting response... 302 Found Location: https://objects.githubusercontent.com/github-production-release-asset-2e65be/538405150/1c1dd67b-49ba-4d5f-a8de-9b4d48ae7fee?X-Amz-Algorithm=AWS4-HMAC-SHA256&X-Amz-Credential=AKIAIWNJYAX4CSVEH53A%2F20231107%2Fus-east-1%2Fs3%2Faws4_request&X-Amz-Date=20231107T055213Z&X-Amz-Expires=300&X-Amz-Signature=10794e82452afec5455b63dd111e6d898f2cb5fc68c51eefdb831cbb8a3b84d4&X-Amz-SignedHeaders=host&actor_id=0&key_id=0&repo_id=538405150&response-content-disposition=attachment%3B%20filename%3Dts.zip&response-content-type=application%2Foctet-stream [following] --2023-11-07 05:52:13-- https://objects.githubusercontent.com/github-production-release-asset-2e65be/538405150/1c1dd67b-49ba-4d5f-a8de-9b4d48ae7fee?X-Amz-Algorithm=AWS4-HMAC-SHA256&X-Amz-Credential=AKIAIWNJYAX4CSVEH53A%2F20231107%2Fus-east-1%2Fs3%2Faws4_request&X-Amz-Date=20231107T055213Z&X-Amz-Expires=300&X-Amz-Signature=10794e82452afec5455b63dd111e6d898f2cb5fc68c51eefdb831cbb8a3b84d4&X-Amz-SignedHeaders=host&actor_id=0&key_id=0&repo_id=538405150&response-content-disposition=attachment%3B%20filename%3Dts.zip&response-content-type=application%2Foctet-stream Resolving objects.githubusercontent.com (objects.githubusercontent.com)... 185.199.108.133, 185.199.109.133, 185.199.110.133, ... Connecting to objects.githubusercontent.com (objects.githubusercontent.com)|185.199.108.133|:443... connected. HTTP request sent, awaiting response... 200 OK Length: 5451231 (5.2M) [application/octet-stream] Saving to: ‘ProDesign/data/ts.zip’ ProDesign/data/ts.z 100%[===================>] 5.20M --.-KB/s in 0.1s 2023-11-07 05:52:13 (52.7 MB/s) - ‘ProDesign/data/ts.zip’ saved [5451231/5451231] Archive: ProDesign/data/ts.zip inflating: ProDesign/data/ts/ts50.json inflating: ProDesign/data/ts/ts500.json --2023-11-07 05:52:14-- https://github.com/A4Bio/PiFold/releases/download/Training%26Data/checkpoint.pth Resolving github.com (github.com)... 140.82.121.3 Connecting to github.com (github.com)|140.82.121.3|:443... connected. HTTP request sent, awaiting response... 302 Found Location: https://objects.githubusercontent.com/github-production-release-asset-2e65be/538405150/9a5583fd-598e-4249-b69a-0684ce1d796a?X-Amz-Algorithm=AWS4-HMAC-SHA256&X-Amz-Credential=AKIAIWNJYAX4CSVEH53A%2F20231107%2Fus-east-1%2Fs3%2Faws4_request&X-Amz-Date=20231107T055214Z&X-Amz-Expires=300&X-Amz-Signature=a0ff47ef131b8dbd0ec5a32144a20e01cb61e7b6c206fdac5c38347792f3a860&X-Amz-SignedHeaders=host&actor_id=0&key_id=0&repo_id=538405150&response-content-disposition=attachment%3B%20filename%3Dcheckpoint.pth&response-content-type=application%2Foctet-stream [following] --2023-11-07 05:52:14-- https://objects.githubusercontent.com/github-production-release-asset-2e65be/538405150/9a5583fd-598e-4249-b69a-0684ce1d796a?X-Amz-Algorithm=AWS4-HMAC-SHA256&X-Amz-Credential=AKIAIWNJYAX4CSVEH53A%2F20231107%2Fus-east-1%2Fs3%2Faws4_request&X-Amz-Date=20231107T055214Z&X-Amz-Expires=300&X-Amz-Signature=a0ff47ef131b8dbd0ec5a32144a20e01cb61e7b6c206fdac5c38347792f3a860&X-Amz-SignedHeaders=host&actor_id=0&key_id=0&repo_id=538405150&response-content-disposition=attachment%3B%20filename%3Dcheckpoint.pth&response-content-type=application%2Foctet-stream Resolving objects.githubusercontent.com (objects.githubusercontent.com)... 185.199.108.133, 185.199.109.133, 185.199.110.133, ... Connecting to objects.githubusercontent.com (objects.githubusercontent.com)|185.199.108.133|:443... connected. HTTP request sent, awaiting response... 200 OK Length: 26768195 (26M) [application/octet-stream] Saving to: ‘ProDesign/results/ProDesign/checkpoint.pth’ ProDesign/results/P 100%[===================>] 25.53M 48.0MB/s in 0.5s 2023-11-07 05:52:15 (48.0 MB/s) - ‘ProDesign/results/ProDesign/checkpoint.pth’ saved [26768195/26768195]
代码
文本
2. 加载模型
代码
文本
[5]
def get_parser():
import argparse
parser = argparse.ArgumentParser()
# Set-up parameters
parser.add_argument('--device', default='cuda', type=str, help='Name of device to use for tensor computations (cuda/cpu)')
parser.add_argument('--display_step', default=10, type=int, help='Interval in batches between display of training metrics')
parser.add_argument('--res_dir', default='ProDesign/results', type=str)
parser.add_argument('--ex_name', default='ProDesign', type=str)
parser.add_argument('--use_gpu', default=True, type=bool)
parser.add_argument('--gpu', default=0, type=int)
parser.add_argument('--seed', default=111, type=int)
# CATH
# dataset parameters
parser.add_argument('--data_name', default='CATH', choices=['CATH', 'TS50'])
parser.add_argument('--data_root', default='ProDesign/data/')
parser.add_argument('--batch_size', default=8, type=int)
parser.add_argument('--num_workers', default=8, type=int)
# method parameters
parser.add_argument('--method', default='ProDesign', choices=['ProDesign'])
parser.add_argument('--config_file', '-c', default=None, type=str)
parser.add_argument('--hidden_dim', default=128, type=int)
parser.add_argument('--node_features', default=128, type=int)
parser.add_argument('--edge_features', default=128, type=int)
parser.add_argument('--k_neighbors', default=30, type=int)
parser.add_argument('--dropout', default=0.1, type=int)
parser.add_argument('--num_encoder_layers', default=10, type=int)
# Training parameters
parser.add_argument('--epoch', default=100, type=int, help='end epoch')
parser.add_argument('--log_step', default=1, type=int)
parser.add_argument('--lr', default=0.001, type=float, help='Learning rate')
parser.add_argument('--patience', default=100, type=int)
# ProDesign parameters
parser.add_argument('--updating_edges', default=4, type=int)
parser.add_argument('--node_dist', default=1, type=int)
parser.add_argument('--node_angle', default=1, type=int)
parser.add_argument('--node_direct', default=1, type=int)
parser.add_argument('--edge_dist', default=1, type=int)
parser.add_argument('--edge_angle', default=1, type=int)
parser.add_argument('--edge_direct', default=1, type=int)
parser.add_argument('--virtual_num', default=3, type=int)
args = parser.parse_args([])
return args
import torch
from ProDesign.main import Exp
from ProDesign.parser import create_parser
args = get_parser()
exp = Exp(args)
svpath = 'ProDesign/results/ProDesign/'
exp.method.model.load_state_dict(torch.load(svpath+'checkpoint.pth'))
Use GPU: cuda:0 100%|██████████| 21668/21668 [00:33<00:00, 655.05it/s] device: cuda display_step: 10 res_dir: ProDesign/results ex_name: ProDesign use_gpu: True gpu: 0 seed: 111 data_name: CATH data_root: ProDesign/data/ batch_size: 8 num_workers: 8 method: ProDesign config_file: None hidden_dim: 128 node_features: 128 edge_features: 128 k_neighbors: 30 dropout: 0.1 num_encoder_layers: 10 epoch: 100 log_step: 1 lr: 0.001 patience: 100 updating_edges: 4 node_dist: 1 node_angle: 1 node_direct: 1 edge_dist: 1 edge_angle: 1 edge_direct: 1 virtual_num: 3
<All keys matched successfully>
代码
文本
3. CATH4.2 结果
代码
文本
[6]
from API.dataloader import make_cath_loader
from API.cath_dataset import CATH
with open('ProDesign/data/cath/chain_set_splits.json','r') as f:
test_split = json.load(f)
with open('ProDesign/data/cath/test_split_L100.json','r') as f:
test_short_split = json.load(f)
with open('ProDesign/data/cath/test_split_sc.json','r') as f:
test_SC_split = json.load(f)
alphabet='ACDEFGHIKLMNPQRSTVWY'
alphabet_set = set([a for a in alphabet])
max_length = 500
with open('ProDesign/data/cath/chain_set.jsonl') as f:
lines = f.readlines()
data_list = []
for line in lines:
entry = json.loads(line)
seq = entry['seq']
for key, val in entry['coords'].items():
entry['coords'][key] = np.asarray(val)
bad_chars = set([s for s in seq]).difference(alphabet_set)
if len(bad_chars) == 0:
if len(entry['seq']) <= max_length:
data_list.append({
'title':entry['name'],
'seq':entry['seq'],
'CA':entry['coords']['CA'],
'C':entry['coords']['C'],
'O':entry['coords']['O'],
'N':entry['coords']['N']
})
test_full_list = []
test_SC_list = []
test_Short_list = []
for data in data_list:
if data['title'] in test_split['test']:
test_full_list.append(data)
if data['title'] in test_SC_split['test']:
test_SC_list.append(data)
if data['title'] in test_short_split['test']:
test_Short_list.append(data)
print(">Loading",len(test_full_list),"samples for testAll")
print(">Loading",len(test_SC_list),"samples for testSC")
print(">Loading",len(test_Short_list),"samples for testShort")
exp.test_loader = make_cath_loader(CATH(data=test_full_list), 'SimDesign', 8)
exp.test()
print("median: {:.4f}\t mean: {:.4f}\t std: {:.4f}\t min: {:.4f}\t max: {:.4f}".format(exp.method.median_recovery, exp.method.mean_recovery, exp.method.std_recovery, exp.method.min_recovery, exp.method.max_recovery))
exp.test_loader = make_cath_loader(CATH(data=test_SC_list), 'SimDesign', 8)
exp.test()
print("median: {:.4f}\t mean: {:.4f}\t std: {:.4f}\t min: {:.4f}\t max: {:.4f}".format(exp.method.median_recovery, exp.method.mean_recovery, exp.method.std_recovery, exp.method.min_recovery, exp.method.max_recovery))
exp.test_loader = make_cath_loader(CATH(data=test_Short_list), 'SimDesign', 8)
exp.test()
print("median: {:.4f}\t mean: {:.4f}\t std: {:.4f}\t min: {:.4f}\t max: {:.4f}".format(exp.method.median_recovery, exp.method.mean_recovery, exp.method.std_recovery, exp.method.min_recovery, exp.method.max_recovery))
>Loading 1120 samples for testAll >Loading 103 samples for testSC >Loading 94 samples for testShort test loss: 1.3224: 100%|██████████| 140/140 [00:23<00:00, 6.07it/s] 100%|██████████| 1120/1120 [00:58<00:00, 19.13it/s] Test Perp: 4.5533, Test Rec: 0.5166 Category Unknown Rec: 0.5166 median: 0.5166 mean: 0.4939 std: 0.1113 min: 0.0896 max: 0.7698 test loss: 1.5457: 100%|██████████| 13/13 [00:01<00:00, 7.08it/s] 100%|██████████| 103/103 [00:05<00:00, 17.75it/s] Test Perp: 6.2962, Test Rec: 0.3846 Category Unknown Rec: 0.3846 median: 0.3846 mean: 0.4050 std: 0.1338 min: 0.1522 max: 0.7556 test loss: 1.7012: 100%|██████████| 12/12 [00:01<00:00, 7.99it/s] 100%|██████████| 94/94 [00:04<00:00, 21.64it/s]Test Perp: 6.0340, Test Rec: 0.3984 Category Unknown Rec: 0.3984 median: 0.3984 mean: 0.4134 std: 0.1407 min: 0.1167 max: 0.7556
代码
文本
4.如果你想从头训练PiFold
代码
文本
pifold仓库提供了训练脚本,可以直接运行main.py
使用parser中定义的默认参数即可
代码
文本
[ ]
%cd ProDesign
!python main.py
/content/ProDesign {'device': 'cuda', 'display_step': 10, 'res_dir': './results', 'ex_name': 'debug', 'use_gpu': True, 'gpu': 0, 'seed': 111, 'data_name': 'CATH', 'data_root': './data/', 'batch_size': 8, 'num_workers': 8, 'method': 'ProDesign', 'config_file': None, 'hidden_dim': 128, 'node_features': 128, 'edge_features': 128, 'k_neighbors': 30, 'dropout': 0.1, 'num_encoder_layers': 10, 'epoch': 100, 'log_step': 1, 'lr': 0.001, 'patience': 100, 'updating_edges': 4, 'node_dist': 1, 'node_angle': 1, 'node_direct': 1, 'edge_dist': 1, 'edge_angle': 1, 'edge_direct': 1, 'virtual_num': 3} Use GPU: cuda:0 100% 21668/21668 [00:21<00:00, 1015.98it/s] device: cuda display_step: 10 res_dir: ./results ex_name: debug use_gpu: True gpu: 0 seed: 111 data_name: CATH data_root: ./data/ batch_size: 8 num_workers: 8 method: ProDesign config_file: None hidden_dim: 128 node_features: 128 edge_features: 128 k_neighbors: 30 dropout: 0.1 num_encoder_layers: 10 epoch: 100 log_step: 1 lr: 0.001 patience: 100 updating_edges: 4 node_dist: 1 node_angle: 1 node_direct: 1 edge_dist: 1 edge_angle: 1 edge_direct: 1 virtual_num: 3 >>>>>>>>>>>>>>>>>>>>>>>>>> training <<<<<<<<<<<<<<<<<<<<<<<<<<<<<<<<< train loss: 2.6155: 6% 135/2253 [00:59<14:09, 2.49it/s]
代码
文本
已赞1
推荐阅读
公开
Reproduction of ESM-IF1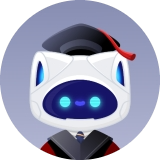
ABOWLofFish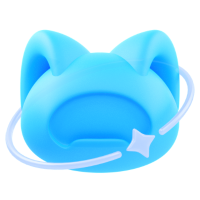
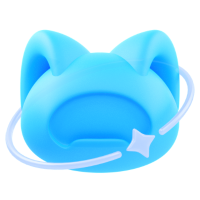
发布于 2023-09-20
1 转存文件
公开
PTM Prediction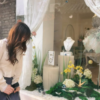
mengyue@dp.tech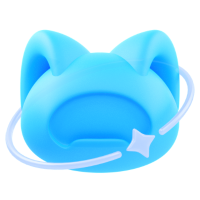
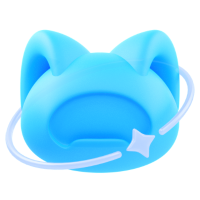
发布于 2023-10-12
2 转存文件
评论
Zhifeng Gao